LECTURE2INTELLIGENTAGENTS
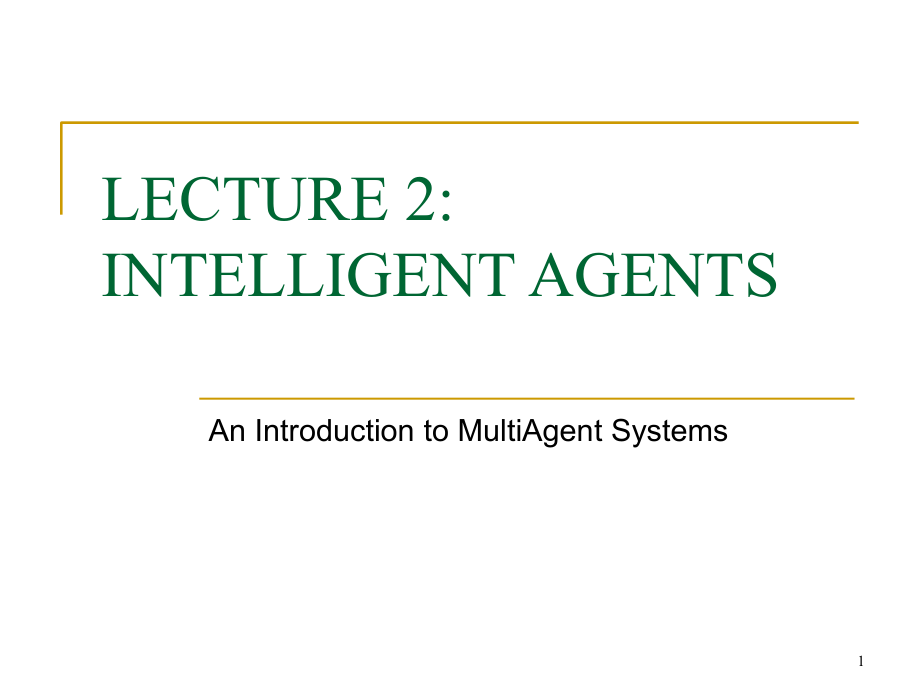


《LECTURE2INTELLIGENTAGENTS》由会员分享,可在线阅读,更多相关《LECTURE2INTELLIGENTAGENTS(60页珍藏版)》请在装配图网上搜索。
1、LECTURE 2:INTELLIGENT AGENTSAn Introduction to MultiAgent Systems1What is an Agent?nThe main point about agents is they are autonomous:capable of acting independently,exhibiting control over their internal statenThus:an agent is a computer system capable of autonomous action in some environment in o
2、rder to meet its design objectivesSYSTEMENVIRONMENTinputoutput2What is an Agent?nTrivial(non-interesting)agents:qthermostatqUNIX daemon(e.g.,biff)nAn intelligent agent is a computer system capable of flexible autonomous action in some environmentnBy flexible,we mean:qreactiveqpro-activeqsocial3React
3、ivitynIf a programs environment is guaranteed to be fixed,the program need never worry about its own success or failure program just executes blindlyqExample of fixed environment:compilernThe real world is not like that:things change,information is incomplete.Many(most?)interesting environments are
4、dynamicnSoftware is hard to build for dynamic domains:program must take into account possibility of failure ask itself whether it is worth executing!nA reactive system is one that maintains an ongoing interaction with its environment,and responds to changes that occur in it(in time for the response
5、to be useful)4ProactivenessnReacting to an environment is easy(e.g.,stimulus response rules)nBut we generally want agents to do things for usnHence goal directed behaviornPro-activeness=generating and attempting to achieve goals;not driven solely by events;taking the initiativenRecognizing opportuni
6、ties5Balancing Reactive and Goal-Oriented BehaviornWe want our agents to be reactive,responding to changing conditions in an appropriate(timely)fashionnWe want our agents to systematically work towards long-term goalsnThese two considerations can be at odds with one anothernDesigning an agent that c
7、an balance the two remains an open research problem6Social AbilitynThe real world is a multi-agent environment:we cannot go around attempting to achieve goals without taking others into accountnSome goals can only be achieved with the cooperation of othersnSimilarly for many computer environments:wi
8、tness the InternetnSocial ability in agents is the ability to interact with other agents(and possibly humans)via some kind of agent-communication language,and perhaps cooperate with others7Other PropertiesnOther properties,sometimes discussed in the context of agency:nmobility:the ability of an agen
9、t to move around an electronic networknveracity:an agent will not knowingly communicate false informationnbenevolence:agents do not have conflicting goals,and that every agent will therefore always try to do what is asked of itnrationality:agent will act in order to achieve its goals,and will not ac
10、t in such a way as to prevent its goals being achieved at least insofar as its beliefs permitnlearning/adaption:agents improve performance over time8Agents and ObjectsnAre agents just objects by another name?nObject:qencapsulates some stateqcommunicates via message passingqhas methods,corresponding
11、to operations that may be performed on this state9Agents and ObjectsnMain differences:qagents are autonomous:agents embody stronger notion of autonomy than objects,and in particular,they decide for themselves whether or not to perform an action on request from another agentqagents are smart:capable
12、of flexible(reactive,pro-active,social)behavior,and the standard object model has nothing to say about such types of behaviorqagents are active:a multi-agent system is inherently multi-threaded,in that each agent is assumed to have at least one thread of active control10Objects do it for freenagents
13、 do it because they want tonagents do it for money11Agents and Expert SystemsnArent agents just expert systems by another name?nExpert systems typically disembodied expertise about some(abstract)domain of discourse(e.g.,blood diseases)nExample:MYCIN knows about blood diseases in humansqIt has a weal
14、th of knowledge about blood diseases,in the form of rulesqA doctor can obtain expert advice about blood diseases by giving MYCIN facts,answering questions,and posing queries12Agents and Expert SystemsnMain differences:qagents situated in an environment:MYCIN is not aware of the world only informatio
15、n obtained is by asking the user questionsqagents act:MYCIN does not operate on patientsnSome real-time(typically process control)expert systems are agents13Intelligent Agents and AInArent agents just the AI project?Isnt building an agent what AI is all about?nAI aims to build systems that can(ultim
16、ately)understand natural language,recognize and understand scenes,use common sense,think creatively,etc.all of which are very hardnSo,dont we need to solve all of AI to build an agent?14Intelligent Agents and AInWhen building an agent,we simply want a system that can choose the right action to perfo
17、rm,typically in a limited domainnWe do not have to solve all the problems of AI to build a useful agent:a little intelligence goes a long way!nOren Etzioni,speaking about the commercial experience of NETBOT,Inc:“We made our agents dumber and dumber and dumberuntil finally they made money.”15Environm
18、ents Accessible vs.inaccessiblenAn accessible environment is one in which the agent can obtain complete,accurate,up-to-date information about the environments statenMost moderately complex environments(including,for example,the everyday physical world and the Internet)are inaccessiblenThe more acces
19、sible an environment is,the simpler it is to build agents to operate in it16Environments Deterministic vs.non-deterministicnA deterministic environment is one in which any action has a single guaranteed effect there is no uncertainty about the state that will result from performing an actionnThe phy
20、sical world can to all intents and purposes be regarded as non-deterministicnNon-deterministic environments present greater problems for the agent designer17Environments-Episodic vs.non-episodicnIn an episodic environment,the performance of an agent is dependent on a number of discrete episodes,with
21、 no link between the performance of an agent in different scenariosnEpisodic environments are simpler from the agent developers perspective because the agent can decide what action to perform based only on the current episode it need not reason about the interactions between this and future episodes
22、18Environments-Static vs.dynamicnA static environment is one that can be assumed to remain unchanged except by the performance of actions by the agentnA dynamic environment is one that has other processes operating on it,and which hence changes in ways beyond the agents controlnOther processes can i
23、nterfere with the agents actions(as in concurrent systems theory)nThe physical world is a highly dynamic environment19Environments Discrete vs.continuousnAn environment is discrete if there are a fixed,finite number of actions and percepts in itnRussell and Norvig give a chess game as an example of
24、a discrete environment,and taxi driving as an example of a continuous onenContinuous environments have a certain level of mismatch with computer systemsnDiscrete environments could in principle be handled by a kind of“lookup table”20Agents as Intentional SystemsnWhen explaining human activity,it is
25、often useful to make statements such as the following:Janine took her umbrella because she believed it was going to rain.Michael worked hard because he wanted to possess a PhD.nThese statements make use of a folk psychology,by which human behavior is predicted and explained through the attribution o
26、f attitudes,such as believing and wanting(as in the above examples),hoping,fearing,and so onnThe attitudes employed in such folk psychological descriptions are called the intentional notions21Agents as Intentional SystemsnThe philosopher Daniel Dennett coined the term intentional system to describe
27、entities whose behavior can be predicted by the method of attributing belief,desires and rational acumennDennett identifies different grades of intentional system:A first-order intentional system has beliefs and desires(etc.)but no beliefs and desires about beliefs and desires.A second-order intenti
28、onal system is more sophisticated;it has beliefs and desires(and no doubt other intentional states)about beliefs and desires(and other intentional states)both those of others and its own22Agents as Intentional SystemsnIs it legitimate or useful to attribute beliefs,desires,and so on,to computer syst
29、ems?23Agents as Intentional SystemsnMcCarthy argued that there are occasions when the intentional stance is appropriate:To ascribe beliefs,free will,intentions,consciousness,abilities,or wants to a machine is legitimate when such an ascription expresses the same information about the machine that it
30、 expresses about a person.It is useful when the ascription helps us understand the structure of the machine,its past or future behavior,or how to repair or improve it.It is perhaps never logically required even for humans,but expressing reasonably briefly what is actually known about the state of th
31、e machine in a particular situation may require mental qualities or qualities isomorphic to them.Theories of belief,knowledge and wanting can be constructed for machines in a simpler setting than for humans,and later applied to humans.Ascription of mental qualities is most straightforward for machin
32、es of known structure such as thermostats and computer operating systems,but is most useful when applied to entities whose structure is incompletely known.24Agents as Intentional SystemsnWhat objects can be described by the intentional stance?nAs it turns out,more or less anything can.consider a lig
33、ht switch:nBut most adults would find such a description absurd!Why is this?It is perfectly coherent to treat a light switch as a(very cooperative)agent with the capability of transmitting current at will,who invariably transmits current when it believes that we want it transmitted and not otherwise
34、;flicking the switch is simply our way of communicating our desires.(Yoav Shoham)25Agents as Intentional SystemsnThe answer seems to be that while the intentional stance description is consistent,.it does not buy us anything,since we essentially understand the mechanism sufficiently to have a simple
35、r,mechanistic description of its behavior.(Yoav Shoham)nPut crudely,the more we know about a system,the less we need to rely on animistic,intentional explanations of its behaviornBut with very complex systems,a mechanistic,explanation of its behavior may not be practicablenAs computer systems become
36、 ever more complex,we need more powerful abstractions and metaphors to explain their operation low level explanations become impractical.The intentional stance is such an abstraction26Agents as Intentional SystemsnThe intentional notions are thus abstraction tools,which provide us with a convenient
37、and familiar way of describing,explaining,and predicting the behavior of complex systemsnRemember:most important developments in computing are based on new abstractions:qprocedural abstractionqabstract data typesqobjectsAgents,and agents as intentional systems,represent a further,and increasingly po
38、werful abstractionnSo agent theorists start from the(strong)view of agents as intentional systems:one whose simplest consistent description requires the intentional stance27Agents as Intentional SystemsnThis intentional stance is an abstraction tool a convenient way of talking about complex systems,
39、which allows us to predict and explain their behavior without having to understand how the mechanism actually worksnNow,much of computer science is concerned with looking for abstraction mechanisms(witness procedural abstraction,ADTs,objects,)So why not use the intentional stance as an abstraction t
40、ool in computing to explain,understand,and,crucially,program computer systems?nThis is an important argument in favor of agents28Agents as Intentional SystemsnOther 3 points in favor of this idea:nCharacterizing Agents:qIt provides us with a familiar,non-technical way of understanding&explaining age
41、ntsnNested Representations:qIt gives us the potential to specify systems that include representations of other systemsqIt is widely accepted that such nested representations are essential for agents that must cooperate with other agents29Agents as Intentional SystemsnPost-Declarative Systems:qThis v
42、iew of agents leads to a kind of post-declarative programming:nIn procedural programming,we say exactly what a system should donIn declarative programming,we state something that we want to achieve,give the system general info about the relationships between objects,and let a built-in control mechan
43、ism(e.g.,goal-directed theorem proving)figure out what to donWith agents,we give a very abstract specification of the system,and let the control mechanism figure out what to do,knowing that it will act in accordance with some built-in theory of agency(e.g.,the well-known Cohen-Levesque model of inte
44、ntion)30An asidenWe find that researchers from a more mainstream computing discipline have adopted a similar set of ideasnIn distributed systems theory,logics of knowledge are used in the development of knowledge based protocolsnThe rationale is that when constructing protocols,one often encounters
45、reasoning such as the following:IF process i knows process j has received message m1THEN process i should send process j the message m2 nIn DS theory,knowledge is grounded given a precise interpretation in terms of the states of a process;well examine this point in detail later31Abstract Architectur
46、e for AgentsnAssume the environment may be in any of a finite set E of discrete,instantaneous states:nAgents are assumed to have a repertoire of possible actions available to them,which transform the state of the environment:nA run,r,of an agent in an environment is a sequence of interleaved environ
47、ment states and actions:32Abstract Architecture for AgentsnLet:qR be the set of all such possible finite sequences(over E and Ac)qRAc be the subset of these that end with an actionqRE be the subset of these that end with an environment state33State Transformer FunctionsnA state transformer function
48、represents behavior of the environment:nNote that environments areqhistory dependentqnon-deterministicnIf(r)=,then there are no possible successor states to r.In this case,we say that the system has ended its runnFormally,we say an environment Env is a triple Env=E,e0,where:E is a set of environment
49、 states,e0 E is the initial state,and is a state transformer function34AgentsnAgent is a function which maps runs to actions:An agent makes a decision about what action to perform based on the history of the system that it has witnessed to date.Let AG be the set of all agents35SystemsnA system is a
50、pair containing an agent and an environmentnAny system will have associated with it a set of possible runs;we denote the set of runs of agent Ag in environment Env by R(Ag,Env)n(We assume R(Ag,Env)contains only terminated runs)36SystemsnFormally,a sequencerepresents a run of an agent Ag in environme
51、nt Env=E,e0,if:1.e0 is the initial state of Env2.0=Ag(e0);and3.For u 0,37Purely Reactive AgentsnSome agents decide what to do without reference to their history they base their decision making entirely on the present,with no reference at all to the pastnWe call such agents purely reactive:nA thermos
52、tat is a purely reactive agent38PerceptionnNow introduce perception system:EnvironmentAgentseeaction39PerceptionnThe see function is the agents ability to observe its environment,whereas the action function represents the agents decision making processnOutput of the see function is a percept:see:E P
53、erwhich maps environment states to percepts,and action is now a functionaction:Per*Awhich maps sequences of percepts to actions40Agents with StatenWe now consider agents that maintain state:EnvironmentAgentseeactionnextstate41Agents with StatenThese agents have some internal data structure,which is
54、typically used to record information about the environment state and history.Let I be the set of all internal states of the agent.nThe perception function see for a state-based agent is unchanged:see:E Per The action-selection function action is now defined as a mappingaction:I Ac from internal stat
55、es to actions.An additional function next is introduced,which maps an internal state and percept to an internal state:next:I Per I42Agent Control Loop1.Agent starts in some initial internal state i02.Observes its environment state e,and generates a percept see(e)3.Internal state of the agent is then
56、 updated via next function,becoming next(i0,see(e)4.The action selected by the agent is action(next(i0,see(e)5.Goto 243Tasks for AgentsnWe build agents in order to carry out tasks for usnThe task must be specified by usnBut we want to tell agents what to do without telling them how to do it44Utility
57、 Functions over StatesnOne possibility:associate utilities with individual states the task of the agent is then to bring about states that maximize utilitynA task specification is a functionu:E which associates a real number with every environment state45Utility Functions over StatesnBut what is the
58、 value of a runqminimum utility of state on run?qmaximum utility of state on run?qsum of utilities of states on run?qaverage?nDisadvantage:difficult to specify a long term view when assigning utilities to individual states(One possibility:a discount for states later on.)46Utilities over RunsnAnother
59、 possibility:assigns a utility not to individual states,but to runs themselves:u:R nSuch an approach takes an inherently long term viewnOther variations:incorporate probabilities of different states emergingnDifficulties with utility-based approaches:qwhere do the numbers come from?qwe dont think in
60、 terms of utilities!qhard to formulate tasks in these terms47Utility in the TileworldnSimulated two dimensional grid environment on which there are agents,tiles,obstacles,and holesnAn agent can move in four directions,up,down,left,or right,and if it is located next to a tile,it can push itnHoles hav
61、e to be filled up with tiles by the agent.An agent scores points by filling holes with tiles,with the aim being to fill as many holes as possiblenTILEWORLD changes with the random appearance and disappearance of holesnUtility function defined as follows:48The Tileworld,Some ExamplesnFrom Goldman and
62、 Rosenschein,AAAI-94:49The Tileworld,Some ExamplesnFrom Goldman and Rosenschein,AAAI-94:50Expected Utility&Optimal AgentsnWrite P(r|Ag,Env)to denote probability that run r occurs when agent Ag is placed in environment EnvNote:nThen optimal agent Agopt in an environment Env is the one that maximizes
63、expected utility:51Bounded Optimal AgentsnSome agents cannot be implemented on some computers(A function Ag:RE Ac may need more than available memory to implement)nWrite AGm to denote the agents that can be implemented on machine(computer)m:nWe can replace equation(1)with the following,which defines
64、 the bounded optimal agent Agopt:52Predicate Task SpecificationsnA special case of assigning utilities to histories is to assign 0(false)or 1(true)to a runnIf a run is assigned 1,then the agent succeeds on that run,otherwise it failsnCall these predicate task specificationsnDenote predicate task spe
65、cification by.Thus :R 0,1.53Task EnvironmentsnA task environment is a pair Env,where Env is an environment,:R 0,1 is a predicate over runs.Let TE be the set of all task environments.nA task environment specifies:qthe properties of the system the agent will inhabitqthe criteria by which an agent will
66、 be judged to have either failed or succeeded54Task EnvironmentsnWrite R(Ag,Env)to denote set of all runs of the agent Ag in environment Env that satisfy:n We then say that an agent Ag succeeds in task environment Env,if55The Probability of SuccessnLet P(r|Ag,Env)denote probability that run r occurs if agent Ag is placed in environment EnvnThen the probability P(|Ag,Env)that is satisfied by Ag in Env would then simply be:56Achievement&Maintenance TasksnTwo most common types of tasks are achievem
- 温馨提示:
1: 本站所有资源如无特殊说明,都需要本地电脑安装OFFICE2007和PDF阅读器。图纸软件为CAD,CAXA,PROE,UG,SolidWorks等.压缩文件请下载最新的WinRAR软件解压。
2: 本站的文档不包含任何第三方提供的附件图纸等,如果需要附件,请联系上传者。文件的所有权益归上传用户所有。
3.本站RAR压缩包中若带图纸,网页内容里面会有图纸预览,若没有图纸预览就没有图纸。
4. 未经权益所有人同意不得将文件中的内容挪作商业或盈利用途。
5. 装配图网仅提供信息存储空间,仅对用户上传内容的表现方式做保护处理,对用户上传分享的文档内容本身不做任何修改或编辑,并不能对任何下载内容负责。
6. 下载文件中如有侵权或不适当内容,请与我们联系,我们立即纠正。
7. 本站不保证下载资源的准确性、安全性和完整性, 同时也不承担用户因使用这些下载资源对自己和他人造成任何形式的伤害或损失。