供暖锅炉的中英文翻译
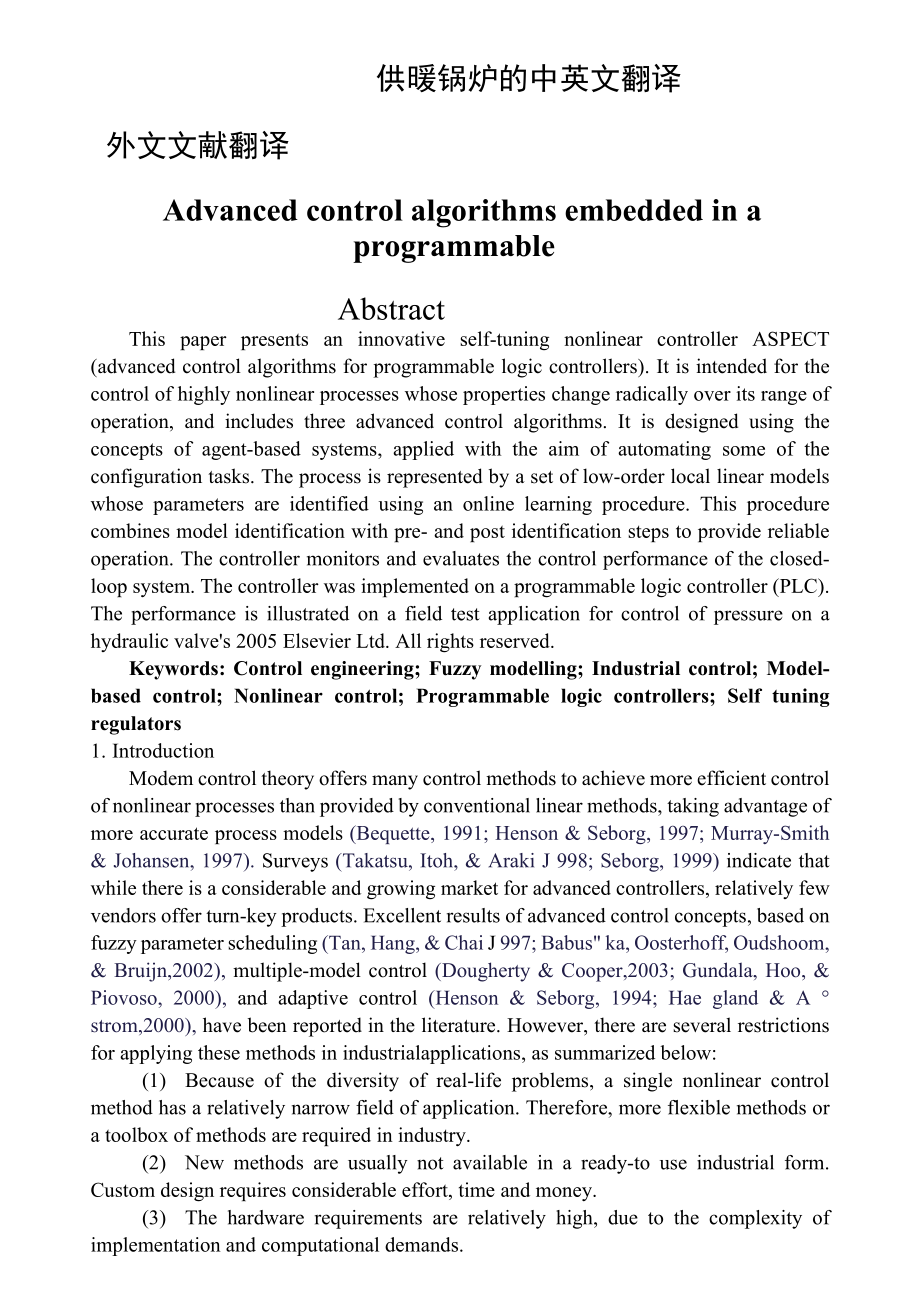


《供暖锅炉的中英文翻译》由会员分享,可在线阅读,更多相关《供暖锅炉的中英文翻译(26页珍藏版)》请在装配图网上搜索。
1、供暖锅炉的中英文翻译外文文献翻译Advanced control algorithms embedded in a programmableAbstractThis paper presents an innovative self-tuning nonlinear controller ASPECT (advanced control algorithms for programmable logic controllers). It is intended for the control of highly nonlinear processes whose properties chan
2、ge radically over its range of operation, and includes three advanced control algorithms. It is designed using the concepts of agent-based systems, applied with the aim of automating some of the configuration tasks. The process is represented by a set of low-order local linear models whose parameter
3、s are identified using an online learning procedure. This procedure combines model identification with pre- and post identification steps to provide reliable operation. The controller monitors and evaluates the control performance of the closed-loop system. The controller was implemented on a progra
4、mmable logic controller (PLC). The performance is illustrated on a field test application for control of pressure on a hydraulic valves 2005 Elsevier Ltd. All rights reserved.Keywords: Control engineering; Fuzzy modelling; Industrial control; Model-based control; Nonlinear control; Programmable logi
5、c controllers; Self tuning regulators1. IntroductionModem control theory offers many control methods to achieve more efficient control of nonlinear processes than provided by conventional linear methods, taking advantage of more accurate process models (Bequette, 1991; Henson & Seborg, 1997; Murray-
6、Smith & Johansen, 1997). Surveys (Takatsu, Itoh, & Araki J 998; Seborg, 1999) indicate that while there is a considerable and growing market for advanced controllers, relatively few vendors offer turn-key products. Excellent results of advanced control concepts, based on fuzzy parameter scheduling (
7、Tan, Hang, & Chai J 997; Babus ka, Oosterhoff, Oudshoom, & Bruijn,2002), multiple-model control (Dougherty & Cooper,2003; Gundala, Hoo, & Piovoso, 2000), and adaptive control (Henson & Seborg, 1994; Hae gland & A strom,2000), have been reported in the literature. However, there are several restricti
8、ons for applying these methods in industrialapplications, as summarized below:(1) Because of the diversity of real-life problems, a single nonlinear control method has a relatively narrow field of application. Therefore, more flexible methods or a toolbox of methods are required in industry.(2) New
9、methods are usually not available in a ready-to use industrial form. Custom design requires considerable effort, time and money.(3) The hardware requirements are relatively high, due to the complexity of implementation and computational demands.(4) The complexity of tuning (Babusv ka et al., 2002) a
10、nd maintenance makes the methods unattractive to nonspecialised engineers.(5) The reliability of nonlinear modelling is often in question.(6) Many nonlinear processes can be controlled using the well-known and industrially proven PID controller. A considerable direct performance increase (financial
11、gain) is demanded when replacing a conventional control system with an advanced one. The maintenance costs of an inadequate conventional control solution may be less obvious.The aim of this work is to design an advanced controller that addresses some of the aforementioned problems by using the conce
12、pts of agent-based systems (ABS) (Wooldridge & Jennings, 1995). The main purpose is to simplify controller configuration by partial automation of the commissioning procedure, which is typically performed by the control engineer. ABS solve difficult problems by assigning tasks to networked software a
13、gents. The software agents are characterized by properties such as autonomy (operation without direct intervention of humans), social ability (interaction with other agents), reactivity (perception and response to the environment), pro-activeness (goal-directed behaviour,taking the initiative), etc.
14、This work does not address issues of ABS theory, but rather the application of the basic concepts of ABS to the field of process systems engineering. In this context, a number of limits have to be considered. For example: initiative is restricted, a high degree of reliability and predictability is d
15、emanded, insight into the problem domain is limited to the sensor readings, specific hardware platforms are used, etc. The ASPECT controller is an efficient and user-friendly engineering tool for implementation of parameter-scheduling control in the process industry. The cumniissionuig of the contro
16、ller is simplified by autoiualic experiiiientatioii and tuning. A distinguishing feature of the controller is that the algorithms are adapted for implementation on PLC or open controller Industrial hardware platforms.The controller parameters are automatically tuned from a nonlinear process model. T
17、he model is obtained from operating process signals by experimental modelling,using a novel online learning procedure. This procedure is based on model identification using the local learning approach (Murray-Smith & Johansen J 997, p. 188). The measurement data are processed batch-wise. Additional
18、steps are performed before and after identification in order to improve the reliability of modelling, compared to adaptive methods that use recursive identification continuously (Ha, gland & A 0 strom,2(XX).The nonlinear model comprises a set of local lowered linear models, each of which is valid ov
19、er a specified operating region. The active local model(s) is selected using a configured scheduling variable. The controller is specifically designed for single-input, single output processes that may include a measured disturbance used for feed-forward. Additionally, the application range of the c
20、ontroller depends on the selected control algorithm. A modular structure of the controller permits use of a range of control algorithms that are most suitable for different processes. The controller monitors the resulting control performance andreacts to detected irregularities.The controller compri
21、ses the run-time module (RTM) and the configuration tool (CT). The RTM runs on a PLC, performing all the main functionality of real-time control, online learning and control performance monitoring. The CT, used on a personal computer (PC) during the initial configuration phase, simplifies the config
22、uration procedure by providing guidance and default parameter values. The outline of the paper is as follows: Section 2 presents an overview of the RTM structure and describes its most important modules; Section 3 gives a brief description of the CT; and finally, Section 4 describes the application
23、of the controller to a pilot plant where it is used for control of the pressure difference on a hydraulic valve in a valve test apparatus.2. Run-Time ModuleThe RTM of the ASPECT controller comprises a set of modules, linked in the form of a multi-agent system. Fig. 1 shows an overview of the RTM and
24、 its main modules: the signal pre-processing agent (SPA), the online learning agent (OLA), the model information agent (MIA), the control algorithm agent (CAA), the control performance monitor (CPM), and the operation supervisor (OS).2.1. Multi-faceted model (MFM)The ASPECT controller is based on th
25、e multi-faceted model concept proposed by Stephanopoulus, Henning, and Leone (1990) and incorporates several model forms required by the CAA and the OLA. Specifically, the MFM includes a set of local firstand second-order discrete-time linear models with time delay and offset,LOCALFig. I. Run-time n
26、uxlulc overview.OPERATIONSUPERVISOR(systemcoordinaton)which are specified by a given scheduling variable s(k).The model equation of firstorder local models isy (& + 1) =y(k)+ h u(k - du .) + c vk - Jv )+ r (1)J1/J/J.while the model equation of second order models isy(A +1) = -a y(k)-a2 y(k - l) + b
27、u(k -du )十% 卅(k - 1 - du+ J 产(& - dv) + c2 v(k - 1 - dv, + rjwhere k is the discrete time index, j is the number of the local model, y(k) is the process output signal, u(k) is the process input signal, v(k) is the optional measured disturbance signal (MD), du is the delay in the model branch from u
28、to y, dv is the delay in the model branch from v to y, and ai J, bi J, cij and ij are the parameters of the jut local model. The set of local models can be interpreted as a Takagi-Surgeon fuzzy model, which in the case of a second order model can be expressed in the following form:mmy(A +1) =00片 i/=
29、mm+ 0-d“/) + Z OJQb?7(kz2(3)mm+ Z-d%) + Z ”k -1 - t/v .)m7-IWhere bj( k) is the value of the membership function of the jut local model at the current value of Ilie scheduling vm iable s(k). Normalized Iriaugulai inenibership functions are used, as illustrated in Fig. 2.The scheduling variable s(k)
30、is calculated using coefficients kr, ky, ku, and kv, using the weighted sums(A) = krr(k) + &)(%) +-1) + kvv(k) (4)The coefficients are configured by the engineer according to the nature of the process nonlinearity.2.2. Online Learning Agent (OLA)The OLA scans the buffer of recent real-time signals,
31、prepared by the SPA, and estimates the parameters of the local models that are excited by the signals. The most recently derived parameters are submitted to the MIA only when they pass the verification test and are proved to be better than the existing set. The OLA is invoked upon demand from the OS
32、 or autonomously, when an interval of the process signals with sufficient excitation is available for processing. It processes the signals batch-wise and using the local learning approach. An advantage of the batch-wise concept is that the decision on whether to adapt the model is not performed in r
33、eal-time but following a delay that allows for inspection of the identification result before it is applied. Thus, better means for control over data selection is provided. A problem of distribution of the computation time required for identification appears with batch-wise processing of data (oppos
34、ed to the online recursive processing that is typically used in adaptive controllers).This problem is resolved using a multi-tasking operation system. Since the OLA typically requires considerably more computation than the real-time control algorithm, it runs in the background as a low-priority task
35、. The following procedure, illustrated in Fig. 3, is executed when the OLA is invoked. 2.2.1. Copy signal bufferThe buffer of the real-time signals is maintained by the SPA. When the OLA is invoked, the relevant section of the buffer is copied for further processing.2.2.2. Excitation checkA quick ex
36、citation check is performed at the start, so that processing of the signals is performed only when they contain excitation. If the standard deviations of the signals r(k), y(k), u(k), and v(k) in the active buffer are below their thresholds, the execution is cancelled.2.2.3. Copy active MFM from MIA
37、The online learning procedure always conipares the newly identified local models with the previous set of parameters. Therefore, the active MFM is copied from the MIA where it is stored. A default set of model parameters is used for the local models that have not yet been identified (see Section 2.3
38、).2.2.4. Select local modelsA local model is selected if the sum of its membership functions bj(k) over the active buffer normalized by the active buffer length exceeds a given threshold. Only the selected local models are included in further processing.2.2.5. IdentificationThe local model parameter
39、s are identified using the fuzzy instrumental variables (FIV) identification method developed by Blazv icv et aL (2003). It is an extension of the linear instrumental variables identification procedure (Ljung, 1987) for the specified MFM, based on the local learning approach (Murray-Smith & Johansen
40、, 1997). The local learning approach is based on the assumption that the parameters of all local models will not be estimated in a single regression operation. Compared to the global approach it is less prone to the problems of ill-conditioning and local minima. This method is well suited to the nee
41、ds of industrial operation (intuitiveness, gradual building of the nonlinear model, modest computational demands). It enables inventory of the local models that are not estimated properly due to insufficient excitation. It is efficient and reliable in early configuration stages, when all local model
42、s have not been estimated yet. On the other hand, the convergence in the vicinity of the optimum is slow. Therefore, it is likely to yield a worse model fit than methods employing nonlinear optimisation.The following briefly describes the procedure. Model identification is performed for each selecte
43、d local model (denotedAby the index j) separately. The initial estimated parameter vector 0is copiedAfrom the active MFM, and the covariance matrix p . WM is initialized to 105 I (identityAAmatrix). The FT S (fi177y least-squares) estimates, 0 人乂 and p are obtainedusing weighted least-squares identi
44、fication, with bj(k) used for weighting. The calculation is performed recursively to avoid matrix inversion. The FIV (fuzzyAAinstrumental variables) estimates, 0 ia and p are calculated using weightedinstrumental variables identification. In order to prevent result degradation by noise, adead zone i
45、s used in each step of FIV and FLS recursivehig. 3. Oriinc learning procedure.Estimation. The vector of parameters and the covariance matrix are updated only if the absolute weighted difference between the process output and its prediction is above the configured noise threshold.In case of lack of e
46、xcitation in the branch from u to y or in the model branch from v to y (or when measured disturbance is not present at all), variants of the method with reduced parameter estimate vectors are used.2.2.6. Verification/validationThis step is performed by comparing the simulation output of a selected l
47、ocal model with the actual process output in the proximity of the local model position. The normalized sum of mean square errors (MSEj) is calculated. The proximity is defined by the membership functions bj. For each of the selected local models, this step is carried out with three sets of model par
48、ameters: 0 A 0 and。 the set with the lowest MSEj is selected. Then, global verification is performed by comparing the simulation output of the fuzzy model including the selected set with the actual process output. The normalized sum of mean square errors (MSEG) is calculated. If the global verificat
49、ion result is improved compared to the initial fuzzy model, the selected set isA sent to the MIA as the result of online learning, otherwise the original set W remains in use. For each processed local model, the MIA receives the MSEj, which serves as a confidence index, and a flag indicating whether
50、 the model is new or not. 2.2.7. Model structure estimationTwo model structure estimation units are also included in the OLA. The dead-time unit (DTU) estimates the process time delay. The membership function unit (MFU) suggests whether a new local model should be inserted. It estimates an additiona
51、l local model in the middle of the interval between the two neighboring local models that are the most excited. The model is submitted to the MIA if the global validation of the resulting fuzzy model is sufficiently improved, compared to the original fuzzy model.2.3. Model Information Agent (MIA)The
52、 task of the MIA is to maintain the active MFM and its status information. Its primary activity is processing the online learning results. When a new local model is received from the OLA, it is accepted if it passes the stability test and its confidence index is sufficient. If it is accepted, a read
53、y fbr tuning“ flag is set fbr the CAA. Another flag indicates whether the local model has been tuned since start-up or not. If the model confidence index is very low, the automatic mode may be disabled. The MIA contains a mechanism for inserting additional local models into the MFM. This may occur e
54、ither by request or auloinaliually, using ihe MFU of the OLA. The MIA may also store the active MFM to a local database or recall a previously stored one, which is useful for changing of modes. At initial configuration, the MIA is filled with default local models based on the initial estimation of t
55、he process dynamics. They are not exact but may provide reliable (although sluggish) control performance, similar to the Safe mode. Using online learning through experiments or normal operating records (when the conditions are appropriate for closed-loop identification),an accurate model of the plan
56、t is estimated gradually by receiving identified local models from the OLA.2.4. Control Algorithm Agent (CAA)The CAA comprises an industrial nonlinear control algorithm and a procedure for automatic tuning of its parameters from the MFM. Several different CAAs may be used in the controller and may b
57、e interchanged in the initial configuration phase. The following modes of operation are supported:_ Manual mode: open-loop operation (actuator constraints are enforced). _ Safe mode: a fixed PI controller with conservatively tuned parameters._ Auto mode (or several auto modes with different tuning p
58、arameters): a nonlinear controller. The CAAs share a common interface of interaction with the OS and acommon modular internal structure, consisting of three layers:1. The control layer offers the functionality of a local linear controller (or several local linear controllers simultaneously), includi
59、ng everything required for industrial control, such as handling of constraints with anti-windup protection, bump-less mode switching, etc.2. The scheduling layer performs real-time switching orScheduling (blending) of tuned local linear controllers, so that in conjunction with the control layer a fi
60、xed-parameter nonlinear controller is realized.3. The tuning layer executes the automatic tuning procedure of the controller parameters from the MFM when the MIA reports that a new local model is generated if auto-tuning is enabled. The parameters of the control layer and the scheduling layer are re
61、placed in such a manner that real-time control is not disturbed. Three CAAs have been developed and each has been proved effective in specific applications: the Fuzzy parameter-scheduling controller (FPSC), the dead-time compensation controller (DTCC), and the rule-based neural controller (RBNC). In
62、 this paper, only the concept of the FPSC is described briefly in the following subsection.4. 4.1. Fuzzy parameter-scheduling controllerAn overview of the FPSC is shown in Fig. 4.(At controlef tuning. foBowvng a moeel change or a change of auUMsng parameters)(In escn samping irtervatFig. 4. FPSC Qve
63、rvKw.The control layer of the FPSC includes a single PID controller in the form suitable for controller blending using vclocity-bascd linearization. It is equipped with anti-windup protection and bump-less transfer. The scheduling layer of the FPSC performs fuzzy blending of the controller parameter
64、s (in the case of Ti, its inversevalue) according to the scheduling variable s(k) and the membership functions bj(k) of the local models. The instrument of velocity-based linearization enables the dynamics of the blended global controller to be a linear combination of the local controller dynamics i
65、n the entire operating region, not just around equilibrium operating points. This provides the potential to improve performance with few local models and more transparent behaviors in off-equilibrium operating points (Leith & Leithead, 1998; Kocijan, Zv unicv ,Strmcv nik, & Vrancv c, 2002).The tuning layer of the FPSC is based on the magnitude optimum (MO) criterion implemented using the multiple integration (MI) method (Vrancv ic ,Stnncv nik, & Juricv icz, 2001). The MO criterion makes the magnitude (amplitude) of the system closed-loop set point r
- 温馨提示:
1: 本站所有资源如无特殊说明,都需要本地电脑安装OFFICE2007和PDF阅读器。图纸软件为CAD,CAXA,PROE,UG,SolidWorks等.压缩文件请下载最新的WinRAR软件解压。
2: 本站的文档不包含任何第三方提供的附件图纸等,如果需要附件,请联系上传者。文件的所有权益归上传用户所有。
3.本站RAR压缩包中若带图纸,网页内容里面会有图纸预览,若没有图纸预览就没有图纸。
4. 未经权益所有人同意不得将文件中的内容挪作商业或盈利用途。
5. 装配图网仅提供信息存储空间,仅对用户上传内容的表现方式做保护处理,对用户上传分享的文档内容本身不做任何修改或编辑,并不能对任何下载内容负责。
6. 下载文件中如有侵权或不适当内容,请与我们联系,我们立即纠正。
7. 本站不保证下载资源的准确性、安全性和完整性, 同时也不承担用户因使用这些下载资源对自己和他人造成任何形式的伤害或损失。