模式识别与机器学习:Machine Learning-Introduction
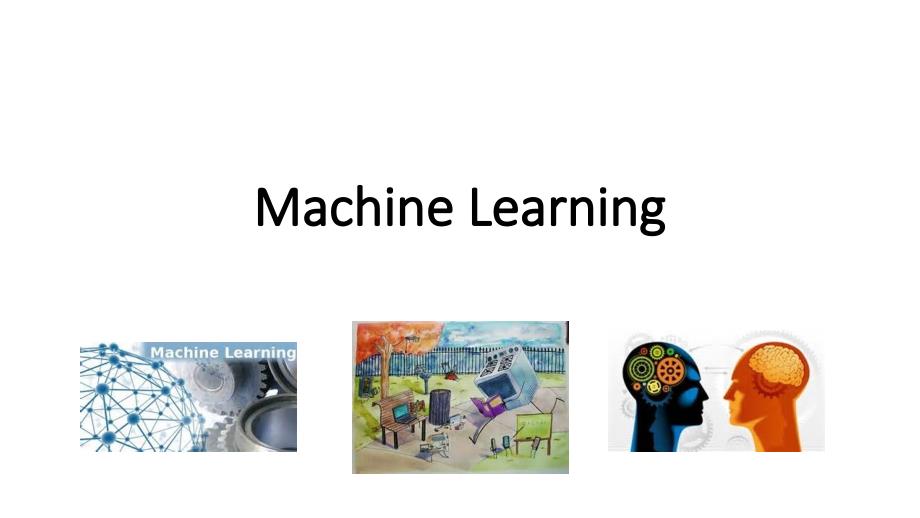


《模式识别与机器学习:Machine Learning-Introduction》由会员分享,可在线阅读,更多相关《模式识别与机器学习:Machine Learning-Introduction(31页珍藏版)》请在装配图网上搜索。
1、Machine LearningMachine LearningSurveySome SuggestionsTeachingTop30%EnglishSlidesElementsRequirementsBasic:ExamEngineering:practiceResearch:theory+practiceRelations with Other FieldsCourses,Books,and ConferencesWhat is Machine Learning?Machinelearningisasubfieldofcomputersciencethatevolvedfromthestu
2、dyofpatternrecognitionandcomputationallearningtheoryinartificialintelligence.Machinelearningexploresthestudyandconstructionofalgorithmsthatcanlearnfromandmakepredictionsondata.Suchalgorithmsoperatebybuildingamodelfromexampleinputsinordertomakedata-drivenpredictionsordecisions,ratherthanfollowingstri
3、ctlystaticprograminstructions.1959,ArthurSamuel:Fieldofstudythatgivescomputerstheabilitytolearnwithoutbeingexplicitlyprogrammed”.TomM.Mitchell:AcomputerprogramissaidtolearnfromexperienceEwithrespecttosomeclassoftasksTandperformancemeasureP,ifitsperformanceattasksinT,asmeasuredbyP,improveswithexperie
4、nceE”.(PACtheory)7Defining the Learning TaskImproveontask,T,withrespecttoperformancemetric,P,basedonexperience,E.T:Categorizeemailmessagesasspamorlegitimate.P:Percentageofemailmessagescorrectlyclassified.E:Databaseofemails,somewithhuman-givenlabelsT:Recognizinghand-writtenwordsP:Percentageofwordscor
5、rectlyclassifiedE:Databaseofhuman-labeledimagesofhandwrittenwordsT:Drivingonfour-lanehighwaysusingvisionsensorsP:Averagedistancetraveledbeforeahuman-judgederrorE:Asequenceofimagesandsteeringcommandsrecordedwhileobservingahumandriver.T:PlayingcheckersP:PercentageofgameswonagainstanarbitraryopponentE:
6、PlayingpracticegamesagainstitselfA Learning ComicExperience:TrainingDataWhatistobelearned:TargetFunctionLearningAlgorithm:howtoinferthetargetfunctionfromtheexperienceEvaluation:TestDataCategories of Machine LearningSupervisedLearning:directlabeledtrainingdataUnsupervisedLearning:unlabeledtrainingdat
7、aSemi-SupervisedLearning:unlabeled+labeledtrainingdataReinforcementLearning:indirectlabeledtrainingdataTransferLearning:trainingandtestdataareNonIIDMulti-TaskLearning:multipletasksharerepresentationActiveLearning:activelychoosetrainingdataHistory of Machine Learning(From Eren Golges Blog)Firststepto
8、wardprevalentMLwasproposedbyHebb,in1949,basedonaneuropsychologicallearningformulation.ItiscalledHebbianLearningtheory.Withasimpleexplanation,itpursuescorrelationsbetweennodesofaRecurrentNeuralNetwork(RNN).Itmemorizesanycommonalitiesonthenetworkandserveslikeamemorylater.Formally,theargumentstatesthat
9、:Letusassumethatthepersistenceorrepetitionofareverberatoryactivity(ortrace)tendstoinducelastingcellularchangesthataddtoitsstability.WhenanaxonofcellAisnearenoughtoexciteacellBandrepeatedlyorpersistentlytakespartinfiringit,somegrowthprocessormetabolicchangetakesplaceinoneorbothcellssuchthatAsefficien
10、cy,asoneofthecellsfiringB,isincreased.History of Machine Learning(From Eren Golges Blog)ArthurSamuelIn1952,ArthurSamuelatIBM,developedaprogramplayingCheckers.Theprogramwasabletoobservepositionsandlearnaimplicitmodelthatgivesbettermovesforthelattercases.Samuelplayedsomanygameswiththeprogramandobserve
11、dthattheprogramwasabletoplaybetterinthecourseoftime.F.RosenblattIn1957,RosenblattsPerceptronwasthesecondmodelproposedagainwithneuroscientificbackgroundanditismoresimilartotodaysMLmodels.ItwasaveryexcitingdiscoveryatthetimeanditwaspracticallymoreapplicablethanHebbiansidea.Theperceptronisdesignedtoill
12、ustratesomeofthefundamentalpropertiesofintelligentsystemsingeneral,withoutbecomingtoodeeplyenmeshedinthespecial,andfrequentlyunknown,conditionswhichholdforparticularbiologicalorganisms.WidrowengravedDeltaLearningrulethatisthenusedaspracticalprocedureforPerceptrontraining.ItisalsoknownasLeastSquarepr
13、oblem.Combinationofthosetwoideascreatesagoodlinearclassifier.History of Machine Learning(From Eren Golges Blog)However,PerceptronsexcitementwashingedbyMinskyin1969.HeproposedthefamousXORproblemandtheinabilityofPerceptronsinsuchlinearlyinseparabledatadistributions.ItwastheMinskystackletoNNcommunity.T
14、hereafter,NNresearcheswouldbedormantupuntil1980sHistory of Machine Learning(From Eren Golges Blog)TherehadbeennottomucheffortuntiltheintuitionofMulti-LayerPerceptron(MLP)wassuggestedbyWerbosin1981withNNspecificBackpropagation(BP)algorithm,albeitBPideahadbeenproposedbeforebyLinnainmaain1970inthenamer
15、eversemodeofautomaticdifferentiation.StillBPisthekeyingredientoftodaysNNarchitectures.Withthosenewideas,NNresearchesacceleratedagain.In1985-1986NNresearcherssuccessivelypresentedtheideaofMLPwithpracticalBPtrainingHecht-Nielsen,Robert.Theoryofthebackpropagationneuralnetwork.Neural Networks,1989.IJCNN
16、.,International Joint Conference on.IEEE,1989.History of Machine Learning(From Eren Golges Blog)Attheanotherspectrum,avery-wellknownMLalgorithmwasproposedbyJ.R.Quinlanin1986thatwecallDecisionTrees,morespecificallyID3algorithm.ThiswasthesparkpointoftheanothermainstreamML.Moreover,ID3wasalsoreleasedas
17、asoftwareabletofindmorereal-lifeusecasewithitssimplisticrulesanditsclearinference,contrarytostillblack-boxNNmodels.AfterID3,manydifferentalternativesorimprovementshavebeenexploredbythecommunity(e.g.ID4,RegressionTrees,CART.)andstillitisoneoftheactivetopicinML.Quinlan,J.Ross.Inductionofdecisiontrees.
18、Machine learning1.1(1986):81-106.History of Machine Learning(From Eren Golges Blog)OneofthemostimportantMLbreakthroughwasSupportVectorMachines(Networks)(SVM),proposedbyVapnikandCortesin1995withverystrongtheoreticalstandingandempiricalresults.ThatwasthetimeseparatingtheMLcommunityintotwocrowdsasNNorS
19、VMadvocates.HoweverthecompetitionbetweentwocommunitywasnotveryeasyfortheNNsideafterKernelizedversionofSVMbynear2000s.(Iwasnotabletofindthefirstpaperaboutthetopic),SVMgotthebestofmanytasksthatwereoccupiedbyNNmodelsbefore.Inaddition,SVMwasabletoexploitalltheprofoundknowledgeofconvexoptimization,genera
20、lizationmargintheoryandkernelsagainstNNmodels.Therefore,itcouldfindlargepushfromdifferentdisciplinescausingveryrapidtheoreticalandpracticalimprovements.Cortes,Corinna,andVladimirVapnik.Support-vectornetworks.Machine learning20.3(1995):273-297.History of Machine Learning(From Eren Golges Blog)NNtooka
21、notherdamagebytheworkofHochreitersthesisin1991andHochreiteret.al.in2001,showingthegradientlossafterthesaturationofNNunitsasweapplyBPlearning.Simplymeans,itisredundanttotrainNNunitsafteracertainnumberofepochsowingtosaturatedunitshenceNNsareveryinclinedtoover-fitinashortnumberofepochs.Littlebefore,ano
22、thersolidMLmodelwasproposedbyFreundandSchapirein1997prescribedwithboostedensembleofweakclassifierscalledAdaboost.ThisworkalsogavetheGodelPrizetotheauthorsatthetime.Adaboosttrainsweaksetofclassifiersthatareeasytotrain,bygivingmoreimportancetohardinstances.Thismodelstillthebasisofmanydifferenttaskslik
23、efacerecognitionanddetection.History of Machine Learning(From Eren Golges Blog)AnotherensemblemodelexploredbyBreimanin2001thatensemblesmultipledecisiontreeswhereeachofthemiscuratedbyarandomsubsetofinstancesandeachnodeisselectedfromarandomsubsetoffeatures.Owingtoitsnature,itiscalledRandomForests(RF).
24、RFhasalsotheoreticalandempiricalproofsofenduranceagainstover-fitting.EvenAdaBoostshowsweaknesstoover-fittingandoutlierinstancesinthedata,RFismorerobustmodelagainstthesecaveats.(FormoredetailaboutRF,refertomyoldpost.).RFshowsitssuccessinmanydifferenttaskslikeKagglecompetitionsaswell.Randomforestsarea
25、combinationoftreepredictorssuchthateachtreedependsonthevaluesofarandomvectorsampledindependentlyandwiththesamedistributionforalltreesintheforest.Thegeneralizationerrorforforestsconvergesa.s.toalimitasthenumberoftreesintheforestbecomeslarge.History of Machine Learning(From Eren Golges Blog)Aswecomecl
26、osertoday,aneweraofNNcalledDeepLearninghasbeencommerced.ThisphrasesimplyrefersNNmodelswithmanywidesuccessivelayers.The3rdriseofNNhasbegunroughlyin2005withtheconjunctionofmanydifferentdiscoveriesfrompastandpresentbyrecentmavensHinton,LeCun,Bengio,AndrewNgandothervaluableolderresearchers.Hinton,G.E.an
27、dSalakhutdinov,R.RReducingthedimensionalityofdatawithneuralnetworks.Science,Vol.313.no.5786,pp.504-507,28July2006.History of Machine Learning(From Eren Golges Blog)History of Machine LearningWiththecombinationofallthoseideasandnon-listedones,NNmodelsareabletobeatoffstateofartatverydifferenttaskssuch
28、asObjectRecognition,SpeechRecognition,NLPetc.However,itshouldbenotedthatthisabsolutelydoesnotmean,itistheendofotherMLstreams.EvenDeepLearningsuccessstoriesgrowrapidly,therearemanycriticsdirectedtotrainingcostandtuningexogenousparametersofthesemodels.AfterthegrowthofWWWandSocialMedia,anewterm,BigData
29、emergedandaffectedMLresearchwildly.BecauseofthelargeproblemsarisingfromBigData,manystrongMLalgorithmsareuselessforreasonablesystems(notforgiantTechCompaniesofcourse).Hence,researchpeoplecomeupwithanewsetofsimplemodelsthataredubbedBanditAlgorithms(formallypredicatedwithOnlineLearning)thatmakeslearnin
30、geasierandadaptableforlargescaleproblems.Applications:Computer VisionSuccess of Deep Learning:Computer VisionApplications:Speech RecognitionSuccess of Deep Learning:Speech RecognitionApplications:Web SearchApplications:Recommender SystemApplications:Social ComputingSuccess of Deep Learning:NLPSuccess of Deep Learning:AlphaGoMore Applications5minBreak
- 温馨提示:
1: 本站所有资源如无特殊说明,都需要本地电脑安装OFFICE2007和PDF阅读器。图纸软件为CAD,CAXA,PROE,UG,SolidWorks等.压缩文件请下载最新的WinRAR软件解压。
2: 本站的文档不包含任何第三方提供的附件图纸等,如果需要附件,请联系上传者。文件的所有权益归上传用户所有。
3.本站RAR压缩包中若带图纸,网页内容里面会有图纸预览,若没有图纸预览就没有图纸。
4. 未经权益所有人同意不得将文件中的内容挪作商业或盈利用途。
5. 装配图网仅提供信息存储空间,仅对用户上传内容的表现方式做保护处理,对用户上传分享的文档内容本身不做任何修改或编辑,并不能对任何下载内容负责。
6. 下载文件中如有侵权或不适当内容,请与我们联系,我们立即纠正。
7. 本站不保证下载资源的准确性、安全性和完整性, 同时也不承担用户因使用这些下载资源对自己和他人造成任何形式的伤害或损失。