毕业论文外文翻译-代理控制交通灯
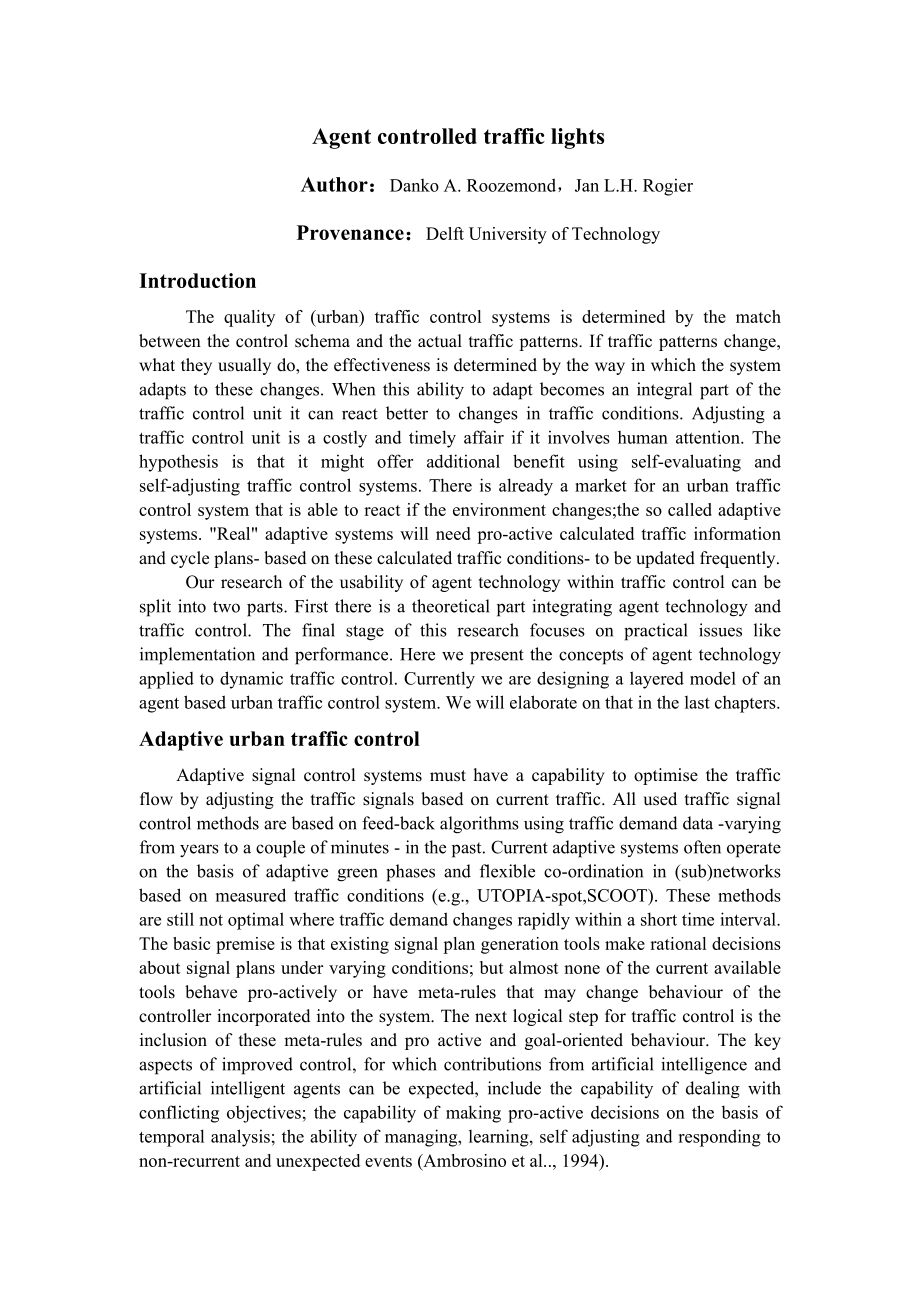


《毕业论文外文翻译-代理控制交通灯》由会员分享,可在线阅读,更多相关《毕业论文外文翻译-代理控制交通灯(15页珍藏版)》请在装配图网上搜索。
1、Agent controlled traffic lightsAuthor:Danko A. Roozemond,Jan L.H. Rogier Provenance:Delft University of TechnologyIntroductionThe quality of (urban) traffic control systems is determined by the match between the control schema and the actual traffic patterns. If traffic patterns change, what they us
2、ually do, the effectiveness is determined by the way in which the system adapts to these changes. When this ability to adapt becomes an integral part of the traffic control unit it can react better to changes in traffic conditions. Adjusting a traffic control unit is a costly and timely affair if it
3、 involves human attention. The hypothesis is that it might offer additional benefit using self-evaluating and self-adjusting traffic control systems. There is already a market for an urban traffic control system that is able to react if the environment changes;the so called adaptive systems. Real ad
4、aptive systems will need pro-active calculated traffic information and cycle plans- based on these calculated traffic conditions- to be updated frequently.Our research of the usability of agent technology within traffic control can be split into two parts. First there is a theoretical part integrati
5、ng agent technology and traffic control. The final stage of this research focuses on practical issues like implementation and performance. Here we present the concepts of agent technology applied to dynamic traffic control. Currently we are designing a layered model of an agent based urban traffic c
6、ontrol system. We will elaborate on that in the last chapters.Adaptive urban traffic control Adaptive signal control systems must have a capability to optimise the traffic flow by adjusting the traffic signals based on current traffic. All used traffic signal control methods are based on feed-back a
7、lgorithms using traffic demand data -varying from years to a couple of minutes - in the past. Current adaptive systems often operate on the basis of adaptive green phases and flexible co-ordination in (sub)networks based on measured traffic conditions (e.g., UTOPIA-spot,SCOOT). These methods are sti
8、ll not optimal where traffic demand changes rapidly within a short time interval. The basic premise is that existing signal plan generation tools make rational decisions about signal plans under varying conditions; but almost none of the current available tools behave pro-actively or have meta-rules
9、 that may change behaviour of the controller incorporated into the system. The next logical step for traffic control is the inclusion of these meta-rules and pro active and goal-oriented behaviour. The key aspects of improved control, for which contributions from artificial intelligence and artifici
10、al intelligent agents can be expected, include the capability of dealing with conflicting objectives; the capability of making pro-active decisions on the basis of temporal analysis; the ability of managing, learning, self adjusting and responding to non-recurrent and unexpected events (Ambrosino et
11、 al., 1994).What are intelligent agents Agent technology is a new concept within the artificial intelligence (AI). The agent paradigm in AI is based upon the notion of reactive, autonomous, internally-motivated entities that inhabit dynamic, not necessarily fully predictable environments (Weiss, 199
12、9). Autonomy is the ability to function as an independent unit over an extended period of time, performing a variety of actions necessary to achieve pre-designated objectives while responding to stimuli produced by integrally contained sensors (Ziegler, 1990). Multi-Agent Systems can be characterise
13、d by the interaction of many agents trying to solve a variety of problems in a co-operative fashion. Besides AI, intelligent agents should have some additional attributes to solve problems by itself in real-time; understand information; have goals and intentions; draw distinctions between situations
14、; generalise; synthesise new concepts and / or ideas; model the world they operate in and plan and predict consequences of actions and evaluate alternatives. The problem solving component of an intelligent agent can be a rule-based system but can also be a neural network or a fuzzy expert system. It
15、 may be obvious that finding a feasible solution is a necessity for an agent. Often local optima in decentralised systems, are not the global optimum. This problem is not easily solved. The solution has to be found by tailoring the interaction mechanism or to have a supervising agent co-ordinating t
16、he optimisation process of the other agents.Intelligent agents in UTC,a helpful paradigmAgent technology is applicable in different fields within UTC. The ones most important mentioning are: information agents, agents for traffic simulation and traffic control. Currently, most applications of intell
17、igent agents are information agents. They collect information via a network. With special designed agents user specific information can be provided. In urban traffic these intelligent agents are useable in delivering information about weather, traffic jams, public transport, route closures, best rou
18、tes, etc. to the user via a Personal Travel Assistant. Agent technology can also be used for aggregating data for further distribution. Agents and multi agent systems are capable of simulating complex systems for traffic simulation. These systems often use one agent for every traffic participant (in
19、 a similar way as object oriented programs often use objects). The application of agents in (Urban) Traffic Control is the one that has our prime interest. Here we ultimately want to use agents for pro-active traffic light control with on-line optimisation. Signal plans then will be determined based
20、 on predicted and measured detector data and will be tuned with adjoining agents. The most promising aspects of agent technology, the flexibility and pro-active behaviour, give UTC the possibility of better anticipation of traffic. Current UTC is not that flexible, it is unable to adjust itself if s
21、ituations change and cant handle un-programmed situations. Agent technology can also be implemented on several different control layers. This gives the advantage of being close to current UTC while leaving considerable freedom at the lower (intersection) level.Designing agent based urban traffic con
22、trol systemsThe ideal system that we strive for is a traffic control system that is based on actuated traffic controllers and is able to pro actively handle traffic situations and handling the different, sometimes conflicting, aims of traffic controllers. The proposed use of the concept of agents in
23、 this research is experimental.Assumptions and considerations on agent based urban traffic controlThere are three aspects where agent based traffic control and -management can improve current state of the art UTC systems:- Adaptability. Intelligent agents are able to adapt its behaviour and can lear
24、n from earlier situations.- Communication. Communication makes it possible for agents to co-operate and tune signal plans.- Pro-active behaviour. Due to the pro active behaviour traffic control systems are able to plan ahead.To be acceptable as replacement unit for current traffic control units, the
25、 system should perform the same or better than current systems. The agent based UTC will require on-line and pro-active reaction on changing traffic patterns. An agent based UTC should be demand responsive as well as adaptive during all stages and times. New methods for traffic control and traffic p
26、rediction should be developed as current ones do not suffice and cannot be used in agent technology. The adaptability can also be divided in several different time scales where the system may need to handle in a different way (Rogier, 1999):- gradual changes due to changing traffic volumes over a lo
27、nger period of time,- abrupt changes due to changing traffic volumes over a longer period of time,- abrupt, temporal, changes due to changing traffic volumes over a short period of time,- abrupt, temporal, changes due to prioritised traffic over a short period of timeOne way of handling the balance
28、between performance and complexity is the use of a hierarchical system layout. We propose a hierarchy of agents where every agent is responsible for its own optimal solution, but may not only be influenced by adjoining agents but also via higher level agents. These agents have the task of solving co
29、nflicts between lower level agents that they cant solve. This represents current traffic control implementations and ideas. One final aspect to be mentioned is the robustness of agent based systems (if all communication fails the agent runs on, if the agent fails a fixed program can be executed.To b
30、e able to keep our first urban traffic control model as simple as possible we have made the following assumptions: we limit ourselves to inner city traffic control (road segments, intersections, corridors), we handle only controlled intersections with detectors (intensity and speed) at all road segm
31、ents, we only handle cars and we use simple rule bases for knowledge representation.Types of agents in urban intersection control As we divide the system in several, recognisable, parts we define the following 4 types of agents:- Roads are represented by special road segment agents (RSA),- Controlle
32、d intersections are represented by intersection agents (ITSA),- For specific, defined, areas there is an area agent (higher level),- For specific routes there can be route agents, that spans several adjoining road segments (higher level).We have not chosen for one agent per signal. This may result i
33、n a more simple solution but available traffic control programs do not fit in that kind of agent. We deliberately choose a more complex agent to be able to use standard traffic control design algorithms and programs. The idea still is the optimisation on a local level (intersection), but with local
34、and global control. Therefor we use area agents and route agents. All communication takes place between neighbouring agents and upper and lower level ones.Design of our agent based systemThe essence of a, demand responsive and pro-active agent based UTC consists of several ITSAs (InTerSection Agent)
35、.,some authority agents (area and route agents) and optional Road Segment Agents (RSA). The ITSA makes decisions on how to control its intersection based on its goals, capability, knowledge, perception and data. When necessary an agent can request for additional information or receive other goals or
36、 orders from its authority agent(s).For a specific ITSA, implemented to serve as an urban traffic control agent, the following actions are incorporated (Roozemond, 1998):- data collection / distribution (via RSA - information on the current state of traffic; from / to other ITSAs - on other adjoinin
37、g signalised intersections);- analysis (with an accurate model of the surrounds and knowing the traffic and traffic control rules define current trend; detect current traffic problems);- calculation (calculate the next, optimal, cycle mathematically correct);- decision making (with other agent decid
38、ing what to use for next cycle; handle current traffic problems);- control (operate the signals according to cycle plan).In figure 1 a more specific example of a simplified, agent based, UTC system is given. Here we have a route agent controlling several intersection agents, which in turn manage the
39、ir intersection controls helped by RSAs. The ITSA is the agent that controls and operates one specific intersection of which it is completely informed. All ITSAs have direct communication with neighbouring ITSAs, RSAs and all its traffic lights. Here we use the agent technology to implement a distri
40、buted planning algorithm. The route agents tasks are controlling, co-ordinating and leading the ITSAs towards a more global optimum. Using all available information the ITSA (re)calculates the next, most optimal, states and control strategy and operates the traffic signals accordingly. The ITSA can
41、directly influence the control strategy of their intersection(s) and is able to get insight into on-coming trafficThe internals of the ITSA modelTraffic dependent intersection control normally works in a fast loop. The detector data is fed into the control algorithm. Based upon predetermined rules a
42、 control strategy is chosen and the signals are operated accordingly. In this research we suggest the introduction of an extra, slow, loop where rules and parameters of a prediction- model can be changed by a higher order meta-model.ITSA modelThe internals of an ITSA consists of several agents. For
43、a better overview of the internal ITSA model-agents and agent based functions see figure 2. Data collection is partly placed at the RSAs and partly placed in the ITSAs. The needed data is collected from different sources, but mainly via detectors. The data is stored locally and may be transmitted to
44、 other agents. The actual operation of the traffic signals is left to an ITSA-controller agent. The central part of the ITSA, acts as a control strategy agent. That agent can operate several control strategies, such as anti-blocking and public transport priority strategies. The control strategy agen
45、t uses the estimates of the prediction model agent which estimates the states in the near future. The ITSA-prediction model agent estimates the states in the near future. The prediction model agent gets its data related to intersection and road segments - as an agent that knows the forecasting equat
46、ions, actual traffic conditions and constraints - and future traffic situations can be calculated by way of an inference engine and its knowledge and data base. On-line optimisation only works if there is sufficient quality in traffic predictions, a good choice is made regarding the performance indi
47、cators and an effective way is found to handle one-time occurrences (Rogier, 1999).Prediction modelWe hope to include pro-activeness via specific prediction model agents with a task of predicting future traffic conditions. The prediction models are extremely important for the development of pro acti
48、ve traffic control. The proposed ITSA-prediction model agent estimates the states of the traffic in the near future via its own prediction model. The prediction meta-model compares the accuracy of the predictions with current traffic and will adjust the prediction parameters if the predictions were
49、insufficient or not accurate. The prediction model agent is fed by several inputs: vehicle detection system, relevant road conditions, control strategies, important data on this intersection and its traffic condition, communication with ITSAs of nearby intersections and higher level agents. The agen
50、t itself has a rule-base, forecasting equations, knows constraints regarding specific intersections and gets insight into current (traffic) conditions. With these data future traffic situations should be calculated by its internal traffic forecasting model. The predicted forecast is valid for a limi
51、ted time. Research has shown that models using historic, up-stream and current link traffic give the best results (Hobeika & Kim, 1994).Control strategy modelThe prediction of the prediction model is used in the control strategy planning phase. We have also included a performance indicating agent, n
52、ecessary to update the control parameters in the slower loop. The control strategy agent uses the estimates of the prediction model agent to calculate the most optimal control strategy to pro-act on the forecasts of the prediction model agent, checks with other adjoining agents its proposed traffic
53、control schema and then plans the signal control strategy The communication schema is based on direct agent to agent communication via a network link. The needed negotiation finds place via a direct link and should take the global perspective into consideration. Specific negotiation rules still have
54、 to be developed. Some traffic regulation rules and data has to be fed into the system initially. Data on average flow on the links is gained by the system during run-time. In the near future computer based programs will be able to do, parts of, these kind of calculus automatically. For real-time co
55、ntrol the same basic computer programs, with some artificial knowledge, will be used. Detectors are needed to give information about queues and number of vehicles. The arrival times can also be given by the RSA so that green on demand is automatically covered.Conclusions and future workAdaptive sign
56、al control systems that are able to optimise and adjust the signal settings are able to improve the vehicular throughput and minimise delay through appropriate response to changes in the measured demand patterns. With the introduction of two un-coupled feed back loops, whether agent technology is us
57、ed or not, a pro-active theory of traffic control can be met. There are several aspects still unresearched. The first thing we are going to do is to build a prototype system of a single intersection to see if the given claims of adaptability and pro activeness can be realised. A working prototype of
58、 such system should give appropriate evidence on the usability of agent based control systems. There are three other major subjects to be researched in depth; namely self adjustable control schemas, on-line optimisation of complex systems and getting good prediction models. For urban traffic control
59、 we need to develop self adjustable control schemes that can deal with dynamic and actuated data. For the optimisation we need mathematical programming methodologies capable of real-time on-line operation. In arterial and agent based systems this subject becomes complex due to several different, con
60、tinuously changing, weights and different goals of the different ITSAs and due to the need for co-ordination and synchronisation. The research towards realising real-time on-line prediction models needs to be developed in compliance with agent based technology. The pro-active and re-active nature of
61、 agents and the double loop control schema seems to be a helpful paradigm in intelligent traffic management and control. Further research and simulated tests on a control strategy, based on intelligent autonomous agents, is necessary to provide appropriate evidence on the usability of agent-based co
62、ntrol systems. 代理控制交通灯作者:Danko A. Roozemond,Jan L.H. Rogier出处:Delft University of Technology前言(城市)交通控制系统的好坏决定于系统控制模式和实际交通流量模式是否相符。如果交通方式改变,他们通常所采取的有效措施是使系统适应这些改变。当这种改变能力成为交通控制系统单元的一部分,它将能更好地改变目前的交通状况。如果考虑人工调整,那么调整交通控制单元是一件花费昂贵和耗时大的事情。假如使用一种能够自我分析和自适应调整的交通控制系统,它将会带来额外的收益。对于这种可以适应环境改变的城市交通控制系统已经有销售。这种
63、系统叫做自适应系统。“实时”自适应系统将需要主动计算交通信息和循环地计划,这种计划是根据不断计算更新的交通信息而采取的。我们研究代理可用性技术在交通控制时可以分成两部分。首先,理论部分结合代理技术和交通控制。这项研究的最后阶段关注的是实际问题,如实施和收到的成效。在这里,我们目前的代理技术应用于动态交通控制概念。目前,我们正在设计一个代理分层模型为基础的城市交通控制系统。我们将在最后一章详细阐述。自适应城市交通控制自适应信号控制系统必须有能力根据目前的交通状况,通过调整优化交通流量。所有使用的交通信号控制方法是基于反馈算法,而反馈算法的数据来源于过去的几分钟到几年时间里的交通需求。当前的交通控
64、制系统运作网络是根据测到的交通状况来协调适应性和灵活性。这些还不是根据交通需求在短时间内改变的最佳方法。其基本前提是,现有的信号计划工具能够根据不同的情况作出合适的信号计划。但是,目前可用的工具都是积极主动型,或者元规则型。这就使得改变控制器的方式放在系统中进行。交通控制的下一个逻辑步骤是将元规则、主动的和目标导向行为都列入。为此,从人工智能和人工智能代理的贡献可以预测,加强管制的主要方面包括:与冲突的目标处理能力;基于时间分析的决策能力;管理、学习、自我调整和应对非经常性和突发事件的能力(Ambrosino等.,1994)。什么是智能代理 代理技术是人工智能的一个新概念。人工智能代理模式是基
65、于无功,自主,内部机动的实体,动态的居住概念,并不一定是完全可预见的环境(Weiss,1999年)。自主是指在一段长时间内,能够作为一个独立单元,执行必要的行动以实现多种预先指定的目标,同时对同一体载传感器产生的刺激产生反应(Ziegler, 1990)。综合代理系统的特点是可以通过很多的代理系统互相合作去解决各种各样的问题。此外,人工智能、智能代理应该有一些额外的属性使得它自己可以解决一些实时问题、理解信息、有目标和方向、对情况进行分清区别、揉合新概念或想法。其解决问题的智能代理组件可以是一个以规则为基础的系统,但也可以是一个神经网络或模糊专家系统。显然,对代理技术而言,找到一个可行的解决方
66、案是必要的。通常在分散系统中最优的是局部而不是全部。这个问题不容易解决。解决的方案可以参照裁缝业的相互作用机制,或者有监督代理人协调其他代理人进行优化的过程。智能代理在UTC的使用是一种有益的范例代理技术适合不同领域的UTC。那些值得最重要一提的是:信息代理、代理交通仿真和交通控制。目前,大多数运用智能代理来进行信息代理。它们通过网络收集信息。对于采用特殊设计的代理商用户可以提供具体的资料。城市交通智能代理可以为用户提供有关的天气信息,交通拥堵,公共交通,道路封锁,最佳路线等,成为个人的旅行助理。代理技术也可以用于数据的分配。代理和多代理系统,有模拟仿真复杂交通系统的能力。这些系统通常为每一个交通参与者使用一个代理(类似于面向
- 温馨提示:
1: 本站所有资源如无特殊说明,都需要本地电脑安装OFFICE2007和PDF阅读器。图纸软件为CAD,CAXA,PROE,UG,SolidWorks等.压缩文件请下载最新的WinRAR软件解压。
2: 本站的文档不包含任何第三方提供的附件图纸等,如果需要附件,请联系上传者。文件的所有权益归上传用户所有。
3.本站RAR压缩包中若带图纸,网页内容里面会有图纸预览,若没有图纸预览就没有图纸。
4. 未经权益所有人同意不得将文件中的内容挪作商业或盈利用途。
5. 装配图网仅提供信息存储空间,仅对用户上传内容的表现方式做保护处理,对用户上传分享的文档内容本身不做任何修改或编辑,并不能对任何下载内容负责。
6. 下载文件中如有侵权或不适当内容,请与我们联系,我们立即纠正。
7. 本站不保证下载资源的准确性、安全性和完整性, 同时也不承担用户因使用这些下载资源对自己和他人造成任何形式的伤害或损失。