《金融计量经济学(双语版)》全套课件【完整版】
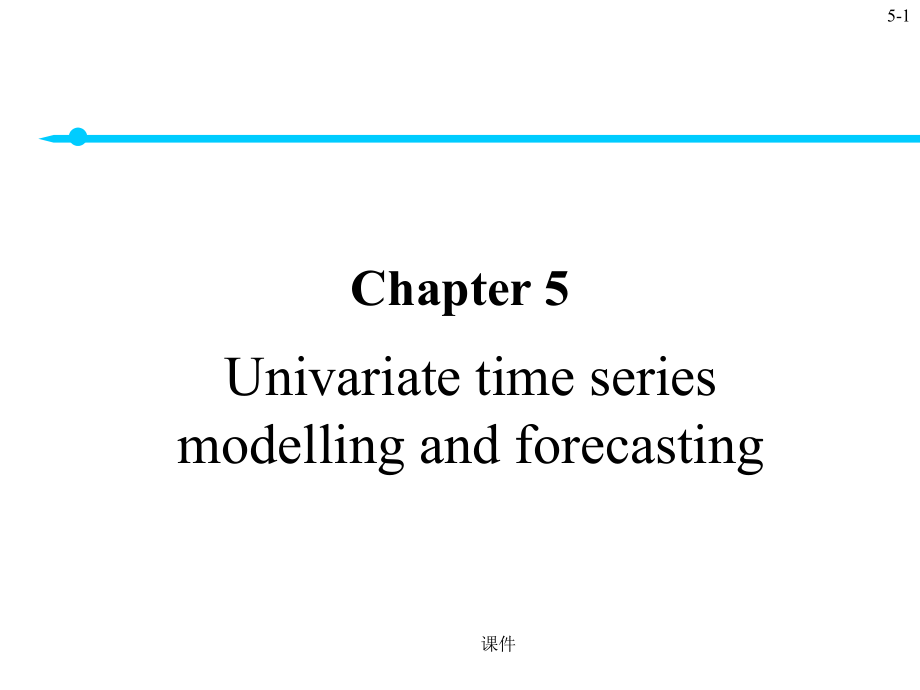


《《金融计量经济学(双语版)》全套课件【完整版】》由会员分享,可在线阅读,更多相关《《金融计量经济学(双语版)》全套课件【完整版】(267页珍藏版)》请在装配图网上搜索。
1、课件5-1Chapter 5Univariate time series modelling and forecasting课件5-21 introduction 单变量时间序列模型单变量时间序列模型 只利用变量的过去信息和可能的误差项的当前和过去值来建模和预测只利用变量的过去信息和可能的误差项的当前和过去值来建模和预测的一类模型的一类模型(设定设定)。与结构模型不同;通常不依赖于经济和金融理论与结构模型不同;通常不依赖于经济和金融理论 用于描述被观测数据的经验性相关特征用于描述被观测数据的经验性相关特征 ARIMA(AutoRegressive Integrated Moving Avera
2、ge)是一类重要的时间序列模型是一类重要的时间序列模型 Box-Jenkins 1976 当结构模型不适用时,时间序列模型却很有用当结构模型不适用时,时间序列模型却很有用 如引起因变量变化的因素中包含不可观测因素,解释变量等观测频率如引起因变量变化的因素中包含不可观测因素,解释变量等观测频率较低。结构模型常常不适用于进行预测较低。结构模型常常不适用于进行预测 本章主要解决两个问题本章主要解决两个问题 一个给定参数的时间序列模型,其变动特征是什么?一个给定参数的时间序列模型,其变动特征是什么?给定一组具有确定性特征的数据,描述它们的合适模型是什么?给定一组具有确定性特征的数据,描述它们的合适模型
3、是什么?课件5-3 A Strictly Stationary ProcessA strictly stationary process is one where For any t1,t2,tn Z,any m Z,n=1,2,A Weakly Stationary ProcessIf a series satisfies the next three equations,it is said to be weakly or covariance stationary1.E(yt)=,t=1,2,.,2.3.t1,t22 Some Notation and ConceptsP ybybP y
4、bybttntmtmnnn,.,.,1111E yytttt()()1221E yytt()()2课件5-4 So if the process is covariance stationary,all the variances are the same and all the covariances depend on the difference between t1 and t2.The moments ,s=0,1,2,.are known as the covariance function.The covariances,s,are known as autocovariance
5、s.However,the value of the autocovariances depend on the units of measurement of yt.It is thus more convenient to use the autocorrelations which are the autocovariances normalised by dividing by the variance:,s=0,1,2,.If we plot s against s=0,1,2,.then we obtain the autocorrelation function(acf)or c
6、orrelogram.Some Notation and Conceptsss0E yE yyE ytttstss()()课件5-5 A white noise process is one with no discernible structure.Thus the autocorrelation function will be zero apart from a single peak of 1 at s=0.如果假设如果假设yt服从标准正态分布服从标准正态分布,则则 approximately N(0,1/T)We can use this to do significance tes
7、ts for the autocorrelation coefficients by constructing a confidence interval.a 95%confidence interval would be given by .If the sample autocorrelation coefficient,falls outside this region for any value of s,then we reject the null hypothesis that the true value of the coefficient at lag s is zero.
8、A White Noise ProcessE yVar yiftrotherwisetttr()()220sT196.1s课件5-6 We can also test the joint hypothesis that all m of the k correlation coefficients are simultaneously equal to zero using the Q-statistic developed by Box and Pierce:where T=sample size,m=maximum lag length The Q-statistic is asympto
9、tically distributed as a .However,the Box Pierce test has poor small sample properties,so a variant has been developed,called the Ljung-Box statistic:This statistic is very useful as a portmanteau(general)test of linear dependence in time series.Joint Hypothesis Testsm2mkkTQ122122mmkkkTTTQ课件5-7 Ques
10、tion:Suppose that we had estimated the first 5 autocorrelation coefficients using a series of length 100 observations,and found them to be(from 1 to 5):0.207,-0.013,0.086,0.005,-0.022.Test each of the individual coefficient for significance,and use both the Box-Pierce and Ljung-Box tests to establis
11、h whether they are jointly significant.Solution:A coefficient would be significant if it lies outside(-0.196,+0.196)at the 5%level,so only the first autocorrelation coefficient is significant.Q=5.09 and Q*=5.26Compared with a tabulated 2(5)=11.1 at the 5%level,so the 5 coefficients are jointly insig
12、nificant.An ACF Example (p234)课件5-8 Let ut(t=1,2,3,.)be a sequence of independently and identically distributed(iid)random variables with E(ut)=0 and Var(ut)=2,then yt=+ut+1ut-1+2ut-2+.+qut-q is a qth order moving average model MA(q).Or using the lag operator notation:Lyt=yt-1 Liyt=yt-i通常,可以将常数项从方程中
13、去掉,而并不失一般性。通常,可以将常数项从方程中去掉,而并不失一般性。3 Moving Average Processes tqittiituLuuLy)(1qqLLLL2211)(课件5-9移动平均过程的性质 Its properties are E(yt)=Var(yt)=0=(1+)2Covariances自相关函数自相关函数 12222.qqsforqsforsqqssss0,.,2,1).(22211课件5-10 Consider the following MA(2)process:where ut is a zero mean white noise process with v
14、ariance .(i)Calculate the mean and variance of Xt(ii)Derive the autocorrelation function for this process(i.e.express the autocorrelations,1,2,.as functions of the parameters 1 and 2).(iii)If 1=-0.5 and 2=0.25,sketch the acf of Xt.Example of an MA Process2211ttttuuuX2课件5-11(i)If E(ut)=0,then E(ut-i)
15、=0 i.So E(Xt)=E(ut+1ut-1+2ut-2)=E(ut)+1E(ut-1)+2E(ut-2)=0 Var(Xt)=EXt-E(Xt)Xt-E(Xt)Var(Xt)=E(Xt)(Xt)=E(ut+1ut-1+2ut-2)(ut+1ut-1+2ut-2)=E +cross-productsBut Ecross-products=0,since Cov(ut,ut-s)=0 for s 0.So Var(Xt)=0=E =Solution222221212tttuuu222221212tttuuu222221222221)1(课件5-12(ii)The acf of Xt 1=EX
16、t-E(Xt)Xt-1-E(Xt-1)=EXt Xt-1=E(ut+1ut-1+2ut-2)(ut-1+1ut-2+2ut-3)=E()=2=EXt-E(Xt)Xt-2-E(Xt-2)=EXt Xt-2=E(ut+1ut-1+2ut-2)(ut-2+1ut-3+2ut-4)=E()=Solution(contd)2221211ttuu221212211)(222tu22课件5-13 3=EXt Xt-3=E(ut+1ut-1+2ut-2)(ut-3+1ut-4+2ut-5)=0 So s=0 for s 2.now calculate the autocorrelations:Solution
17、(contd)0001sss002)1()()1()(2221211222212211011)1()1()(222122222122022课件5-14(iii)For 1=-0.5 and 2=0.25,substituting these into the formulae above gives 1=-0.476,2=0.190.Thus the acf plot will appear as follows:ACF Plot-0.6-0.4-0.200.20.40.60.811.20123456sacf课件5-15 An autoregressive model of order p,A
18、R(p)can be expressed asOr using the lag operator notation:Lyt=yt-1 Liyt=yt-i or or where 4 Autoregressive Processes()(.)LLLLpp 1122tptptttuyyyy.2211pititituyy1pittiituyLy1ttuyL)(课件5-16 平稳性使平稳性使AR模型具有一些很好的性质。如前期误差项对当前模型具有一些很好的性质。如前期误差项对当前值的影响随时间递减。值的影响随时间递减。The condition for stationarity of a general
19、 AR(p)model is that the roots of 特征方程特征方程 all lie outside the unit circle.Example 1:Is yt=yt-1+ut stationary?The characteristic root is 1,so it is a unit root process(so non-stationary)Example 2:p241 A stationary AR(p)model is required for it to have an MA()representation.The Stationary Condition fo
20、r an AR Model 10122zzzpp.课件5-17 States that any stationary series can be decomposed into the sum of two unrelated processes,a purely deterministic part and a purely stochastic part,which will be an MA().For the AR(p)model,ignoring the intercept,the Wold decomposition iswhere,可以证明,算子多项式可以证明,算子多项式R(L)
21、的集合与代数多项式的集合与代数多项式R(z)的集的集合是同结构的,因此可以对算子合是同结构的,因此可以对算子L做加、减、乘和比率运算。做加、减、乘和比率运算。Wolds Decomposition TheoremttuyL)(ttuLy)(3322112211)1()(LLLLLLLpp课件5-18 The moments of an autoregressive process are as follows.The mean is given by*The autocovariances and autocorrelation functions can be obtained by sol
22、ving what are known as the Yule-Walker equations:*If the AR model is stationary,the autocorrelation function will decay exponentially to zero.The Moments of an Autoregressive Processpppppppp.22112211212111ptyE211)(课件5-19 Consider the following simple AR(1)model(i)Calculate the(unconditional)mean of
23、yt.For the remainder of the question,set =0 for simplicity.(ii)Calculate the(unconditional)variance of yt.(iii)Derive the autocorrelation function for yt.Sample AR Problem tttuyy11课件5-20(i)E(yt)=E(+1yt-1)=+1E(yt-1)But also E(yt)=+1(+1E(yt-2)=+1 +12 E(yt-2)=+1 +12(+1E(yt-3)=+1 +12 +13 E(yt-3)An infin
24、ite number of such substitutions would give E(yt)=(1+1+12+.)+1 y0So long as the model is stationary,i.e.,then 1 =0.So E(yt)=(1+1+12+.)=Solution1211tttuyy1111课件5-21(ii)Calculating the variance of yt:*From Wolds decomposition theorem:So long as ,this will converge.Solution(contd)tttuyy11ttuLy)1(1ttuLy
25、11)1(ttuLLy.)1(221111.22111ttttuuuy课件5-22Var(yt)=Eyt-E(yt)yt-E(yt)but E(yt)=0,since we are setting =0.Var(yt)=E(yt)(yt)*有简便方法=E =E=E=Solution(contd).2211122111ttttttuuuuuu).(224121212productscrossuuuttt.)(224121212tttuuu.2412212uuu.)1(41212u)1(212u课件5-23(iii)Turning now to calculating the acf,first
26、calculate the autocovariances:(*用简便方法)1=Cov(yt,yt-1)=Eyt-E(yt)yt-1-E(yt-1)1=Eytyt-1 1=E =E =Solution(contd).)(22111tttuuu.)(321211tttuuu.2231211productscrossuutt.25123121)1(2121课件5-24Solution(contd)For the second autocorrelation coefficient,2=Cov(yt,yt-2)=Eyt-E(yt)yt-2-E(yt-2)Using the same rules as
27、 applied above for the lag 1 covariance2=Eyt yt-2 =E =E =.)(22111tttuuu.)(421312tttuuu.23412221productscrossuutt.241221.)1(4121221)1(21221课件5-25Solution(contd)If these steps were repeated for 3,the following expression would be obtained 3=and for any lag s,the autocovariance would be given by s=The
28、acf can now be obtained by dividing the covariances by the variance:)1(21231)1(2121s课件5-26Solution(contd)0=1=2=3=s=1001212212101)1()1(212122122102)1()1(31s1课件5-27 Measures the correlation between an observation k periods ago and the current observation,after controlling for observations at intermedi
29、ate lags(i.e.all lags 2,the eqn is over-identifiedIf#excluded variables 0.The two simplest forecasting“methods”1.Assume no change:f(yt+s)=E(yt+s t)=yt,对随机游对随机游动是最优预测动是最优预测2.Forecasts are the long term average f(yt+s)=对平对平稳序列来说优于稳序列来说优于1。y课件5-108Models for Forecasting 对于时间序列预测,时间序列模型通常优于结构模型对于时间序列预测,
30、时间序列模型通常优于结构模型 Structural modelse.g.To forecast y,we require the conditional expectation of its future value:But what are etc.?We could use etc.,so =!tktkttuxxy221tktktttuxxEyE2211ktktxExE221)(2tx2xkktxxyE221y课件5-109Models for Forecasting Time Series ModelsThe current value of a series,yt,is modelle
31、d as a function only of its previous values and the current value of an error term(and possibly previous values of the error term).Models include:simple unweighted averages exponentially weighted averages ARIMA models Non-linear models e.g.threshold models,GARCH,bilinear models,etc.课件5-110The foreca
32、sting model typically used is of the form:where ft,s=yt+s,s 0;ut+s=0,s 0 =ut+s,s 0 Forecasting with ARMA Modelsqjjstjpiististuff11,课件5-111 An MA(q)only has memory of length q.e.g.say we have estimated an MA(3)model:yt=+1ut-1+2ut-2+3ut-3+utyt+1=+1ut+2ut-1+3ut-2+ut+1yt+2=+1ut+1+2ut+3ut-1+ut+2yt+3=+1ut
33、+2+2ut+1+3ut+ut+3 We are at time t and we want to forecast 1,2,.,s steps ahead.We know yt,yt-1,.,and ut,ut-1,.。Forecasting with MA Models 课件5-112ft,1=yt+1,t=E(yt+1 t)=E(+1ut+2ut-1+3ut-2+ut+1)=+1ut+2ut-1+3ut-2 ft,2=yt+2,t=E(yt+2 t)=E(+1ut+1+2ut+3ut-1+ut+2)=+2ut+3ut-1 ft,3=yt+3,t=E(yt+3 t)=E(+1ut+2+2u
34、t+1+3ut+ut+3)=+3ut ft,4=yt+4,t=E(yt+4 t)=ft,s=yt+s,t=E(yt+s t)=s 4Forecasting with MA Models课件5-113 自回归模型有无限记忆。自回归模型有无限记忆。Say we have estimated an AR(2)yt=+1yt-1+2yt-2+utyt+1=+1yt+2yt-1+ut+1yt+2=+1yt+1+2yt+ut+2yt+3=+1yt+2+2yt+1+ut+3 ft,1=E(yt+1 t)=E(+1yt+2yt-1+ut+1)=+1E(yt)+2E(yt-1)=+1yt+2yt-1 ft,2=
35、E(yt+2 t)=E(+1yt+1+2yt+ut+2)=+1E(yt+1)+2E(yt)=+1 ft,1+2yt Forecasting with AR Models课件5-114ft,3=E(yt+3 t)=E(+1yt+2+2yt+1+ut+3)=+1E(yt+2)+2E(yt+1)=+1 ft,2+2 ft,1 We can see immediately that ft,4=+1 ft,3+2 ft,2 etc.,so ft,s=+1 ft,s-1+2 ft,s-2 Can easily generate ARMA(p,q)forecasts in the same way.Fore
36、casting with AR Models 课件5-115For example,say we predict that tomorrows return on the FTSE will be 0.2,but the outcome is actually-0.4.Is this accurate?Define ft,s as the forecast made at time t for s steps ahead(i.e.the forecast made for time t+s),and yt+s as the realised value of y at time t+s.Som
37、e of the most popular criteria for assessing the accuracy of time series forecasting techniques are:*Mean absolute percentage error:How can we test whether a forecast is accurate or not?TTtTtthsststyyTTfyhMSE1121,112,)(11)(1TTttTtthsstststyyyTTyfyhMAPE111,11,1100100课件5-116 It has,however,also recent
38、ly been shown(Gerlow et al.,1993)that the accuracy of forecasts according to traditional statistical criteria are not related to trading profitability.A measure more closely correlated with profitability:%correct sign predictions wherezt+s=1 if(yt+s.ft,s)0zt+s=0 otherwise 对于大的误差的惩罚程度由小到大依次为对于大的误差的惩罚
39、程度由小到大依次为符号预测符号预测MAEMSEHow can we test whether a forecast is accurate or not?hsstzh11课件5-117 Given the following forecast and actual values,calculate the MSE,MAE and percentage of correct sign predictions:MSE=0.079,MAE=0.180,%of correct sign predictions=40Forecast Evaluation Example Steps Ahead Fore
40、cast Actual 1 0.20-0.40 2 0.15 0.20 3 0.10 0.10 4 0.06-0.10 5 0.04-0.05 课件5-118Statistical Versus Economic or Financial loss functions Statistical evaluation methods may not be appropriate.How well does the forecast perform in doing the job we wanted it for?Limits of forecasting:What can and cannot
41、be forecast?All statistical forecasting models are essentially extrapolative Forecasting models are prone to break down around turning points Series subject to structural changes or regime shifts cannot be forecast Predictive accuracy usually declines with forecasting horizon Forecasting is not a su
42、bstitute for judgement The Usually Optimal Approach To use a statistical forecasting model built on solid theoretical foundations supplemented by expert judgements and interpretation.课件5-119Chapter 4 Further issues with the classical linear regression model课件5-120本章目标继续讨论古典线性回归模型 了解确定模型优劣的各种方法 普通最小二
43、乘法OLS可能遇到的各种问题及其处理课件5-1211 Goodness of Fit Statistics We would like some measure of how well our regression model actually fits the data.*We have goodness of fit statistics to test this:i.e.how well the sample regression function(srf)fits the data.The most common goodness of fit statistic is known a
44、s R2.One way to define R2 is to say that it is the square of the correlation coefficient between y and .For another explanation,recall that what we are interested in doing is explaining the variability of y about its mean value,i.e.the total sum of squares,TSS总变差总变差:We can split the TSS into two par
45、ts,the part which we have explained(known as the explained sum of squares,ESS)and the part which we did not explain using the model(the RSS)*.yttyyTSS2y课件5-122Defining R2 That is,TSS =ESS +RSS Goodness of fit statistic is R2 must always lie between zero and one.To understand this,consider two extrem
46、esRSS=TSS i.e.ESS=0 soR2=ESS/TSS=0ESS=TSS i.e.RSS=0 so R2=ESS/TSS=1RESSTSS2RESSTSSTSSRSSTSSRSSTSS21ttttttuyyyy222课件5-123The Limit Cases:R2=0 and R2=1 ty y tx ty tx课件5-124Problems with R2 as a Goodness of Fit Measure1.R2 is defined in terms of variation about the mean of y so that if a model is repar
47、ameterised(rearranged)and the dependent variable changes,R2 will change.2.R2 never falls if more regressors are added to the regression,e.g.consider:Regression 1:yt=1+2x2t+3x3t+utRegression 2:y=1+2x2t+3x3t+4x4t+utR2 will always be at least as high for regression 2 relative to regression 1.3.R2 quite
48、 often takes on values of 0.9 or higher for time series regressions.课件5-125Adjusted R2 In order to get around these problems,a modification is often made which takes into account the loss of degrees of freedom associated with adding extra variables.This is known as ,or adjusted R2:So if we add an ex
49、tra regressor,k increases and unless R2 increases by a more than offsetting amount,will actually fall.可用于决定某一变量是否应包括在模型中。可用于决定某一变量是否应包括在模型中。There are still problems with the criterion:1.A“soft”rule。如果只按这一标准选择模型,模型中会。如果只按这一标准选择模型,模型中会 包含很多边际显著或不显著的变量。包含很多边际显著或不显著的变量。2.No distribution for or R2。从而不能进行
50、假设。从而不能进行假设检验,以比较一个模型的拟合优度是否显著高于另一个检验,以比较一个模型的拟合优度是否显著高于另一个模型。模型。2R)1(1122RkTTR2R2R2R课件5-1262 A Regression Example:Hedonic House Pricing Models Hedonic models are used to value real assets,especially housing,and view the asset as representing a bundle of characteristics.Des Rosiers and Thrialt(1996)
51、consider the effect of various amenities on rental values for buildings and apartments 5 sub-markets in the Quebec area of Canada.The rental value in Canadian Dollars per month(the dependent variable)is a function of 9 to 14 variables(depending on the area under consideration).The paper employs 1990
52、 data,and for the Quebec City region,there are 13,378 observations,and the 12 explanatory variables are:课件5-127LnAGE-log of the apparent age of the propertyNBROOMS-number of bedroomsAREABYRM-area per room(in square metres)ELEVATOR-a dummy variable=1 if the building has an elevator;0 otherwiseBASEMEN
53、T-a dummy variable=1 if the unit is located in a basement;0 otherwiseOUTPARK-number of outdoor parking spacesINDPARK-number of indoor parking spacesNOLEASE-a dummy variable=1 if the unit has no lease租借权租借权 attached to it;0 otherwiseLnDISTCBD-log of the distance in kilometres to the central business
54、districtSINGLPAR-percentage of single parent families in the area where the building standsDSHOPCNTR-distance in kilometres to the nearest shopping centreVACDIFF1-vacancy difference between the building and the census figure课件5-128Hedonic House Pricing Models:Examine the signs and sizes of the coeff
55、icients.The coefficient estimates themselves show the Canadian dollar rental price per month of each feature of the dwelling.The coefficient on the constant term often has little useful interpretation.课件5-129Hedonic House Price ResultsDependent Variable:Canadian Dollars per Month Variable Coefficien
56、t t-ratio A priori sign expected Intercept 282.21 56.09+LnAGE -53.10 -59.71-NBROOMS 48.47 104.81+AREABYRM 3.97 29.99+ELEVATOR 88.51 45.04+BASEMENT -15.90 -11.32-OUTPARK 7.17 7.07+INDPARK 73.76 31.25+NOLEASE-16.99 -7.62-LnDISTCBD 5.84 4.60-SINGLPAR -4.27-38.88-DSHOPCNTR-10.04 -5.97-VACDIFF1 0.29 5.98
57、-Notes:Adjusted R2=0.65l;regression F-statistic=2082.27.Source:Des Rosiers and Thrialt (1996).Reprinted with permission of the American Real Estate Society.课件5-1303 Tests of Non-nested Hypotheses All of the hypothesis tests concluded thus far have been in the context of“nested”models.But what if we
58、wanted to compare between the following models?We could use R2 or adjusted R2,but what if the number of explanatory variables were different across the 2 models?还有一些其他的“信息准则”。但很多情况下,不同的准则可能导致选取不同的模型。An alternative approach is an encompassing test,based on examination of the hybrid model:ttttttvxyuxy
59、321221:2Model:1Modelttttwxxy33221:3Model课件5-131Tests of Non-nested Hypotheses There are 4 possible outcomes when Model 3 is estimated:2 is significant but 3 is not,model 1 3 is significant but 2 is not,model 2 2 and 3 are both statistically significant,Model 3 Neither 2 nor 3 are significant,none;ot
60、her method Problems with encompassing approach Hybrid model may be meaningless Possible high correlation between x2 and x3.课件5-1324 Violation of the Assumptions of the CLRM Recall that we assumed of the CLRM disturbance terms:1.E(ut)=02.Var(ut)=2 3.Cov(ui,uj)=04.The X matrix is non-stochastic or fix
61、ed in repeated samples5.ut N(0,2)课件5-133Investigating Violations of the Assumptions of the CLRM We will now study these assumptions further:-How we test for violations-Causes-Consequencesin general we could encounter any combination of 3 problems:-the coefficient estimates are wrong-the associated s
62、tandard errors are wrong-the distribution that we assumed for thetest statistics will be inappropriate-Solutions-the assumptions are no longer violated-we work around the problem so that weuse alternative techniques which are still valid课件5-134Statistical Distributions for Diagnostic Tests Often,an
63、F-and a 2-version of the test are available.The F-test version(Wald test)involves estimating a restricted and an unrestricted version of a test regression and comparing the RSS.The 2-version is sometimes called an“LM”test,and only has one degree of freedom parameter:the number of restrictions being
64、tested,m.Asymptotically,the 2 tests are equivalent since the 2 is a special case of the F-distribution:For small samples,the F-version is preferable.kTkTmFmmas,2课件5-1355 Assumption 1:E(ut)=0 Assumption that the mean of the disturbances is zero.For all diagnostic tests,we cannot observe the disturban
65、ces and so perform the tests of the residuals.The mean of the residuals will always be zero provided that there is a constant term in the regression.没有常数项时,可能导致斜率估计值出现偏差。没有常数项时,可能导致斜率估计值出现偏差。R2 可能是负的。可能是负的。课件5-1366 Assumption 2:Var(ut)=2 k,T2k.An alternative formulation is the predictive failure tes
66、t.What we do with the predictive failure test is estimate the regression over a“long”sub-period(i.e.most of the data)and then we predict values for the other period and compare the two.-Run the regression for the whole period(the restricted regression)and obtain the RSS-Run the regression for the“large”sub-period and obtain the RSS(called RSS1).Note we call the number of observations T1 where T2=number of observations we are attempting to“predict”.The test statistic will follow an F(T2,T1-k).*21
- 温馨提示:
1: 本站所有资源如无特殊说明,都需要本地电脑安装OFFICE2007和PDF阅读器。图纸软件为CAD,CAXA,PROE,UG,SolidWorks等.压缩文件请下载最新的WinRAR软件解压。
2: 本站的文档不包含任何第三方提供的附件图纸等,如果需要附件,请联系上传者。文件的所有权益归上传用户所有。
3.本站RAR压缩包中若带图纸,网页内容里面会有图纸预览,若没有图纸预览就没有图纸。
4. 未经权益所有人同意不得将文件中的内容挪作商业或盈利用途。
5. 装配图网仅提供信息存储空间,仅对用户上传内容的表现方式做保护处理,对用户上传分享的文档内容本身不做任何修改或编辑,并不能对任何下载内容负责。
6. 下载文件中如有侵权或不适当内容,请与我们联系,我们立即纠正。
7. 本站不保证下载资源的准确性、安全性和完整性, 同时也不承担用户因使用这些下载资源对自己和他人造成任何形式的伤害或损失。