毕业设计论文 外文文献翻译 中英文对照 快速和简单 的沟通使用Co – SVM对图像检索进行主动学习
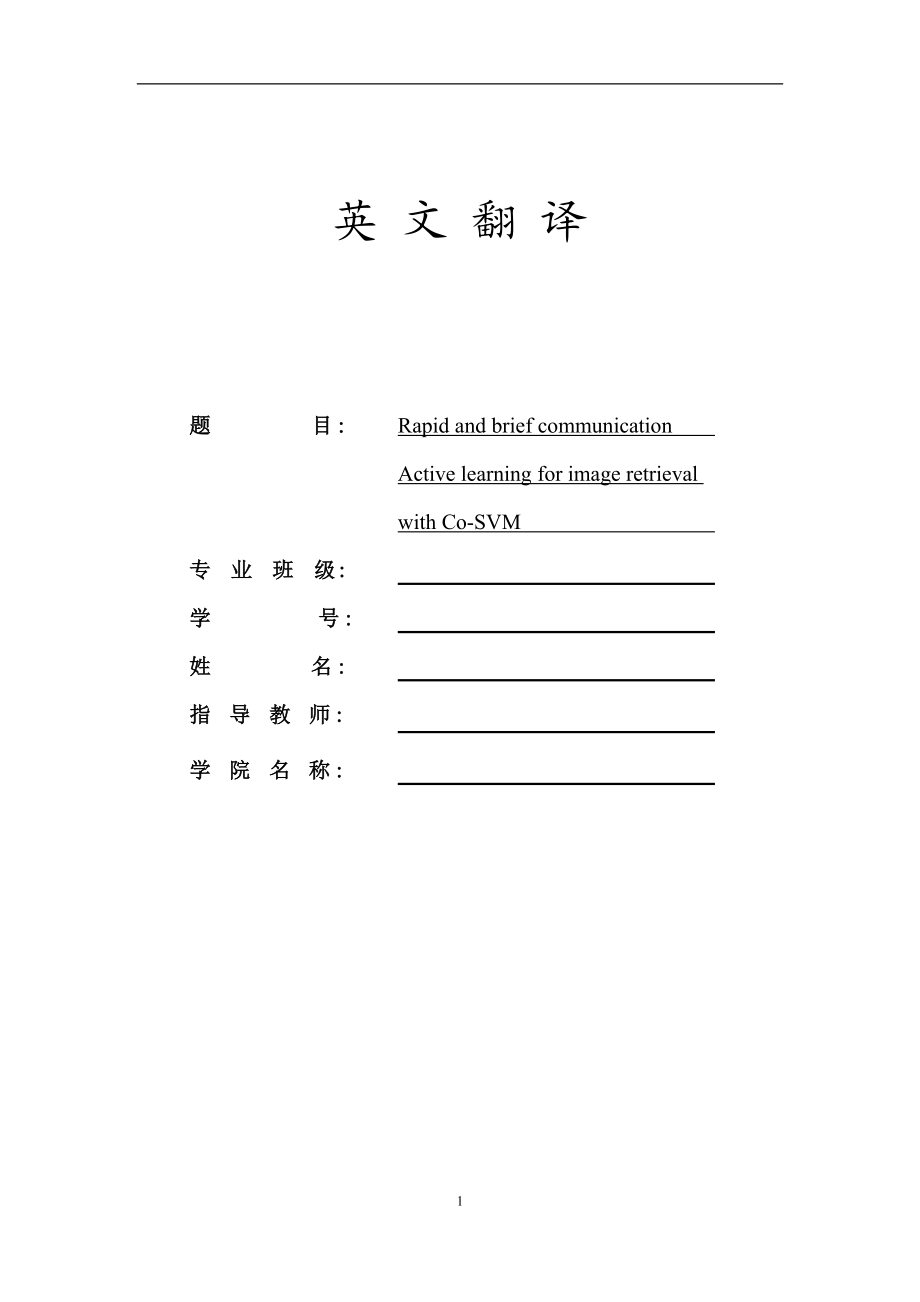


《毕业设计论文 外文文献翻译 中英文对照 快速和简单 的沟通使用Co – SVM对图像检索进行主动学习》由会员分享,可在线阅读,更多相关《毕业设计论文 外文文献翻译 中英文对照 快速和简单 的沟通使用Co – SVM对图像检索进行主动学习(14页珍藏版)》请在装配图网上搜索。
1、英 文 翻 译题 目:Rapid and brief communication Active learning for image retrieval with Co-SVM 专 业 班 级: 学 号: 姓 名: 指 导 教 师: 学 院 名 称: 13快速和简单的沟通使用Co SVM对图像检索进行主动学习摘 要在相关反馈算法中,选择性抽样通常用来减少标签成本以及探讨未标记的数据。在本文中,为了提高图像检索选择性抽样的表现,我们提出了一个积极的学习算法,这个算法被称为Co SVM。在Co SVM算法中,色彩和纹理很自然的当做一幅图像足够的、不相关的试图。我们能够分别在颜色和纹理的特征子空间上
2、学习SVM分类器。因此,这两个分类器被用于分类未标记的数据。当两个分类器分辨出不同时,这些未标记的数据就成为了选择标签。实验结果表明我们提出的这种算法对图像检索是非常有利的。1 前 言相关反馈是提高图像检索性能的一种重要的方法1。对于企业大型图像数据库检索的问题,标记图像总是比未标记的图像罕见。当只提供一个小的标记图像时,如何利用大量未标记的图像去增强学习算法的性能已成为一个热门话题。Tong 和Chang提出了一种主动学习的算法,这种算法叫做SVMAct ive 2。他们认为处于边界的样本是最丰富的。因此在每一轮相关反馈中,能作为标签返回给用户的是那些最接近支持矢量边界的图像。通常情况下,图
3、像的特征表示是一个组合的,多样化的功能,如颜色,纹理,形状等。例如,对于指定的图像,不同特征的重要性是显著不同的。另一方面,重要性相同的特征对于不同图像也是不同的。例如,通常情况下,颜色是比形状更为突出的图像。然而,检索结果是所有特征的平均作用,而忽略了个别特征鲜明的特性。一些研究显示多视角的学习比单视图的假说要好3,4。在本文中,我们把颜色和纹理作为图像描述的两个充分的、不相关的图像特征。受SVMAct ive的启发,我们提出了一种新的主动学习法,这种方法叫做CoSVM。首先,在不同的特征表示上分别学习SVM分类器,然后这些分类器用来从未标记的数据中选择最翔实的合作样本,最后,这些翔实样本将
4、作为标签返回给用户。2 支持向量机作为一个有效的二元分类器,将SVM用于图像检索相关反馈的分类是特别适合的5。随着标签图像,SVM学习一个边界(即超平面),就是从带有最大利润的不相关的图片中分离相关图像。处于边界一侧的图像被认为是相关的,而处于另一侧的则被认为是不相关的。给定一个标记图像集(x1,y1), . . . ,(xn,yn) , xi 是一幅图像的特征描述,yi 1,+1 是类标签(- 1表示正极,+1表示负极)。训练SVM分类器会导致下面的二次优化问题:S.t:其中C是一个常数,k为内核的功能。边界(超平面)是其中满足任何支持向量的条件是:该分类函数可以写为:3 合作支持向量机3.
5、1. 双试图计划假设图像的颜色特征和纹理特征是两个互不相关的观点是自然的也是合理的。假设x=c1, . . . ,ci , t1 , . . . ,tj 是一幅图像的特征表示,其中 c1, . . . ,ci和 t1, . . . ,tj 分别是颜色属性和纹理属性。为简单起见,我们定义特征表示空间V = VC VT , 而c1, . . . ,ciVC , t1, . . . ,tj VT。为了尽可能找到相关的图像,像一般相关反馈的方法,在第一阶段的联合视图V中支持向量机用于学习标记的样本分类h。通过h未标记集被分为正面和负面的.然后m的正面形象将返回到用户的标签。在第二个阶段,通过VC颜色视
6、图和VT纹理视图SVM用于在标记样本上分别学习hc和ht两种分类器 。对于两个分类有分歧的未标记的样本推荐给用户做标签,并将其命名为争夺样本。也就是说,争论样本以HC(CP)的分类划分为阳性,以HT(TN)的分类划分为阴性。或以HC(CN)的分类划分为阴性,以HT(TP)的分类划分为阳性。对于每一个分类器,样品之间的超平面(边界)的距离可以被看作信心程度。越大的距离,越高的信任度。为了确保用户可以标签最翔实的样本,在两种意见上接近超平面的样本被推荐给用户作为标签。3.2. 多视图计划在两个个案中,提出的算法很容易扩展到多视图计划。假设,一个是彩色图像特征被表示为V = V2的 V1的 Vk,钾
7、 2条所界定,每个VI,i= 1,钾对应的彩色图有不同的看法。然后在每一个视图上可以学习K向量机分类。所有未标记的数据被 k 支持向量机分类器归类为阳性 (+ 1) 或阴性 (1)。定义置信度D(x) _ki=1sign(hi(x) _。置信度可以反映上一示例中指定的所有分类器的一致性。置信度越高,分类器越一致。相反,低置信度说明分类器是不确定的。这些不确定的样本标签将导致性能的最大改进。因此,其信任度是最低的未标记的样本被视为争夺样本。3.3. SVM 简介SVM( Support Vector machine, 支持向量机) 方法4是建立在统计学习理论的VC 维理论和结构风险最小原理基础上
8、的,根据有限的样本信息在模型的复杂性和学习能力之间寻求最佳的折衷, 以期获得最好的推广能力。SVM的主要思想是建立一个超平面作为决策曲面, 使得正例和反例之间的隔离边缘被最大化。对于二维线性可分情况, 令H 为把两类训练样本没有错误地分开的分类线, H1, H2分别为过各类中离分类线最近的样本且平行于分类线的直线, 它们之间的距离叫做分类间隔。所谓最优分类线就是要求分类线不但能将两类正确分开, 而且使分类间隔最大。在高维空间, 最优分类线就成为最优分类面。实验:为了验证了在性能上改进算法的有效性,我们将它与Tong & Chang SVMAct ive及传统的相关反馈算法支持向量机进行比较。C
9、orel 图像光盘从所选子集中执行实验。在我们的子集中有 50 个类别。每个类别包含100个图像,一共有5000个图像。该类别有不同的语义,如动物,建筑,景观等的含义。我们的实验的主要目的是验证联合支持向量机的学习机制是否有用,因此,我们只用来简单的颜色和纹理特征来表示图RGB 颜色特征包括 125 维颜色直方图矢量和 6 维颜色矩矢量。像。纹理特征提取使用 3 级离散小波变换 (DWT)。均值和方差平均每10个子带被排列成20维纹理特征矢量。在支持向量机分类器中采用径向基核。该内核宽度是由交叉验证的方法得到的。每个类别500个图像的前10 个形象被选择作为查询图像来探测检索性能。在每一轮中,
10、只有前10名的图像标记和10个最不自信的图片集争夺选定的标签。以下文本中的所有精度都为平均测试的所有图像的准确性。第三轮及第五轮相关反馈后2和3是描述3种算法的相关反馈后准确性的范围曲线。从比较的结果中,我们可以看到拟议的算法 (联合-支持向量机) 胜于 SVMAct (活动支持向量机) 和传统的相关反馈方法 (支持向量机)。此外,我们在调查前100名中前10名各种算法的准确性并有五轮的反馈。由于空间有限我们只分别在图片1和图片2中表示了前 30 和前 50的结果。图一 前30名的平均图像检索 图二前30名的平均图像检索5 相关作品:co-training 3 和 co-testing 4是两
11、种典型的多视点学习算法 。co-training 算法采用合作学习策略,要求这两种视图的数据是兼容和冗余的。我们曾尝试结合 co-training增加颜色和纹理分类器的性能,但结果却更糟。考虑到 co-training 的状况,人们会很自然的发现颜色属性和纹理属性是一幅彩色图像不兼容的并且不相关的属性。相比之下,co-testing 的要求图像应该是兼容并且相关的,使分类器能更独立的分类。Tong和Chang首先推出的 SVMAct 2 的是主动学习关于图像检索相关反馈的方法。他们认为在处于边界的示例可以尽快减少版本空间,即消除假说。因此,每次的相关反馈,最接近该超平面的图像会作为标记返回给用
12、户。SVMActive 是在单一视图的情况下对版本空间最小化最佳的。建议的算法可以被认为是 SVMActive 在多个视图的情况下的扩展。6 总结在这篇文章中,我们建议积极学习相关反馈中的选择性的抽样算法联合支持向量机。为了提高性能,相关反馈分为两个阶段。第一阶段我们通过未标记的图像的相似性查询排名,并让用户可以像常见的相关反馈算法那样标签顶部图像。在第二阶段,为了减少标签规定,只有一组内容最丰富的示例被联合-支持向量机所选择作为标签。实验结果显示联合-支持向量机与SVMActive和没有主动学习的传统相关反馈算法相比,有明显的改善。鸣谢,第一作者被授予诺基亚博士后奖学金。参考资料1 Y. R
13、ui, T.S. Huang, S.F. Chang, Image retrieval: current techniques,promising directions and open issues, J. Visual Commun. ImageRepresentation 10 (1999) 3962.2 S. Tong, E. Chang, Support vector machine active learning for imageretrieval, in: Proceedings of the Ninth ACM International Conferenceon Multi
14、media, 2001, pp. 107118.3 A. Blum, T. Mitchell, Combining labeled and unlabeled data withco-training, in: Proceedings of the 11th Annual Conference onComputational Learning Theory, 1998, pp. 92100.4 I. Muslea, S. Minton, C.A. Knoblock, Selective sampling withredundant views, in: Proceedings of the 1
15、7th National Conference onArtificial Intelligence, 2000, pp. 621626.5 V. Vapnik, Statistical Learning Theory, Wiley, New York, 1998.Rapid and brief communicationActive learning for image retrieval with Co-SVMAbstractIn relevance feedback algorithms, selective sampling is often used to reduce the cos
16、t of labeling and explore the unlabeled data. In this paper, we proposed an active learning algorithm, Co-SVM, to improve the performance of selective sampling in image retrieval. In Co-SVM algorithm, color and texture are naturally considered as sufficient and uncorrelated views of an image. SVM cl
17、assifiers are learned in color and texture feature subspaces, respectively. Then the two classifiers are used to classify the unlabeled data. These unlabeled samples which are differently classified by the two classifiers are chose to label. The experimental results show that the proposed algorithm
18、is beneficial to image retrieval.1. IntroductionRelevance feedback is an important approach to improve the performance of image retrieval systems 1. For largescale image database retrieval problem, labeled images are always rare compared with unlabeled images. It has become a hot topic how to utiliz
19、e the large amounts of unlabeled images to augment the performance of the learning algorithms when only a small set of labeled images is available. Tong and Chang proposed an active learning paradigm, named SVMAct ive 2. They think that the samples lying beside the boundary are the most informative.
20、 Therefore, in each round of relevance feedback, the images that are closest to the support vector boundary are returned to users for labeling. Usually, the feature representation of an image is a combination of diverse features, such as color, texture, shape, etc. For a specified example, the contr
21、ibution of different features is significantly different. On the other hand, the importanceof the same feature is also different for differentsamples. For example, color is often more prominent than shape for a landscape image. However, the retrieval results are the averaging effort of all features,
22、 which ignores the distinct properties of individual feature. Some works have suggested that multi-view learning can do much better thanthe single-view learning in eliminating the hypotheses consistent with the training set 3,4.In this paper, we consider color and texture as two sufficient and uncor
23、related feature representations of an image. Inspired by SVMAct ive, we proposed a novel active learning method, Co-SVM. Firstly, SVM classifiers are separately learnt in different feature representations and then these classifiers are used to cooperatively select the most informative samples from t
24、he unlabeled data. Finally, the informativesamples are returned to users to ask for labeling.2. Support vector machinesBeing an effective binary classifier, Support Vector Machines (SVM) is particularly fit for the classification task in relevance feedback of image retrieval 5. With the labeled imag
25、es, SVM learns a boundary (i.e., hyper plane) separating the relevant images from the irrelevant images with maximum margin. The images on a side of boundary areconsidered as relevance, and on the other side are looked as irrelevance.Given a set of labeled images (x1, y1), . . . , (xn, yn), xi is th
26、e feature representation of one image, yi 1,+1 is the class label (1 denotes negative and +1 denotes positive). Training SVM classifier leads to the following quadratic optimization problem:S.t:where C is a constant and k is the kernel function. The boundary (hyper plane) isWhere are any support vec
27、tors satisfied: The classification function can be written as 3. Co-SVM3.1. Two-view schemeIt is natural and reasonable to assume that color features and texture features are two sufficient and uncorrelated views of an image. Assume that x = c1, . . . , ci, t1, . . . , tj is the feature representati
28、on of an image, where c1, . . . , ci and t1, . . . , tj are color attributes and texture attributes, respectively. For simplicity, we define the feature representation space V = VC VT , and c1, . . . , ci VC, t1, . . . , tj VT .In order to find relevant images as much as possible, like the general r
29、elevance feedback methods, SVM is used to learn a classifier h on these labeled samples with the combined view V at the first stage. The unlabeled set is classified into positive and negative by h. Then m positive images are returned to user to label. At the second stage, SVM is used to separately l
30、earn two classifiers hC and hT on the labeled samples only with color view VC and texture view VT , respectively. A set of unlabeled samples that disagree between the two classifiers is recommended to user to label, which named contention samples. That is, the contention samples are classified as po
31、sitive by hC (CP) while are classified as negative by hT (TN), or are classified as negative by hC (CN) while are classified as positiveby hT (TP). For each classifier, the distance between sample and the hyper plane (boundary) can be looked as the confidence degree. The larger the distance, the hig
32、her the confidence degree is. In order to ensure that users can label the most informative samples, the samples which are close to hyper plane in both views are recommended to user to label. 3.2. Multi-view schemeThe proposed algorithm in two-view case is easily extended to multi-view scheme. Assume
33、 that the feature representation of a color image is defined as V = V1 V2 Vk, k2, each Vi, i = 1, . . . , k corresponds to a different view of the color image. Then k SVM classifiers hi can be individually learnt on each view. All unlabeled data are classified as positive (+1) or negative (1) by k S
34、VM classifiers, respectively. Define the confidence degreeD(x) =_ki=1sign(hi(x)_.The confidence degree can reflect the consistency of all classifiers on a specified example. The higher the confidence degree, the more consistent the classification is. Inversely, lower degree indicates that the classi
35、fication is uncertain. The labeling on these uncertain samples will result in maximum improvement of performance. Therefore, the unlabeled samples whose confidence degrees are the lowest are considered as the contention samples.3.3. About SVM SVM (Support Vector machine, support vector machine) meth
36、od 4 is based on statistical learning theory and the theory of VC dimension based on structural risk minimization principle, according to the limited sample information in the model complexity and learning ability of the most sought between good compromise to obtain the best generalization ability.
37、The main idea of SVM is a hyperplane as the decision surface, making the positive examples and counterexamples of separation between the edges is maximized. For the two-dimensional linear separable case, so H to the two types of training samples is not wrong to separate classification line, H1, H2,
38、respectively from the classification of various types in the sample line and the recent classification of lines parallel to the line, they shall called the interval distance between categories. The so-called optimal separating line is to ask the correct classification of line not only be able to sep
39、arate the two, but also the largest classification interval. In high dimensional space, the optimal classification line has become the optimal classification surface.4. ExperimentsTo validate the effectiveness of the proposed algorithm in improvement of performance, we compare it with Tong & Changs
40、SVMAct ive and the traditional relevance feedback algorithm using SVM. Experiments are performed on a subset selected from the Corel image CDs. There are 50 categories in our subset. Each category contains 100 images, 5000 images in all. The categories have different semantic meanings, such as anima
41、l, building, landscape, etc. In our experiments, the main purpose is to verify if the learning mechanisms of Co-SVM are useful, so we only employed simple color and texture features to represent images. The color features include 125-dimensional color histogram vector and 6-dimensional color moment
42、vector in RGB. The texture features are extracted using 3-level discrete wavelet transformation (DWT). The mean and variance averaging on each of 10 subbands are arranged to a 20-dimensional texture feature vector. RBF kernel is adopted in SVM classifiers. The kernel width is learnt by cross-validat
43、ion approach.The first 10 images of each category, 500 images in total,are selected as query images to probe the retrieval performance. In each round, only the top 10 images are labeled and 10 least confident images selected from contention set are labeled. All accuracy in the following text is the
44、averaging accuracy of all test images. Figs. 2 and 3 are the accuracy vs. scope curve of the three algorithms after the third and fifth rounds of relevance feedback, respectively. From the comparison results we can see that the proposed algorithm (Co-SVM) is better than SVMAct ive (active SVM) and t
45、he traditional relevance feedback method (SVM). Furthermore, we investigate the accuracy of various algorithms within top 10 to top 100, and with five rounds of feedback. For limited space, we only picture the results of top 30 and top 50 in Figs.1and 5, respectively. The detailed results are summar
46、ized in Table 1. The results depicted in Table 1 show thatCo-SVM achieves the highest performance.5. Related worksCo-training 3 and co-testing 4 are two representative multi-view learning algorithms. Co-training algorithm adopts cooperative learning strategy and requires that the two views of data a
47、re compatible and redundant. We have attempted to augment the performance of both color and texture classifiers by combining co-training, but the results were worse. Considering the condition of co-training, it is not surprising to find that color attribute and texture attribute are not compatible b
48、ut uncorrelated for a color image. In contrast, co-testing requires that the views should be sufficient and uncorrelated which makes the classifiers more independent for classification.Tong and Chang firstly introduced active learning approach to relevance feedback of image retrieval, SVMAct ive 2.
49、They think that the samples lying beside the boundary can reduce the version space as fast as possible, i.e. eliminating the hypotheses. Therefore, in each round of relevance feedback, the images that are closest to the hyperplane are returned to users for labeling. SVMAct ive is optimal for minimiz
50、ing the version space in case of single view. The proposed algorithm can be regarded as an extension of SVMAct ive in multiple view case.6. ConclusionsIn this paper, we proposed a novel active learning algorithm for selective sampling in relevance feedback, Co-SVM. In order to improve the performanc
51、e, the relevancefeedback is divided into two stages. At the first stage, we rank the unlabeled images by their similarity to the query and let users to label the top images like the common relevance feedback algorithms. In order to reduce the labeling requirement, only a set of the most informative
52、samples are selected by Co-SVM to label at the second stage. The experimental results show that the Co-SVM achieves obvious improvement compared with SVMAct ive and the traditional relevance feedback algorithm without active learning.AcknowledgementsThe first author was supported under Nokia Postdoc
53、toral Fellowship.References1 Y. Rui, T.S. Huang, S.F. Chang, Image retrieval: current techniques,promising directions and open issues, J. Visual Commun. ImageRepresentation 10 (1999) 3962.2 S. Tong, E. Chang, Support vector machine active learning for imageretrieval, in: Proceedings of the Ninth ACM
54、 International Conferenceon Multimedia, 2001, pp. 107118.3 A. Blum, T. Mitchell, Combining labeled and unlabeled data withco-training, in: Proceedings of the 11th Annual Conference onComputational Learning Theory, 1998, pp. 92100.4 I. Muslea, S. Minton, C.A. Knoblock, Selective sampling withredundant views, in: Proceedings of the 17th National Conference onArtificial Intelligence, 2000, pp. 621626.5 V. Vapnik, Statistical Learning Theory, Wiley, New York, 1998.
- 温馨提示:
1: 本站所有资源如无特殊说明,都需要本地电脑安装OFFICE2007和PDF阅读器。图纸软件为CAD,CAXA,PROE,UG,SolidWorks等.压缩文件请下载最新的WinRAR软件解压。
2: 本站的文档不包含任何第三方提供的附件图纸等,如果需要附件,请联系上传者。文件的所有权益归上传用户所有。
3.本站RAR压缩包中若带图纸,网页内容里面会有图纸预览,若没有图纸预览就没有图纸。
4. 未经权益所有人同意不得将文件中的内容挪作商业或盈利用途。
5. 装配图网仅提供信息存储空间,仅对用户上传内容的表现方式做保护处理,对用户上传分享的文档内容本身不做任何修改或编辑,并不能对任何下载内容负责。
6. 下载文件中如有侵权或不适当内容,请与我们联系,我们立即纠正。
7. 本站不保证下载资源的准确性、安全性和完整性, 同时也不承担用户因使用这些下载资源对自己和他人造成任何形式的伤害或损失。