ASReml软件讲义ASRemlworkshop学习教案
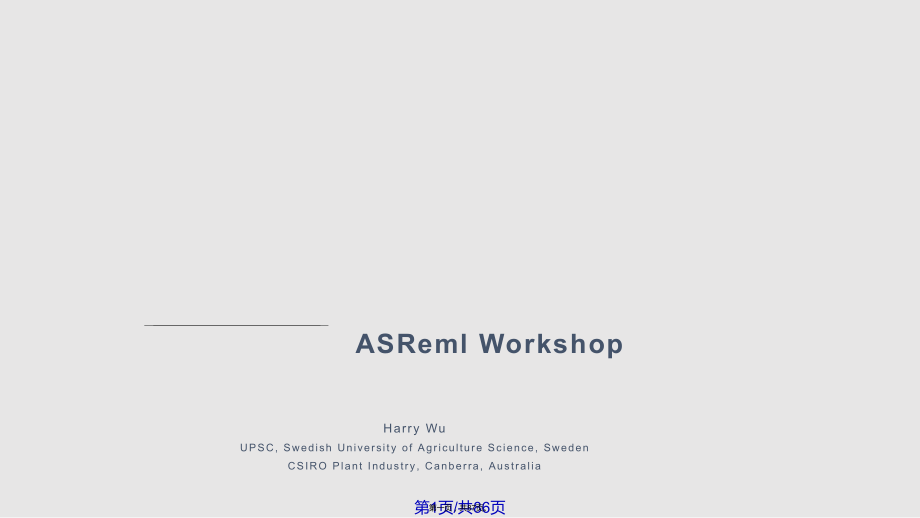


《ASReml软件讲义ASRemlworkshop学习教案》由会员分享,可在线阅读,更多相关《ASReml软件讲义ASRemlworkshop学习教案(87页珍藏版)》请在装配图网上搜索。
1、ASReml WorkshopHarry WuUPSC, Swedish University of Agriculture Science, SwedenCSIRO Plant Industry, Canberra, Australia第1页/共86页第一页,共87页。 Workshop Outline1. Linear model2. Mixed linear model3. Breeding values4. ASReml and ConTEXT Primer5. Example of full-sib mating6. Example of diallel mating7. Row-C
2、olumn design8. Longitudinal data9. Spatial analysis第2页/共86页第二页,共87页。1. What Is a Linear Model?Y = b1X1 + b 2X2 + b 3X3 +. e A linear combination of things (X)multiplied by some coefficients (b) thatexplain the data (Y), with some error (e) X can be The mean A covariate A factor Want to estimate the
3、coefficients usingsome data第3页/共86页第三页,共87页。Put Experiment into a Linear Model Any experiment can be described by a linear model.How can seed weight (xi) and family (2 families - f1 and f2) affect seedling growth (yi)? The relationship y with x and f can be expressed using a set of simultaneous equa
4、tions for four seedlings from two families as:y1 = + cx1 + f1 + e1 y2 = + cx2 + f1 + e2 y3 = + cx3 + f2 + e3 y4 = + cx4 + f2 + e4第4页/共86页第四页,共87页。Put the Linear Model into Matrix42432321211143212143214321101101011011efcxefcxefcxefcxeeeffcxxxxyyyyeXYYou can get the OLS solutionby assuming residuals a
5、re iid (independently and identically distributed) YXX)X(1第5页/共86页第五页,共87页。Useful Matrix OperationsYXX)X(1TransposeMultiplicationTraceDeterminantInverseDirect sum ( )Direct product ( )第6页/共86页第六页,共87页。2. What Is Mixed Linear Model A combination of fixed effects and random effects. Fixed: where there
6、 are different populations (levels), each with its own mean. We are mostly interested in estimating the means. Random: the levels are random samples from one population. We are interested in the variances (although we might want prediction for the levels). Very powerful at dealing with unbalanced da
7、ta What are some fixed and random effects?第7页/共86页第七页,共87页。An Example of Mixed Linear ModelMixed linear modelA family trial in a replicated experiment:1. To examine whether there are differences among families2. Relative importance of variation between-family and between-trees.For the first objectiv
8、e, we can treat family effect either fixed or random, but for the second objective, we have to treat j as random. yijl = + i + j + ij + eijlfixedrandomj independently and identically distributed (IID)ij - IIDeijl - IID第8页/共86页第八页,共87页。Mixed Linear ModelPut the scalar model into matrix formeZuX Y)(an
9、d)(R0eG0u,),(),(RZGZX VX YandThe BLUE of is estimated asand BLUP of u is)(111YVXXVX)(1XYGZVu第9页/共86页第九页,共87页。Solution of Mixed Linear ModelActual solution is through the standard Mixed Model Equation (MME) eZuX YThis Mixed Model can be applied in various genetic trials in forest species.YRZYRXuGZRZX
10、RZZRXXRX1111111第10页/共86页第十页,共87页。Traditional Mixed Linear Model in Tree BreedingIn traditional analysis of genetic trial, such as half-sib, full-sib families eZuXYSuch simple mixed model can be analyzed by most commercial software: SAS GLM)(and)(R0eG0u,anaIRIG222222000000and000000eeefff第11页/共86页第十一页
11、,共87页。Complex Mixed Linear ModelHowever, for individual tree model, or multiple-trait, or repeated measure,or spatial model with special variance structure eZuXYSuch complex mixed model can only be analyzed by specialized software: SAS Mixed, ASReml)(and)(R0eG0u,32312322113121covcovcovcovcovcovandco
12、vcovcovcovcovcov223231232221131221eeeeeeeeRG第12页/共86页第十二页,共87页。Solution of Mixed Linear ModelFor solutions need R and G, use and These are the variance of each error and each random effect For simple situations so the variances are needed. They are unknown, but can be estimated Various methods REML
13、is popular ASReml Estimates (co-)variances Solves mixed model equationsRGanaIRIG222222000000and000000eeefff第13页/共86页第十三页,共87页。REML Restricted (or Residual) MaximumLikelihood Likelihood of the fixed effects (b) and thedata variance (V), given the data (y). A transformation of the data so that fixedef
14、fects are excluded Log Likelihood is maximised by iterativemethods第14页/共86页第十四页,共87页。ASRemlASReml is a statistical package that fits linear mixed models using Residual MaximumLikelihood (REML).Uses average information algorithm toclimb the likelihood mountain第15页/共86页第十五页,共87页。Likelihood Ratio Test
15、Fixed effects must be the same in both models Hierarchical models only For single variances2 * D Log Likelihood where D Log Likelihood is the LL difference with and without the effect (Section 2.5)For multiple variancesFor correlations against 0 against 125 . 025 . 0n2n25 . 0n第16页/共86页第十六页,共87页。Othe
16、r Model Comparators Non-hierarchical models Akaike Information Criterion Minimise AIC = -2*LogL+2p (p=no. vcs) Bayes Information Criterion Minimise BIC = -2*LogL+p*log(dfe)第17页/共86页第十七页,共87页。3. Basic Concept of Breeding ValueConsidering a simplest case with individual trees without any replication,
17、with linear model as yi = + i + ei where i is the additive genetic value of individual.kaaa21100000100001,111uZXA is the additive genetic relationship matrix with Aij = 2 * and the is the coefficient of coancestry between tree i and j.AG2212222111211222221222211121AkkkkkkAaaaaaaaaaAAAAAAAAAkkkkkkThe
18、 variance and covariance of u is第18页/共86页第十八页,共87页。Basic Concept of Breeding ValueYZYXuAZZXZZXXX1where = E2/A2 = (1-h2)/h2, and since R-1= E-2 I, and G-1= A-2 A-1yynni1uAI111Substitute X, Z, this reduces to If we assume residual errors are unrelated between individuals, R= E2 I, the MME reduced to 第
19、19页/共86页第十九页,共87页。Basic Concept of Breeding ValueA numerical example of five individuals in two generations1253496109754321yyyyyy1. Build A matrix assuming tree 1, 2 and 3 are non-inbred and unrelated 2. Use previous equation to calculate breeding value for tree 1 to 5 assuming h2=0.5 and 0.2 () 第20
20、页/共86页第二十页,共87页。Basic Concept of Breeding ValueA numerical example of five individuals in two generations1253496109754321yyyyyy第21页/共86页第二十一页,共87页。Basic Concept of Breeding ValueA numerical example of five individuals in two generations12534yynni1uAI111= E2/A2 = (1-h2)/h2第22页/共86页第二十二页,共87页。4. ASRem
21、l primer Prepare the data (using a spreadsheet or data base program) Export that data as a .csv file from Excel Prepare a job file with filename extension .as Run the job file with ASReml Review the various output files Revise the job and re-run it, or Extract results for your reportExamples:第23页/共8
22、6页第二十三页,共87页。ConTEXT Primer2. Copy ASREML.chl to the c:/program files/context/highlightersfolder3. Restart ConText4. Set up Context to run ASRemla. From the menu select “Options” “Environment Options”b. Go to the tab “Execute Keys”c. Under the “User exec keys” box select “Add”d. For the file extensi
23、ons, enter “as, asc”e. In the “User exec keys” box, under “as,asc”, select “F9”f. Fill in the other information as below:ii. Parameters: %fiii. Hint: Run ASRemliv. Capture console output: yesv. Scroll console to last line: yes第24页/共86页第二十四页,共87页。Case Analysis5. Full-sib (2-way treatment in a RCB)6.
24、Diallel mating structure7. Row-column design8. Longitudinal data structure9. Spatial data analysis第25页/共86页第二十五页,共87页。5. Full-sib (2-way treatment in RCB)The phenotypic value can be derived from:MDDMMDMDDMFSSCAGCAGCAIDAAP2/22/2DMFSMDAAPSCANon-additive SCAMD (. dominance and epistasis) effects can be
25、 calculated as: 第26页/共86页第二十六页,共87页。Full-sib (2-way treatment in RCB)Parental model for full-sib families:ijkljkkjiijkemddmryIndividual-tree model for full-sib families:ijkjijkiijkemdtry第27页/共86页第二十七页,共87页。Full-sib (2-way treatment in RCB)Heritability and dominance proportion for a parental model:)/
26、()*4(/22222222emddmmPAh)/()*4(/22222222emddmmdPDD2241Am2241Ad2241Dmd2224321DAe)/()(/2222222efrttPAh22Atand for an individual tree model :第28页/共86页第二十八页,共87页。Full-sib (Factorial treatment in RCB)Single-pair matings第29页/共86页第二十九页,共87页。Full-sib (Factorial treatment in RCB)Full-factorial matings第30页/共86
27、页第三十页,共87页。Full-sib (Factorial treatment in RCB)Tester (male) design:第31页/共86页第三十一页,共87页。Full-sib (Factorial treatment in RCB)Example 2: RAD200p full-factorial design analyses:Trial ID Trial NameFSHS (Ch.) TreesLatLongAltPlanted200 Dandongadale, Blades 4x4 Factorial16-480-3649146393206/1986第32页/共86页
28、第三十二页,共87页。Full-sib (Factorial treatment in RCB)第33页/共86页第三十三页,共87页。6. Diallel Mating Structure Same parent can be male and female Four types of diallel mating method Method 1 - full diallel including self and reciprocal Method 2 - half diallel with self Method 3 full diallel without self Method 4 h
29、alf diallelSex 123456 1XXXXX 2XXXX3XXX 4XX 5X 6 第34页/共86页第三十四页,共87页。Diallel Mating StructureUniqueness of diallel same individual used for male and female In the Z sub-matrix for additive effect, not a diagonal sub-matrixThe model without design structure isy= + gi + gj + sij + eijkwhere gi and gj a
30、re the ith and jth general combining ability (GCA), and sij is the ijkth SCA effect. 第35页/共86页第三十五页,共87页。Diallel Mating StructureUniqueness of diallel same individual used for male and female In the Z sub-matrix for non-additive effect, a diagonal sub-matrixThe model without design structure isy= +
31、gi + gj + sij + eijkwhere gi and gj are the ith and jth general combining ability (GCA), and sij is the ijkth SCA effect. 第36页/共86页第三十六页,共87页。Diallel Mating Structure Example using SAS Mixed and ASReml Without missing crosses, Diallel-SAS and Diallel-SAS05 With missing crosses, DIAFIXED and DIARAND
32、(Wu and Matheson)Diallel analyses - Hayman diallel Methed4 data (1954) rep 2 mother !I father !AS mother yS5E_DiallelHaymanM4.txt !skip 1第37页/共86页第三十七页,共87页。Diallel Mating Structure第38页/共86页第三十八页,共87页。Diallel Mating StructureBLUP for GCA and first 8 SCA listed 第39页/共86页第三十九页,共87页。Diallel Mating Stru
33、ctureAlso can be analysed by individual tree modelDiallel analyses - Hayman diallel Methed4 data (1954) genotype !P rep 2 mother 7 father 8 yS5E_DiallelHaymanM4p.txt !skip 1S5E_DiallelHaymanM4.txt !skip 1 !AISING# Diallel individual tree modely mu rep !r genotype 第40页/共86页第四十页,共87页。Diallel Mating St
34、ructureBLUP for GCA compared using diallel model and individual tree model第41页/共86页第四十一页,共87页。7. Row-column DesignTreatment StructureDesign StructureRandomizationExperimental DesignHalf-sibFactorial full-sibDiallel matingProv/familyRCBSplit-plot Incomplete blockLattice design Latin square Row-column
35、Overall Aim: reducing residual error第42页/共86页第四十二页,共87页。Row-column Design We use a row-column design to demonstrate incomplete block design. The example is based on a CSIRO Casuarina trial. The design (see following figure for layout) There were 60 seedlots, A latinized row-column design for 4 repli
36、cates generated, each with six rows and 10 columns. Only 59 seedlots were planted. Each plot consisted of 5 x 5 trees. 第43页/共86页第四十三页,共87页。5 Row-column Design第44页/共86页第四十四页,共87页。Row-column DesignLinear model for RCB yijm = + i + j + eijm Linear model for row and columnyijklm = + i + j + ck+ cik + ri
37、l + eijklm Analyses were done by RCB, and row-column to demonstrate the extra efficiency using incomplete blocks.第45页/共86页第四十五页,共87页。Row-column DesignCasuarina Row-Column Design Model Repl 4 Row 6 Column 10 Inoc 2 Prov 59 !I Country 18 DBH Casuarina.csv !SKIP 1 !DOPART 3 !PART 1 # RCB analysisDBH mu
38、 Repl Prov!PART 2 # Row-Column fixedDBH mu Repl Column Repl.Row Repl.Column Prov!PART 3 # Row-Column random第46页/共86页第四十六页,共87页。Row-column DesignThe best provenance changed第47页/共86页第四十七页,共87页。Row-column DesignThe prediction error reduced第48页/共86页第四十八页,共87页。8. Longitudinal Data Structure Repeated meas
39、ures on time and space on the same subjects 第49页/共86页第四十九页,共87页。Longitudinal Data Structure 第50页/共86页第五十页,共87页。Longitudinal Data StructureThe mixed linear model is:ijkijjiijkemffmy第51页/共86页第五十一页,共87页。Longitudinal Data StructureUnstructured (US) co-variance matrix between n measurements n(n + 1)/2 pa
40、rameters to estimate.)()()()()()()()()()()(131332212131211nnnmVarmmCovmVarmmCovmmCovmVarmmCovmmCovmmCovmmCovmVarRi.e. for n = 10 measurements there are 55 parameters to estimate 第52页/共86页第五十二页,共87页。Longitudinal Data StructureParameters can be reduced with a structured variance and covariances: AR1 c
41、orrelation structure has only one correlation parameter 11111212nn 2eR第53页/共86页第五十三页,共87页。Longitudinal Data StructureExamples using 36 radiata families:1. Modelling AR1 correlation structure2. Random regression model第54页/共86页第五十四页,共87页。Longitudinal Data Structure!PART 2D80 D85 D90 D95 Trait !r Trait
42、.Blk Trait.Family !f mv1 2 20 !S2=1Trait 0 DIAG 93 188 283 421Trait.Blk 2Trait 0 DIAG 1 5 10 17 !GPBlkTrait.Family 2Trait 0 DIAG 5 20 30 50 !GPFamilyFirst, regard each measurement as independent trait and estimate variance for residual, block and family第55页/共86页第五十五页,共87页。Longitudinal Data Structure
43、!PART 2D80 D85 D90 D95 Trait !r Trait.Blk Trait.Family !f mv1 2 20 !S2=1Trait 0 DIAG 93 188 283 421Trait.Blk 2Trait 0 DIAG 1 5 10 17 !GPBlkTrait.Family 2Trait 0 DIAG 5 20 30 50 !GPFamilyFirst, regard each measurement as independent trait and estimate variance for residual, block and family第56页/共86页第
44、五十六页,共87页。Longitudinal Data StructureSource Model terms Gamma Component Comp/SE % C Residual DIAGonal 1 108.362 108.362 16.32 0 U Residual DIAGonal 2 194.844 194.844 16.30 0 U Residual DIAGonal 3 289.454 289.454 16.27 0 U Residual DIAGonal 4 428.241 428.241 16.17 0 U Trait.Blk DIAGonal 1 0.703281 0.
45、703281 0.45 0 P Trait.Blk DIAGonal 2 4.14438 4.14438 1.04 0 P Trait.Blk DIAGonal 3 9.32464 9.32464 1.31 0 P Trait.Blk DIAGonal 4 15.6495 15.6495 1.39 0 P Trait.Family DIAGonal 1 4.64890 4.64890 1.67 0 P Trait.Family DIAGonal 2 17.5049 17.5049 2.40 0 P Trait.Family DIAGonal 3 33.1926 33.1926 2.63 0 P
46、 Trait.Family DIAGonal 4 55.7703 55.7703 2.74 0 P Most Blk effects are not significant第57页/共86页第五十七页,共87页。Longitudinal Data StructureSo we focused on correlated residual and family effect!PART 5D80 D85 D90 D95 Trait Rep !r Trait.Family !f mv #1 2 10 !S2=1Trait 0 US !+10Trait.Family 2Trait 0 AR1H 0.9
47、 5 18 33 55Family第58页/共86页第五十八页,共87页。Longitudinal Data StructureSo we focused on correlated residual and family effect12=5.04, 22=21.06, 32=40.25, 42Covariance/Variance/Correlation Matrix UnStructured Residual 1 234 197.56 0.9221 0.8347 0.7419 2128.7 199.6 0.9705 0.9103 3142.6 237.2 299.3 0.9775 第59
48、页/共86页第五十九页,共87页。Longitudinal Data Structure!PART 2Diam mu Rep Meas !r pol(Meas,2).Familypredict Meas FamilyFitting random regression for a two-degree polynomial2321xxy第60页/共86页第六十页,共87页。Longitudinal Data StructureFitting random regression for a two-degree polynomial3 parameters for each family?.123
49、第61页/共86页第六十一页,共87页。9. Spatial Data Analysis “Things closer together are morelikely to be more similar”Saint Ronald A. Fisher, 近朱者赤(jn zh(jn zh zhzh ch), ch), 近墨者黑第62页/共86页第六十二页,共87页。Spatial Data Analysis Account for macro (trend) or micro-environment variability within site and increase power for d
50、etecting differences among genotypes 第63页/共86页第六十三页,共87页。Spatial Data AnalysisTypes of spatial variationEnvironment of field trials in forestry is usually highly variable-Global pattern a gradient or large scale trend (slope, soil depth, old road)-Local variation patchy pattern (variation in soil or
51、 microclimate)-Extraneous variation non-spatial variation (planting procedure, labelling mistakes or measurement errors)第64页/共86页第六十四页,共87页。Spatial Data AnalysisMethods for spatial analysesAdjustments of data for global and local variation: Nearest neighbour analyses rij=0.5(ri-1,j + ri+1,j) and cij
52、=0.5(ci,j-1 + r i, j+1) Row-column latinised design fitted within replications as random effects permitting different paterns within blocks (i.e. interblock information recovery) Kriging interpolation method smooth surfaces of BLUPs on a spatial grid: 1) variogram - optimal interpolation weights2) i
53、nterpolation 第65页/共86页第六十五页,共87页。Spatial Data Analysis Semivariance and VariogramThe semi-variance (h) was calculated as:21)()(21)(nihiiszsznhSemivariance increases with distance if there isa spatial association : Variogram第66页/共86页第六十六页,共87页。Spatial Data AnalysiseZuX YThe focus of spatial analyses
54、is to model the big R YRZYRXuGZRZXRZZRXXRX1111111第67页/共86页第六十七页,共87页。Spatial Data Analysis Modelling of the autoregressive processOrdinary least squares errorsAR1 - One-dimensional auto-correlated component in field order1000010000100001)(22eeIVareRiii1=0.9=0.6=0.3第68页/共86页第六十八页,共87页。Spatial Data An
55、alysis Modelling of the autoregressive processAR1 - One-dimensional auto-correlated component in field orderiii11111121222nneIR第69页/共86页第六十九页,共87页。Spatial Data Analysis Modelling of the autoregressive processTwo-dimensional separable spatially auto-correlated componentrrcceR2ccis a first-order autor
56、egressive correlation matrix with an autocorrelation : 第70页/共86页第七十页,共87页。Spatial Data Analysis Two-dimensional separable spatially auto-correlated componentEnding up a very big matrix of nr*nc rows and nr*nc columns11111111121212122ccrrncccccncccnrrrrrnrrrR第71页/共86页第七十一页,共87页。Spatial Data Analysis
57、Modelling of the autoregressive process“Nugget” effect unstructured environmental correlation: ccrrnnecrIIR22第72页/共86页第七十二页,共87页。Spatial Data AnalysisModelling of the autoregressive processRAD195 trial Dothistroma infection data (0-10 scores) surface plot第73页/共86页第七十三页,共87页。Spatial Data Analysis!PAR
58、T 1Dothitr_0400 mu !r Rep Plot Genotype_id !f mv1 2 0Prow Prow IDENPpos Ppos IDEN!PART 2Dothitr_0400 mu !r Rep Plot Genotype_id !f mv1 2 0!PART 3Dothitr_0400 mu !r Rep Plot Genotype_id units !f mv1 2 0第74页/共86页第七十四页,共87页。Spatial Data Analysis第75页/共86页第七十五页,共87页。Spatial Data AnalysisModel 1 gives a v
59、ariogram that is flat which indicates that the residualshave little spatial structure 第76页/共86页第七十六页,共87页。Spatial Data AnalysisMaking the R matrix have an auto-regressive structure (model 2)gives a considerable model improvement, with the auto-correlations arelow (0.42-0.65) 第77页/共86页第七十七页,共87页。Spat
60、ial Data AnalysisAdding the independent error term (units) further improves the fit of the model (Model 3). The auto-correlations increased slightly (0.52-0.72), and the additive variance returns to its previous levels 第78页/共86页第七十八页,共87页。Spatial Data Analysis第79页/共86页第七十九页,共87页。Spatial Data Analysi
61、sHeritability was improved 18% from 0.51 to 0.60.第80页/共86页第八十页,共87页。10. Multiple Site Analysis Multiple sites usually involve heterogeneous error variances and G by E interaction. In order to incorporate the heterogeneous error variances, variance structure for R should be defined.,212121eeeuuuyyYwi
62、th y1, y2 as vectors of individual site 1 and 221A212A is additive variance in site 1 and is additive covariance between sites 1 and 2. AGAG02222121212212121212121*AAAAAAAAAAAAiRR第81页/共86页第八十一页,共87页。Multiple Site Analysis We use diallel Di_3S_5T.as as example for three sites model. 第82页/共86页第八十二页,共8
63、7页。11. Multiple Trait Analysis Multiple traits usually involve heterogeneous error variances and genetic correlation among-traits. In order to incorporate the heterogeneous error variances and genetic covariance, variance structure for error R and covariance G should be defined.,212121eeeuuuyyYfor t
64、rait t1, t2, and t3. 3211111tttyyyyanRIRRGAGiand0where G0 is genetic variance and covariance matrix among traits, and Ra = Ri is the among-traits residual variance and covariance matrix for individual i, and Ri is usually assumed same for all individuals. with y1, y2 as vectors of individual 1 and 2
65、第83页/共86页第八十三页,共87页。Multiple Trait AnalysisWe use diallel Di_3S_5T.as as example for three traits model. 第84页/共86页第八十四页,共87页。12 Example from RISF (ptaAUS )第85页/共86页第八十五页,共87页。感谢您的观看(gunkn)!第86页/共86页第八十六页,共87页。NoImage内容(nirng)总结ASReml Workshop。 Estimates (co-)variances。Tester (male) design:。7. Row-column Design。r Trait.Blk Trait.Family。S2=1。感谢您的观看(gunkn)第八十七页,共87页。
- 温馨提示:
1: 本站所有资源如无特殊说明,都需要本地电脑安装OFFICE2007和PDF阅读器。图纸软件为CAD,CAXA,PROE,UG,SolidWorks等.压缩文件请下载最新的WinRAR软件解压。
2: 本站的文档不包含任何第三方提供的附件图纸等,如果需要附件,请联系上传者。文件的所有权益归上传用户所有。
3.本站RAR压缩包中若带图纸,网页内容里面会有图纸预览,若没有图纸预览就没有图纸。
4. 未经权益所有人同意不得将文件中的内容挪作商业或盈利用途。
5. 装配图网仅提供信息存储空间,仅对用户上传内容的表现方式做保护处理,对用户上传分享的文档内容本身不做任何修改或编辑,并不能对任何下载内容负责。
6. 下载文件中如有侵权或不适当内容,请与我们联系,我们立即纠正。
7. 本站不保证下载资源的准确性、安全性和完整性, 同时也不承担用户因使用这些下载资源对自己和他人造成任何形式的伤害或损失。