商务统计学课件英文版BSFC7eCH02
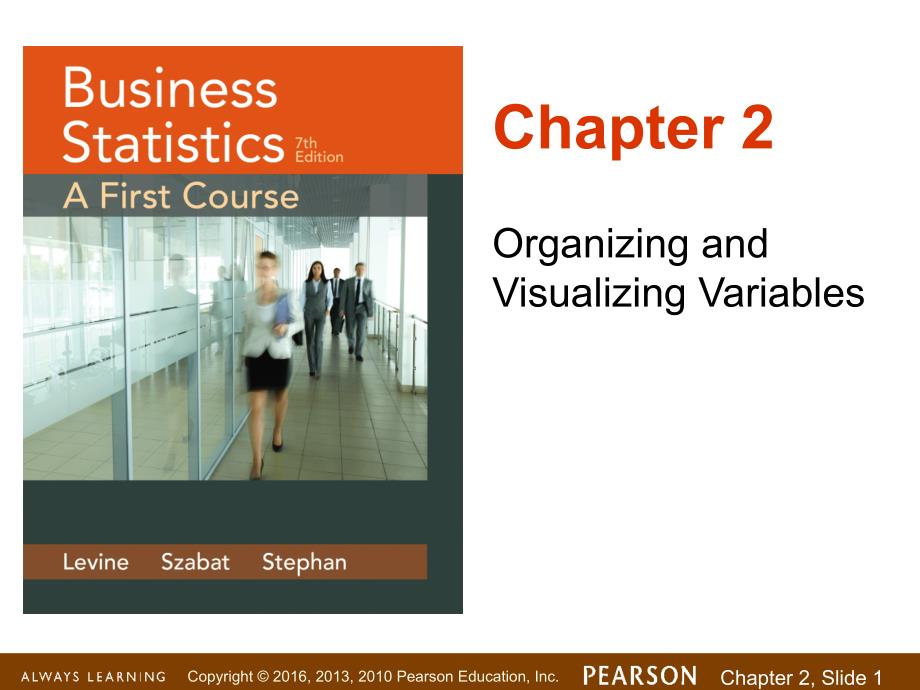


《商务统计学课件英文版BSFC7eCH02》由会员分享,可在线阅读,更多相关《商务统计学课件英文版BSFC7eCH02(61页珍藏版)》请在装配图网上搜索。
1、 Copyright 2016,2013,2010 Pearson Education,Inc.Chapter 2,Slide 1Organizing and Visualizing VariablesChapter 2 Copyright 2016,2013,2010 Pearson Education,Inc.Chapter 2,Slide 2ObjectivesIn this chapter you learn:nMethods to organize variables.nMethods to visualize variables.nMethods to organize or vi
2、sualize more than one variable at the same time.nPrinciples of proper visualizations.Copyright 2016,2013,2010 Pearson Education,Inc.Chapter 2,Slide 3Categorical Data Are Organized By Utilizing TablesDCOVACategorical DataTallying Data Summary Table One Categorical Variable Two Categorical VariablesCo
3、ntingency Table Copyright 2016,2013,2010 Pearson Education,Inc.Chapter 2,Slide 4Organizing Categorical Data:Summary TableA summary table tallies the frequencies or percentages of items in a set of categories so that you can see differences between categories.Reason For Shopping Online?PercentBetter
4、Prices37%Avoiding holiday crowds or hassles29%Convenience18%Better selection13%Ships directly 3%DCOVAMain Reason Young Adults Shop OnlineSource:Data extracted and adapted from“Main Reason Young Adults Shop Online?”USA Today,December 5,2012,p.1A.Copyright 2016,2013,2010 Pearson Education,Inc.Chapter
5、2,Slide 5A Contingency Table Helps Organize Two or More Categorical VariablesnUsed to study patterns that may exist between the responses of two or more categorical variablesnCross tabulates or tallies jointly the responses of the categorical variablesnFor two variables the tallies for one variable
6、are located in the rows and the tallies for the second variable are located in the columnsDCOVA Copyright 2016,2013,2010 Pearson Education,Inc.Chapter 2,Slide 6Contingency Table-ExamplenA random sample of 400 invoices is drawn.nEach invoice is categorized as a small,medium,or large amount.nEach invo
7、ice is also examined to identify if there are any errors.nThis data are then organized in the contingency table to the right.DCOVANoErrorsErrorsTotalSmallAmount17020190MediumAmount10040140LargeAmount65570Total33565400Contingency Table ShowingFrequency of Invoices CategorizedBy Size and The Presence
8、Of Errors Copyright 2016,2013,2010 Pearson Education,Inc.Chapter 2,Slide 7Contingency Table Based On Percentage Of Overall TotalNoErrorsErrorsTotalSmallAmount17020190MediumAmount10040140LargeAmount65570Total33565400DCOVANoErrorsErrorsTotalSmallAmount42.50%5.00%47.50%MediumAmount25.00%10.00%35.00%Lar
9、geAmount16.25%1.25%17.50%Total83.75%16.25%100.0%42.50%=170/40025.00%=100/40016.25%=65/40083.75%of sampled invoices have no errors and 47.50%of sampled invoices are for small amounts.Copyright 2016,2013,2010 Pearson Education,Inc.Chapter 2,Slide 8Contingency Table Based On Percentage of Row TotalsNoE
10、rrorsErrorsTotalSmallAmount17020190MediumAmount10040140LargeAmount65570Total33565400DCOVANoErrorsErrorsTotalSmallAmount89.47%10.53%100.0%MediumAmount71.43%28.57%100.0%LargeAmount92.86%7.14%100.0%Total83.75%16.25%100.0%89.47%=170/19071.43%=100/14092.86%=65/70Medium invoices have a larger chance(28.57
11、%)of having errors than small(10.53%)or large(7.14%)invoices.Copyright 2016,2013,2010 Pearson Education,Inc.Chapter 2,Slide 9Contingency Table Based On Percentage Of Column TotalsNoErrorsErrorsTotalSmallAmount17020190MediumAmount10040140LargeAmount65570Total33565400DCOVANoErrorsErrorsTotalSmallAmoun
12、t50.75%30.77%47.50%MediumAmount29.85%61.54%35.00%LargeAmount19.40%7.69%17.50%Total100.0%100.0%100.0%50.75%=170/33530.77%=20/65There is a 61.54%chance that invoices with errors are of medium size.Copyright 2016,2013,2010 Pearson Education,Inc.Chapter 2,Slide 10Tables Used For Organizing Numerical Dat
13、aDCOVANumerical DataOrdered ArrayCumulativeDistributionsFrequencyDistributions Copyright 2016,2013,2010 Pearson Education,Inc.Chapter 2,Slide 11Organizing Numerical Data:Ordered ArrayAn ordered array is a sequence of data,in rank order,from the smallest value to the largest value.Shows range(minimum
14、 value to maximum value)May help identify outliers(unusual observations)Age of Surveyed College StudentsDay Students161717181818191920202122222527323842Night Students181819192021232832334145DCOVA Copyright 2016,2013,2010 Pearson Education,Inc.Chapter 2,Slide 12Organizing Numerical Data:Frequency Dis
15、tributionThe frequency distribution is a summary table in which the data are arranged into numerically ordered classes.You must give attention to selecting the appropriate number of class groupings for the table,determining a suitable width of a class grouping,and establishing the boundaries of each
16、 class grouping to avoid overlapping.The number of classes depends on the number of values in the data.With a larger number of values,typically there are more classes.In general,a frequency distribution should have at least 5 but no more than 15 classes.To determine the width of a class interval,you
17、 divide the range(Highest valueLowest value)of the data by the number of class groupings desired.DCOVA Copyright 2016,2013,2010 Pearson Education,Inc.Chapter 2,Slide 13Organizing Numerical Data:Frequency Distribution ExampleExample:A manufacturer of insulation randomly selects 20 winter days and rec
18、ords the daily high temperature24,35,17,21,24,37,26,46,58,30,32,13,12,38,41,43,44,27,53,27DCOVA Copyright 2016,2013,2010 Pearson Education,Inc.Chapter 2,Slide 14Organizing Numerical Data:Frequency Distribution ExampleSort raw data in ascending order:12,13,17,21,24,24,26,27,27,30,32,35,37,38,41,43,44
19、,46,53,58Find range:58-12=46Select number of classes:5(usually between 5 and 15)Compute class interval(width):10(46/5 then round up)Determine class boundaries(limits):Class 1:10 but less than 20Class 2:20 but less than 30Class 3:30 but less than 40Class 4:40 but less than 50Class 5:50 but less than
20、60Compute class midpoints:15,25,35,45,55Count observations&assign to classesDCOVA Copyright 2016,2013,2010 Pearson Education,Inc.Chapter 2,Slide 15Organizing Numerical Data:Frequency Distribution Example Class MidpointsFrequency10 but less than 20 15320 but less than 30 25630 but less than 40 355 40
21、 but less than 50 45450 but less than 60 552 Total 20Data in ordered array:12,13,17,21,24,24,26,27,27,30,32,35,37,38,41,43,44,46,53,58DCOVA Copyright 2016,2013,2010 Pearson Education,Inc.Chapter 2,Slide 16Organizing Numerical Data:Relative&Percent Frequency Distribution Example Class Frequency10 but
22、 less than 20 3 .15 15%20 but less than 30 6 .30 30%30 but less than 40 5 .25 25%40 but less than 50 4 .20 20%50 but less than 60 2 .10 10%Total 20 1.00 100%RelativeFrequency PercentageDCOVARelative Frequency=Frequency/Total,e.g.0.10=2/20 Copyright 2016,2013,2010 Pearson Education,Inc.Chapter 2,Slid
23、e 17Organizing Numerical Data:Cumulative Frequency Distribution ExampleClass10 but less than 20 3 15%3 15%20 but less than 30 6 30%9 45%30 but less than 40 5 25%14 70%40 but less than 50 4 20%18 90%50 but less than 60 2 10%20 100%Total 20 100 20100%PercentageCumulative PercentageCumulative Percentag
24、e=Cumulative Frequency/Total*100 e.g.45%=100*9/20FrequencyCumulative FrequencyDCOVA Copyright 2016,2013,2010 Pearson Education,Inc.Chapter 2,Slide 18Why Use a Frequency Distribution?nIt condenses the raw data into a more useful formnIt allows for a quick visual interpretation of the datanIt enables
25、the determination of the major characteristics of the data set including where the data are concentrated/clusteredDCOVA Copyright 2016,2013,2010 Pearson Education,Inc.Chapter 2,Slide 19Frequency Distributions:Some TipsnDifferent class boundaries may provide different pictures for the same data(espec
26、ially for smaller data sets)nShifts in data concentration may show up when different class boundaries are chosennAs the size of the data set increases,the impact of alterations in the selection of class boundaries is greatly reducednWhen comparing two or more groups with different sample sizes,you m
27、ust use either a relative frequency or a percentage distributionDCOVA Copyright 2016,2013,2010 Pearson Education,Inc.Chapter 2,Slide 20Visualizing Categorical Data Through Graphical DisplaysDCOVACategorical DataVisualizing Data BarChartSummary Table For One VariableContingency Table For Two Variable
28、sSide By Side Bar Chart Pie Chart ParetoChart Copyright 2016,2013,2010 Pearson Education,Inc.Chapter 2,Slide 21Visualizing Categorical Data:The Bar ChartThe bar chart visualizes a categorical variable as a series of bars.The length of each bar represents either the frequency or percentage of values
29、for each category.Each bar is separated by a space called a gap.DCOVAReason For Shopping Online?PercentBetter Prices37%Avoiding holiday crowds or hassles29%Convenience18%Better selection13%Ships directly 3%Copyright 2016,2013,2010 Pearson Education,Inc.Chapter 2,Slide 22Visualizing Categorical Data:
30、The Pie ChartThe pie chart is a circle broken up into slices that represent categories.The size of each slice of the pie varies according to the percentage in each category.DCOVAReason For Shopping Online?PercentBetter Prices37%Avoiding holiday crowds or hassles29%Convenience18%Better selection13%Sh
31、ips directly 3%Copyright 2016,2013,2010 Pearson Education,Inc.Chapter 2,Slide 23Visualizing Categorical Data:The Pareto ChartnUsed to portray categorical datanA vertical bar chart,where categories are shown in descending order of frequencynA cumulative polygon is shown in the same graphnUsed to sepa
32、rate the“vital few”from the“trivial many”DCOVA Copyright 2016,2013,2010 Pearson Education,Inc.Chapter 2,Slide 24Visualizing Categorical Data:The Pareto Chart(cont)DCOVA CumulativeCause Frequency PercentPercentWarped card jammed 365 50.41%50.41%Card unreadable 234 32.32%82.73%ATM malfunctions32 4.42%
33、87.15%ATM out of cash28 3.87%91.02%Invalid amount requested 23 3.18%94.20%Wrong keystroke 23 3.18%97.38%Lack of funds in account 19 2.62%100.00%Total 724 100.00%Source:Data extracted from A.Bhalla,“Dont Misuse the Pareto Principle,”Six Sigma ForumMagazine,May 2009,pp.1518.Ordered Summary Table For C
34、ausesOf Incomplete ATM Transactions Copyright 2016,2013,2010 Pearson Education,Inc.Chapter 2,Slide 25Visualizing Categorical Data:The Pareto Chart(cont)DCOVAThe“VitalFew”Copyright 2016,2013,2010 Pearson Education,Inc.Chapter 2,Slide 26Visualizing Categorical Data:Side By Side Bar ChartsThe side by s
35、ide bar chart represents the data from a contingency table.DCOVA0.0%10.0%20.0%30.0%40.0%50.0%60.0%70.0%No ErrorsErrorsInvoice Size Split Out By Errors&No ErrorsLargeMediumSmallInvoices with errors are much more likely to be ofmedium size(61.54%vs 30.77%and 7.69%)NoErrorsErrorsTotalSmallAmount50.75%3
36、0.77%47.50%MediumAmount29.85%61.54%35.00%LargeAmount19.40%7.69%17.50%Total100.0%100.0%100.0%Copyright 2016,2013,2010 Pearson Education,Inc.Chapter 2,Slide 27Visualizing Numerical Data By Using Graphical DisplaysNumerical DataOrdered ArrayStem-and-LeafDisplayHistogramPolygonOgiveFrequency Distributio
37、ns andCumulative DistributionsDCOVA Copyright 2016,2013,2010 Pearson Education,Inc.Chapter 2,Slide 28Stem-and-Leaf DisplaynA simple way to see how the data are distributed and where concentrations of data existMETHOD:Separate the sorted data series into leading digits(the stems)and the trailing digi
38、ts(the leaves)DCOVA Copyright 2016,2013,2010 Pearson Education,Inc.Chapter 2,Slide 29Organizing Numerical Data:Stem and Leaf DisplayA stem-and-leaf display organizes data into groups(called stems)so that the values within each group(the leaves)branch out to the right on each row.StemLeaf167788899200
39、1225732842 Age of College Students Day Students Night StudentsStemLeaf1889920138323415Age of Surveyed College StudentsDay Students161717181818191920202122222527323842Night Students181819192021232832334145DCOVA Copyright 2016,2013,2010 Pearson Education,Inc.Chapter 2,Slide 30Visualizing Numerical Dat
40、a:The HistogramA vertical bar chart of the data in a frequency distribution is called a histogram.In a histogram there are no gaps between adjacent bars.The class boundaries(or class midpoints)are shown on the horizontal axis.The vertical axis is either frequency,relative frequency,or percentage.The
41、 height of the bars represent the frequency,relative frequency,or percentage.DCOVA Copyright 2016,2013,2010 Pearson Education,Inc.Chapter 2,Slide 31Visualizing Numerical Data:The Histogram Class Frequency10 but less than 20 3 .15 1520 but less than 30 6 .30 3030 but less than 40 5 .25 25 40 but less
42、 than 50 4 .20 2050 but less than 60 2 .10 10 Total 20 1.00 100RelativeFrequency Percentage(In a percentage histogram the vertical axis would be defined to show the percentage of observations per class)DCOVA Copyright 2016,2013,2010 Pearson Education,Inc.Chapter 2,Slide 32Visualizing Numerical Data:
43、The PolygonA percentage polygon is formed by having the midpoint of each class represent the data in that class and then connecting the sequence of midpoints at their respective class percentages.The cumulative percentage polygon,or ogive,displays the variable of interest along the X axis,and the cu
44、mulative percentages along the Y axis.Useful when there are two or more groups to compare.DCOVA Copyright 2016,2013,2010 Pearson Education,Inc.Chapter 2,Slide 33Visualizing Numerical Data:The Percentage PolygonDCOVAUseful When Comparing Two or More Groups Copyright 2016,2013,2010 Pearson Education,I
45、nc.Chapter 2,Slide 34Visualizing Numerical Data:The Percentage PolygonDCOVA Copyright 2016,2013,2010 Pearson Education,Inc.Chapter 2,Slide 35Visualizing Two Numerical Variables By Using Graphical DisplaysTwo Numerical VariablesScatter PlotTime-Series PlotDCOVA Copyright 2016,2013,2010 Pearson Educat
46、ion,Inc.Chapter 2,Slide 36Visualizing Two Numerical Variables:The Scatter PlotScatter plots are used for numerical data consisting of paired observations taken from two numerical variablesOne variable is measured on the vertical axis and the other variable is measured on the horizontal axisScatter p
47、lots are used to examine possible relationships between two numerical variablesDCOVA Copyright 2016,2013,2010 Pearson Education,Inc.Chapter 2,Slide 37Scatter Plot ExampleVolume per dayCost per day231252614029146331603816742170501885519560200DCOVA Copyright 2016,2013,2010 Pearson Education,Inc.Chapte
48、r 2,Slide 38nA Time-Series Plot is used to study patterns in the values of a numeric variable over timenThe Time-Series Plot:nNumeric variable is measured on the vertical axis and the time period is measured on the horizontal axisVisualizing Two Numerical Variables:The Time Series PlotDCOVA Copyrigh
49、t 2016,2013,2010 Pearson Education,Inc.Chapter 2,Slide 39Time Series Plot ExampleYearNumber of Franchises1996 431997 541998 601999 732000 822001 952002 1072003 992004 95DCOVA Copyright 2016,2013,2010 Pearson Education,Inc.Chapter 2,Slide 40nA multidimensional contingency table is constructed by tall
50、ying the responses of three or more categorical variables.nIn Excel creating a Pivot Table to yield an interactive display of this type.nWhile Minitab will not create an interactive table,it has many specialized statistical&graphical procedures(not covered in this book)to analyze&visualize multidime
51、nsional data.Organizing Many Categorical Variables:The Multidimensional Contingency TableDCOVA Copyright 2016,2013,2010 Pearson Education,Inc.Chapter 2,Slide 41Using Excel Pivot Tables To Organize&Visualize Many VariablesA pivot table:nSummarizes variables as a multidimensional summary tablenAllows
52、interactive changing of the level of summarization and formatting of the variablesnAllows you to interactively“slice”your data to summarize subsets of data that meet specified criterianCan be used to discover possible patterns and relationships in multidimensional data that simpler tables and charts
53、 would fail to make apparent.DCOVA Copyright 2016,2013,2010 Pearson Education,Inc.Chapter 2,Slide 42A Multidimensional Contingency Table Tallies Responses Of Three or More Categorical VariablesTwo Dimensional Table Showing The Mean 10 Year Return%Broken Out By Type Of Fund&Risk LevelDCOVAThree Dimen
54、sional Table Showing The Mean 10 Year Return%Broken Out By Type Of Fund,Market Cap,&Risk Level Copyright 2016,2013,2010 Pearson Education,Inc.Chapter 2,Slide 43Data Discovery Methods Can Yield Initial Insights Into Data nData discovery are methods enable the performance of preliminary analyses by ma
55、nipulating interactive summarizationsnAre used to:nTake a closer look at historical or status datanReview data for unusual valuesnUncover new patterns in datanDrill-down is perhaps the simplest form of data discoveryDCOVA Copyright 2016,2013,2010 Pearson Education,Inc.Chapter 2,Slide 44Drill-Down Re
56、veals The Data Underlying A Higher-Level SummaryDCOVAResults of drilling down to the details about smallmarket cap value funds withlow risk.Copyright 2016,2013,2010 Pearson Education,Inc.Chapter 2,Slide 45Some Data Discovery Methods Are Primarily VisualnA treemap is such a methodnA treemap visualize
57、s the comparison of two or more variables using the size and color of rectangles to represent valuesnWhen used with one or more categorical variables it forms a multilevel hierarchy or tree that can uncover patterns among numerical variables.DCOVA Copyright 2016,2013,2010 Pearson Education,Inc.Chapt
58、er 2,Slide 46An Example Of A TreemapDCOVAA treemap of the numerical variables assets(size)and 10-yearreturn percentage(color)for growth and value funds that havesmall market capitalizations and low risk Copyright 2016,2013,2010 Pearson Education,Inc.Chapter 2,Slide 47The Challenges in Organizing and
59、 Visualizing VariablesnWhen organizing and visualizing data need to be mindful of:nThe limits of others ability to perceive and comprehendnPresentation issues that can undercut the usefulness of methods from this chapter.nIt is easy to create summaries thatnObscure the data ornCreate false impressio
60、nsDCOVA Copyright 2016,2013,2010 Pearson Education,Inc.Chapter 2,Slide 48An Example Of Obscuring Data,Information OverloadDCOVA Copyright 2016,2013,2010 Pearson Education,Inc.Chapter 2,Slide 49False Impressions Can Be Created In Many WaysnSelective summarizationnPresenting only part of the data coll
61、ectednImproperly constructed chartsnPotential pie chart issuesnImproperly scaled axesnA Y axis that does not begin at the origin or is a broken axis missing intermediate valuesnChartjunkDCOVA Copyright 2016,2013,2010 Pearson Education,Inc.Chapter 2,Slide 50An Example of Selective Summarization,These
62、 Two Summarizations Tell Totally Different StoriesDCOVACompanyChange from Prior YearCompanyYear 1Year 2Year 3A+7.2%A-22.6%-33.2%+7.2%B+24.4%B-4.5%-41.9%+24.4%C+24.9%C-18.5%-31.5%+24.9%D+24.8%D-29.4%-48.1%+24.8%E+12.5%E-1.9%-25.3%+12.5%F+35.1%F-1.6%-37.8%+35.1%G+29.7%G+7.4%-13.6%+29.7%Copyright 2016,
63、2013,2010 Pearson Education,Inc.Chapter 2,Slide 51How Obvious Is It That Both Pie Charts Summarize The Same Data?DCOVAWhy is it hard to tell?What would you do to improve?Copyright 2016,2013,2010 Pearson Education,Inc.Chapter 2,Slide 52Graphical Errors:No Relative BasisAs received by students.As rece
64、ived by students.Bad Presentation0200300FRSOJRSRFreq.10%30%FRSOJRSRFR=Freshmen,SO=Sophomore,JR=Junior,SR=Senior10020%0%Good PresentationDCOVA Copyright 2016,2013,2010 Pearson Education,Inc.Chapter 2,Slide 53Graphical Errors:Compressing the Vertical AxisGood PresentationQuarterly SalesQuarterly Sales
65、Bad Presentation02550Q1Q2Q3Q4$0100200Q1Q2Q3Q4$DCOVA Copyright 2016,2013,2010 Pearson Education,Inc.Chapter 2,Slide 54Graphical Errors:No Zero Point on the Vertical AxisMonthly Sales36394245JFMAMJ$Graphing the first six months of salesMonthly Sales 0394245JFMAMJ$36Good PresentationsBad PresentationDC
66、OVA Copyright 2016,2013,2010 Pearson Education,Inc.Chapter 2,Slide 55Graphical Errors:Chart Junk,Can You Identify The Junk?Bad PresentationGood PresentationDCOVA Copyright 2016,2013,2010 Pearson Education,Inc.Chapter 2,Slide 56Graphical Errors:Chart Junk,Can You Identify The Junk?Bad PresentationGood PresentationDCOVA Copyright 2016,2013,2010 Pearson Education,Inc.Chapter 2,Slide 57Graphical Errors:Chart Junk,Can You Identify The Junk?DCOVA Copyright 2016,2013,2010 Pearson Education,Inc.Chapter
- 温馨提示:
1: 本站所有资源如无特殊说明,都需要本地电脑安装OFFICE2007和PDF阅读器。图纸软件为CAD,CAXA,PROE,UG,SolidWorks等.压缩文件请下载最新的WinRAR软件解压。
2: 本站的文档不包含任何第三方提供的附件图纸等,如果需要附件,请联系上传者。文件的所有权益归上传用户所有。
3.本站RAR压缩包中若带图纸,网页内容里面会有图纸预览,若没有图纸预览就没有图纸。
4. 未经权益所有人同意不得将文件中的内容挪作商业或盈利用途。
5. 装配图网仅提供信息存储空间,仅对用户上传内容的表现方式做保护处理,对用户上传分享的文档内容本身不做任何修改或编辑,并不能对任何下载内容负责。
6. 下载文件中如有侵权或不适当内容,请与我们联系,我们立即纠正。
7. 本站不保证下载资源的准确性、安全性和完整性, 同时也不承担用户因使用这些下载资源对自己和他人造成任何形式的伤害或损失。