TheApplicationofPropensityScoreAnalysistoNon
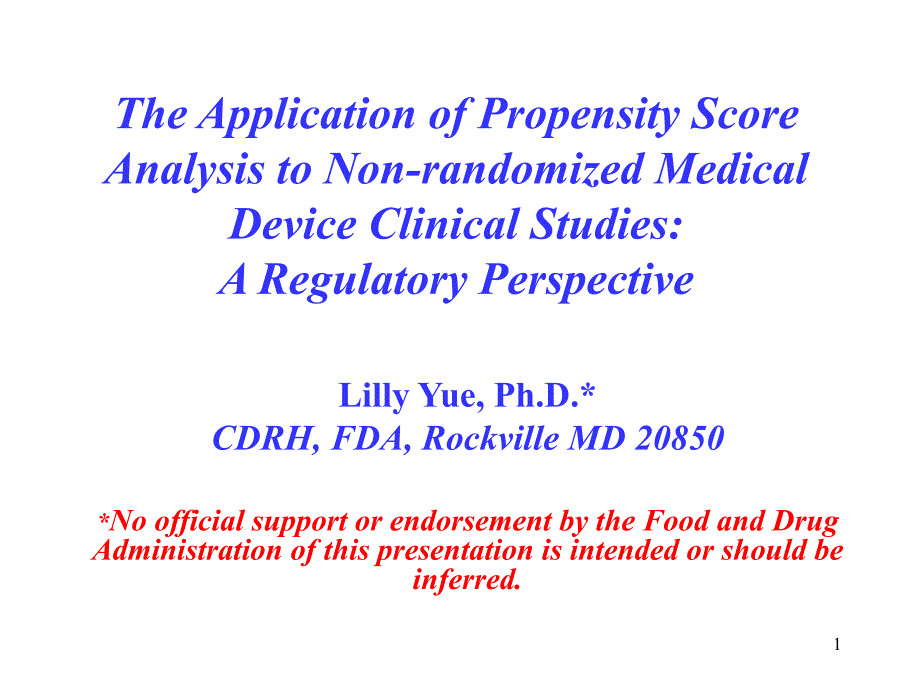


《TheApplicationofPropensityScoreAnalysistoNon》由会员分享,可在线阅读,更多相关《TheApplicationofPropensityScoreAnalysistoNon(45页珍藏版)》请在装配图网上搜索。
1、1The Application of Propensity Score Analysis to Non-randomized Medical Device Clinical Studies:A Regulatory PerspectiveLilly Yue,Ph.D.*CDRH,FDA,Rockville MD 20850*No official support or endorsement by the Food and Drug Administration of this presentation is intended or should be inferred.2OutlineRa
2、ndomized clinical trialsNon-randomized studies and a potential problem Propensity scores methods for bias reductionPractical issues with the application of propensity score methodologyLimitations of propensity score methodsConclusions3Randomized Trials All patients have a specified chance of receivi
3、ng each treatment.Treatments are concurrent.Data collection is concurrent,uniform,and high quality.Expect that all patient covariates,measured or unmeasured,e.g.,age,gender,duration of disease,are balanced between the two treatment groups.4Randomized Trials Assumptions underlying statistical compari
4、son tests are met.So,the two trt groups are comparable and observed treatment difference is an unbiased estimate of true treatment difference.But,the above advantages are not guaranteed for small,poorly designed or poorly conducted randomized trials.5Nonrandomized Studies and a Potential Problem Non
5、e of advantages provided by randomized trials is available in non-randomized studies.A potential problem:Two treatment groups were not comparable before the start of treatment.i.e.,not comparable due to imbalanced covariates between two treatment groups.So,direct treatment comparisons are invalid.6A
6、djustments for Covariates Three common methods of adjusting for confounding covariates:Matching Subclassification(stratification)Regression(Covariate)adjustment7 Question:When there are many confounding covariates needed to adjust for,e.g.,age,gender,Matching based on many covariates is not practica
7、l.Subclassification is difficulty:As the number of covariates increases,the number of subclasses grows exponentially:Each covariate:2 categories 5 covariates:32 subclasses Regression adjustment may not be possible:Potential problem:over-fitting8Propensity Score Methodology Replace the collection of
8、confounding covariates with one scalar function of these covariates:the propensity score.Age GenderDuration.1 composite covariate:Propensity Score Balancing score9Propensity Score Methodology(cont.)Propensity score(PS):conditional prob.of receiving Trt A rather than Trt B,given a collection of obser
9、ved covariates.Purpose:simultaneously balance many covariates in the two trt groups and thus reduce the bias.10 Propensity scores construction Statistical modeling of relationship between treatment membership and covariates Statistical methods:multiple logistic regression or others Outcome:event-act
10、ual trt membership:A or B Predictor variables:all measured covariates,some interaction terms or squared terms,e.g.,age,gender,duration of disease,age*duration,11 Propensity scores construction Clinical outcome variable,e.g.,major complication event,is NOT involved in the modeling No concern of over-
11、fitting Obtain a propensity score model:a math equation PS=f(age,gender,)Calculate estimated propensity scores for all patients12 Properties of propensity scores A group of patients with the same propensity score are equally likely to have been assigned to trt A.Within a group of patients with the s
12、ame propensity score,e.g.,0.7,some patients actually got trt A and some got trt B,just as they had been randomly allocated to whichever trt they actually received.13 “Randomized After the Fact”PS=0.7Trt A Trt B14 When the propensity scores are balanced across two treatment groups,the distribution of
13、 all the covariates are balanced in expectation across the two groups.Use the propensity scores as a diagnostic tool to measure treatment group comparability.If the two treatment groups overlap well enough in terms of the propensity scores,we compare the two treatment groups adjusting for the PS.15
14、Compare treatments adjusting for propensity score Matching Subclassification(stratification)Regression(Covariate)adjustment16Matching based on propensity scores(PS)PS Trt A vs.Trt B Compare treatments based on matched pairs Problem:may exclude unmatched patientsPS1 PS2PSm17StratificationAll patients
15、 are sorted by propensity scores.Divide into equal-sized subclasses.Compare two trts within each subclass,as in a randomized trial;then estimate overall trt effect as weighted average.It is intended to use all patients.But,if trial size is small,some subclass may contain patients from only one treat
16、ment group.PS 12.518Regression(covariate)adjustment Treatment effect estimation model fitting:the relationship of clinical outcome and treatment Outcome:Clinical outcome,e.g.,adverse eventsPredictor variables:trt received,propensity score,a subset of important covariatesStatistical method:e.g.,regre
17、ssion or logistical regression 19Propensity Score Methods SummaryFit propensity score(PS)model using all measured covariatesEstimate PS for all patientsusing PS modelCompare treatments adjusting for propensity scores20Practical IssuesIssues in propensity score estimationHow to handle missing baselin
18、e covariate valuesWhat terms of covariates should be includedEvaluation of treatment group comparability Assessment of the resulting balance of the distributions of covariatesIssues in treatment comparison:Which method:matching,stratification,regressionIssues in study design with PS analysisPre-spec
19、ified vs.post hoc PS analysisPre-specify the covariates needed to collect in the study and then included in PS estimationSample size estimation adjusting for the propensity scores21Example Device A Non-concurrent,two-arm,multi-center study Control:Medical treatment without device,N=65,hospital recor
20、d collection Treatment:Device A,N=130 Primary effectiveness endpoint:Treatment success Hypothesis testing:superiority in success rate 20 imbalanced clinically important baseline covariates,e.g.,prior cardiac surgery 22%patients with missing baseline covariate values22Enrollment Time23 Two treatment
21、groups are not comparable Imbalance in multiple baseline covariates Imbalance in the time of enrollment So,any direct treatment comparisons on the effectiveness endpoint are inappropriate.And,p-values from direct treatment comparisons are un-interpretable.What about treatment comparisons adjusting f
22、or the imbalanced covariates?Traditional covariate analysis Propensity score analysis24 Performed propensity score(PS)analysis Handed missing values MI:generate multiple data sets for PS analysis Generate one data set:generalized PS analysis Others Included all statistically significant and/or clini
23、cally important baseline covariates in PS modeling.Checked comparability of two trt groups through estimated propensity score distributions.Found that the two trt groups did not overlap well.25Estimated Propensity Scores(with time)CtlTrt0.00.20.40.60.81.0Estimated Propensity Score26Estimated Propens
24、ity Scores(w/o time)CtlTrt0.00.20.40.60.81.0Estimated Propensity Score27Patients in Propensity Score Quintile 1 2 3 4 5 Total Ctl 38 18 8 1 0 65(w/time)58%28%12%2%0%Trt 1 21 31 38 39 130 1%16%24%29%30%Ctl 29 24 8 4 0 65(w/o time)45%37%12%6%0%Trt 10 14 32 35 39 130 8%11%24%27%30%28Treatment Success 1
25、 2 3 4 5 Total Crl S 16 8 1 0 25 N 38 18 8 1 0 65 Trt S 0 14 25 24 23 86 N 1 21 31 38 39 130 Tried Cochran-Mantel-Haenszel test controlling for PS quintile,Logistic regression using PS as a continuous covariate However,the sig.p-values are un-interpretable 29 Conclusion:The two treatment groups did
26、not overlap enough to allow a sensible treatment comparison.So,any treatment comparisons adjusting for imbalanced covariates are problematic.30Example:Device B New vs.control in a non-randomized study Primary endpoint:MACE incidence rate at 6-month after treatment Non-inferiority margin:7%,in this s
27、tudy Sample size:new:290,control:560 14 covariates were considered.31 Covariate balance checking before and after propensity score stratification adjustment Mean p-value New Control Before After-Mi 0.25 0.40 .0001 0.4645 Diab 0.28 0.21 0.0421 0.8608 CCS 2.41 2.75 0.0003 0.3096 Lesleng 11.02 12.16 .0
28、001 0.5008 Preref 3.00 3.08 0.0202 0.2556 Presten 62.75 66.81 .0001 0.405332Model Building The PS is conditional Prob.that a patient would have been assigned to new device,based on his or her baseline covariates.A hierarchical logistic regression model with a stepwise selection process was used to b
29、uild the propensity score model.The final propensity score model includes all covariates as well as a quadratic term.33Table 2.Distribution of patients at five strata Subclass Control New Total 1 142 28 170 2 127 43 170 3 122 48 170 4 119 51 170 50 120 170Total 560 290 85034Estimated Propensity Scor
30、esN(new)=560,N(control)=290ControlNew0.00.20.40.60.81.0Estimated Propensity Score35 Covariate balance checking before and after propensity score stratification adjustment Mean p-value New Control Before After-Mi 0.25 0.40 .0001 0.4645 Diab 0.28 0.21 0.0421 0.8608 CCS 2.41 2.75 0.0003 0.3096 Lesleng
31、11.02 12.16 .0001 0.5008 Preref 3.00 3.08 0.0202 0.2556 Presten 62.75 66.81 .0001 0.405336After adj.balance check:Prior Mi rate:Overall:Group%patients with prior Mi New 25 Control 40 Diff 15 After:Quintile Group 1 2 3 4 5 New 70.4 32.6 25.0 17.6 15.0 Control 75.2 32.8 30.0 24.8 10.437Percentage of p
32、atients with prior Mi38 Adjusted Difference:Mew Control:Point estimate:-1.5%2-sided 95%C.I.:(-6.6%,3.6%)Non-inferiority margin:7%Claim:Non-inferiority w.r.t.Mace 6-month39Study Design Plan in advance Pre-specify clinically relevant baseline covariates:as many as possible Sample size estimation:Ignor
33、e the propensity score adjustment?Could be inappropriate40Limitations Propensity score methods can only adjust for observed confounding covariates and not for unobserved ones.Propensity score is seriously degraded when important variables influencing selection have not been collected.Propensity scor
34、e may not eliminate all selection bias.41Limitations Propensity score methods work better in larger samples.Propensity score is not only way of adjusting for covariates.And,it may or may not be helpful in a particular comparison study.Randomized trials are considered the highest level of evidence fo
35、r trt comparison.Propensity score methods lack the discipline and rigor of randomized trials,and not as definitive as randomized trials.42Conclusions Propensity score methods generalize technique with one confounding covariate to allow simultaneous adjustment for many covariates and thus reduce bias
36、.Propensity score methodology is an addition to,not a substitute of traditional covariate adjustment methods.Plan ahead and carefully consider the practical issues discussed above.Randomized studies are still preferred and strongly encouraged whenever possible!43References Rubin,DB,Estimating casual
37、 effects from large data sets using propensity scores.Ann Intern Med 1997;127:757-763 Rosenbaum,PR,Rubin DB,Reducing bias in observational studies using subclassification on the propensity score.JASA 1984;79:516-524 Dagostino,RB,Jr.,Propensity score methods for bias reduction in the comparison of a
38、treatment to a non-randomized control group,Statistics in medicine,1998,17:2265-228144References Blackstone,EH,Comparing apples and oranges,J.Thoracic and Cardiovascular Surgery,January 2002;1:8-15 Grunkemeier,GL and et al,Propensity score analysis of stroke after off-pump coronary artery bypass grafting,Ann Thorac Surg 2002;74:301-305 Wolfgang,C.and et al,Comparing mortality of elder patients on hemodialysis versus peritoneal dialysis:A propensity score approach,J.Am Soc Nephrol 2002;13:2353-236245 Thanks!
- 温馨提示:
1: 本站所有资源如无特殊说明,都需要本地电脑安装OFFICE2007和PDF阅读器。图纸软件为CAD,CAXA,PROE,UG,SolidWorks等.压缩文件请下载最新的WinRAR软件解压。
2: 本站的文档不包含任何第三方提供的附件图纸等,如果需要附件,请联系上传者。文件的所有权益归上传用户所有。
3.本站RAR压缩包中若带图纸,网页内容里面会有图纸预览,若没有图纸预览就没有图纸。
4. 未经权益所有人同意不得将文件中的内容挪作商业或盈利用途。
5. 装配图网仅提供信息存储空间,仅对用户上传内容的表现方式做保护处理,对用户上传分享的文档内容本身不做任何修改或编辑,并不能对任何下载内容负责。
6. 下载文件中如有侵权或不适当内容,请与我们联系,我们立即纠正。
7. 本站不保证下载资源的准确性、安全性和完整性, 同时也不承担用户因使用这些下载资源对自己和他人造成任何形式的伤害或损失。