IEEE粒子滤波PPT
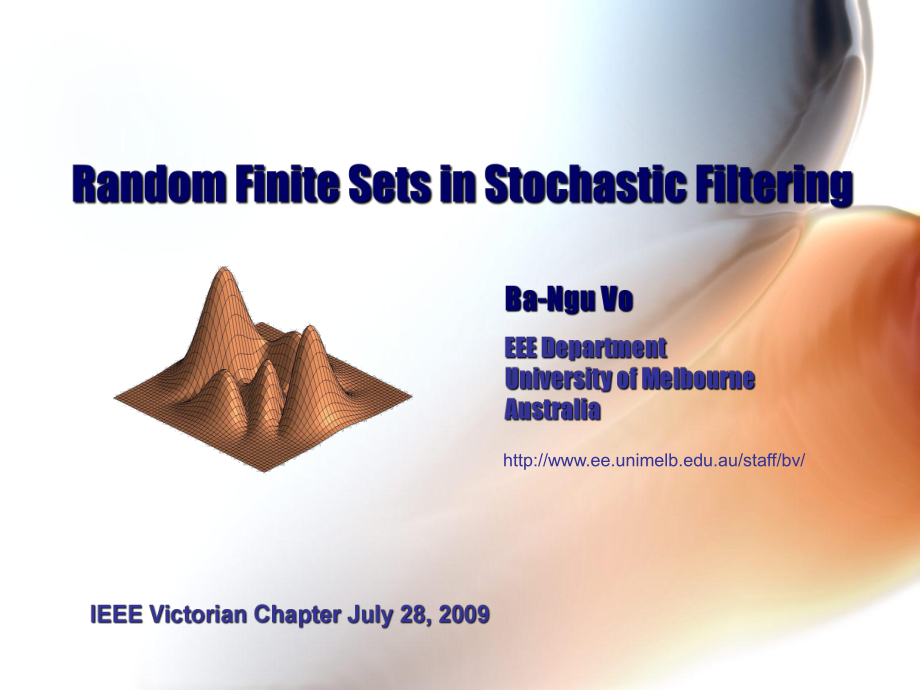


《IEEE粒子滤波PPT》由会员分享,可在线阅读,更多相关《IEEE粒子滤波PPT(43页珍藏版)》请在装配图网上搜索。
1、http:/www.ee.unimelb.edu.au/staff/bv/N.Wiener(1894-1964)A.N.Kolmogorov(1903-1987)R.E.Kalman(1930-)state-vectorstate dynamicstate spaceobservation spacexkxk-1zk-1zk fk|k-1(xk|xk-1)Markov Transition DensityMeasurement Likelihoodgk(zk|xk)Objectivemeasurement history(z1,zk)posterior pdf of the statepk(x
2、k|z1:k)System Modelstate-vectorstate dynamicstate spaceobservation spacexkxk-1zk-1zkBayes filterpk-1(xk-1|z1:k-1)pk|k-1(xk|z1:k-1)pk(xk|z1:k)predictiondata-update pk-1(xk-1|z1:k-1)dxk-1 fk|k-1(xk|xk-1)gk(zk|xk)pk|k-1(xk|z1:k-1)fk|k-1(xk|xk-1)gk(zk|xk)gk(zk|xk)pk-1(xk-1|z1:k-1)dxkstate-vectorstate dy
3、namicstate spaceobservation spacexkxk-1zk-1zk fk|k-1(xk|xk-1)gk(zk|xk)pk-1(.|z1:k-1)pk|k-1(.|z1:k-1)pk(.|z1:k)predictiondata-updateBayes filterN(.;mk-1,Pk-1)N(.;mk|k-1,Pk|k-1)N(.;mk,Pk)Kalman filteri=1Nwk|k-1,xk|k-1i=1N(i)(i)wk,xk i=1 N(i)(i)wk-1,xk-1(i)(i)Particle filterstate-vectorstate dynamicsta
4、te spaceobservation spacexkxk-1zk-1zk fk|k-1(xk|xk-1)gk(zk|xk)Not detectedDetectedor Number of false observationsunknown random False+Observation=Not detectedDetectedFalsestate-vectorstate dynamicstate spaceobservation spacexkxk-1zk-1zkobservation produced by objectsstate dynamicstate spaceobservati
5、on space5 objects3 objectsXk-1XkObjective:Jointly estimate the number&states of objectsNumerous applications:defence,surveillance,robotics,biomed,Challenges:Random number of objects and measurementsDetection uncertainty,clutter,association uncertaintyTrueMulti-object stateEstimatedMulti-object state
6、|2XX2 objects2 objects()min|0perm XXX00 11 00 11 TrueMulti-object stateEstimatedMulti-object State2 objectsno objectTrueMulti-object stateEstimatedMulti-object State2 objects1 object 00 11 00 11 00 ()min|0perm XXXstatesmulti-object statemulti-object observation X X observations X Z pk-1(Xk-1|Z1:k-1)
7、pk(Xk|Z1:k)pk|k-1(Xk|Z1:k-1)predictiondata-update sample u uniform0,1if u r,sample x p(.),end;EESample n Poiss(r),for i=1:n,sample xi p(.),end;ESample n c(.),for i=1:n,sample xi p(.),end;pk-1(Xk-1|Z1:k-1)pk(Xk|Z1:k)pk|k-1(Xk|Z1:k-1)predictiondata-update?statesmulti-object statemulti-object observati
8、on X X observations X ZMulti-object Bayes filterBelief“density”of f:F(E)0,)b(T)=T f(X)dXBelief“distribution”of b(T)=P(T),T EEProbability density of p:F(E)0,)P(T)=T p(X)m(dX)Probability distribution of P(T)=P(T ),T F(E)F(E)Collection of finite subsets of E State space Mahlers Finite Set Statistics(19
9、94)Choquet(1968)TTConventional integralSet integralPoint Process Theory(1950-1960s)VSD(2005)Computationally expensive!single-object Bayes filter multi-object Bayes filter state of system:random vectorfirst-moment filter(e.g.a-b-g filter)state of system:random setfirst-moment filter(“PHD”filter)Singl
10、e-object Multi-object pk-1(Xk-1|Z1:k-1)pk(Xk|Z1:k)pk|k-1(Xk|Z1:k-1)predictiondata-update Multi-object Bayes filterx0state spacev PHD(intensity function)of an RFS S v(x)dx=expected number of objects in SSv(x0)=density of expected number of objects at x0state space vk vk-1 PHD filter Mahler 03 vk-1(xk
11、-1|Z1:k-1)vk(xk|Z1:k)vk|k-1(xk|Z1:k-1)PHD predictionPHD update Multi-object Bayes filter pk-1(Xk-1|Z1:k-1)pk(Xk|Z1:k)pk|k-1(Xk|Z1:k-1)predictionupdate Avoids data association!vk|k-1(xk|Z1:k-1)=fk|k-1(xk,xk-1)vk-1(xk-1|Z1:k-1)dxk-1+gk(xk)intensity from previoustime-step term for spontaneousobject bir
12、thsfk|k-1(xk,xk-1)=ek|k-1(xk-1)fk|k-1(xk|xk-1)+bk|k-1(xk|xk-1)Markovtransitionintensityprobabilityof objectsurvivalterm for objectsspawned byexisting objectsMarkov transition densitypredictedintensityNk|k-1=vk|k-1(x|Z1:k-1)dxpredicted expected number of objects(Fk|k-1a)(xk)fk|k-1(xk,x)a(x)dx+gk(xk)v
13、k|k-1 Fk|k-1vk-1 vk(xk|Z1:k)zZkDk(z)+kk(z)pD,k(xk)gk(z|xk)+1 pD,k(xk)vk|k-1(xk|Z1:k-1)Dk(z)=pD,k(x)gk(z|x)vk|k-1(x|Z1:k-1)dx Nk=vk(x|Z1:k)dxBayes-updated intensitypredicted intensity(from previous time)intensity offalse alarmssensor likelihood functionprobabilityof detectionexpected number of object
14、smeasurementvk Ykvk|k-1(Yka)(x)=zZk+kk(z)yk,z(x)+1 pD,k(x)a(x)vk-1(.|Z1:k-1)vk(.|Z1:k)vk|k-1(.|Z1:k-1)1|Fkk kY wk-1,xk-1j=1Jk-1(j)(j)j=1Jk|k-1(j)(j)wk|k-1,xk|k-1 wk,xk j=1 Jk(j)(j)wk-1,mk-1,Pk-1j=1Jk-1(j)(j)(j)wk|k-1,mk|k-1,Pk|k-1j=1Jk|k-1(j)(j)(j)wk,mk,Pk j=1 Jk(j)(j)(j)Data courtesy of Czyz et.al.
15、Data courtesy of K.Smith IDIAP Research Institute.CPHD filter Mahler 06,07,Gaussian Mixture CPHD filter VVC 06,07 vk-1(xk-1|Z1:k-1)vk(xk|Z1:k)vk|k-1(xk|Z1:k-1)intensity predictionintensity update ck-1(n|Z1:k-1)ck(n|Z1:k)ck|k-1(n|Z1:k-1)cardinality predictioncardinality update Courtesy of Lockheed Ma
16、rtinCourtesy of Lockheed MartinOSPA distance(satisfies all metric axioms)=per target cardinality&state error0102030405060708090100050010001500TimeCardinality TrueEstimate1020304050607080901000102030TimeOSPA(km)1020304050607080901000102030TimeOSPA Loc(km)1020304050607080901000102030TimeOSPA Card(km)R
17、obot poseMapMeasurementsControlsMeasurement likelihoodSet integralTransition densityRFS-SLAM predictionRFS-SLAM updateSet integralRFS-SLAM Mullane et.al.08PHD of the posterior map RFSExperiment:Nanyang Technological University Campus Low clutter:All 3 algorithms can close the loopHigher clutter:Only
18、 PHD-SLAM can close the loopGround truth plotted in greenThank You!For more info please see http:/randomsets.ee.unimelb.edu.auSee also:http:/www.ee.unimelb.edu.au/staff/bv/publications.htmlBooksD.Daley and D.Vere-Jones,An Introduction to the Theory of Point Processes,Springer-Verlag,1988.D.Stoyan,D.
19、Kendall,J.Mecke,Stochastic Geometry and its Applications,John Wiley&Sons,1995I.Goodman,R.Mahler,and H.Nguyen,Mathematics of Data Fusion.Kluwer Academic Publishers,1997.R.Mahler,Statistical Multisource-Multitarget Information Fusion,ArtechHouse,2007.M.Mallick,V.Krisnamurthy,B.-N.Vo(eds),Advanced Topi
20、cs and Applications in Integrated Tracking,Classification,and Sensor Management,IEEE-Wiley(under review)PapersR.Mahler,“Multi-target Bayes filtering via first-order multi-target moments,”IEEE Trans.AES,vol.39,no.4,pp.11521178,2003.B.-N.Vo,S.Singh,and A.Doucet,“Sequential Monte Carlo methods for mult
21、i-target filtering with random finite sets,”IEEE Trans.AES,vol.41,no.4,pp.12241245,2005.B.-N.Vo,and W.K.Ma,“The Gaussian mixture PHD filter,”IEEE Trans.Signal Processing,IEEE Trans.Signal Processing,Vol.54,No.11,pp.4091-4104,2006.R.Mahler,“PHD filter of higher order in target number,”IEEE Trans.Aero
22、space&Electronic Systems,vol.43,no.4,pp.15231543,2007B.T.Vo,B.-N.Vo,and A.Cantoni,Analytic implementations of the Cardinalized Probability Hypothesis Density Filter,IEEE Trans.Signal Processing,Vol.55,No.7,Part 2,pp.3553-3567,2007.B.-T.Vo,B.-N Vo,and A.Cantoni,The Cardinality Balanced Multi-target Multi-Bernoulli filter and its implementations,IEEE Trans.Signal Processing,vol.57,no.2,pp.409423,2009.J.Mullane,B.-N.Vo,M.Adams and S.Wijesoma,A Random Set Formulation for Bayesian SLAM,International Conference on Intelligent Robots and Systems,Nice,France,2008.
- 温馨提示:
1: 本站所有资源如无特殊说明,都需要本地电脑安装OFFICE2007和PDF阅读器。图纸软件为CAD,CAXA,PROE,UG,SolidWorks等.压缩文件请下载最新的WinRAR软件解压。
2: 本站的文档不包含任何第三方提供的附件图纸等,如果需要附件,请联系上传者。文件的所有权益归上传用户所有。
3.本站RAR压缩包中若带图纸,网页内容里面会有图纸预览,若没有图纸预览就没有图纸。
4. 未经权益所有人同意不得将文件中的内容挪作商业或盈利用途。
5. 装配图网仅提供信息存储空间,仅对用户上传内容的表现方式做保护处理,对用户上传分享的文档内容本身不做任何修改或编辑,并不能对任何下载内容负责。
6. 下载文件中如有侵权或不适当内容,请与我们联系,我们立即纠正。
7. 本站不保证下载资源的准确性、安全性和完整性, 同时也不承担用户因使用这些下载资源对自己和他人造成任何形式的伤害或损失。