外文文献翻译译稿和原文
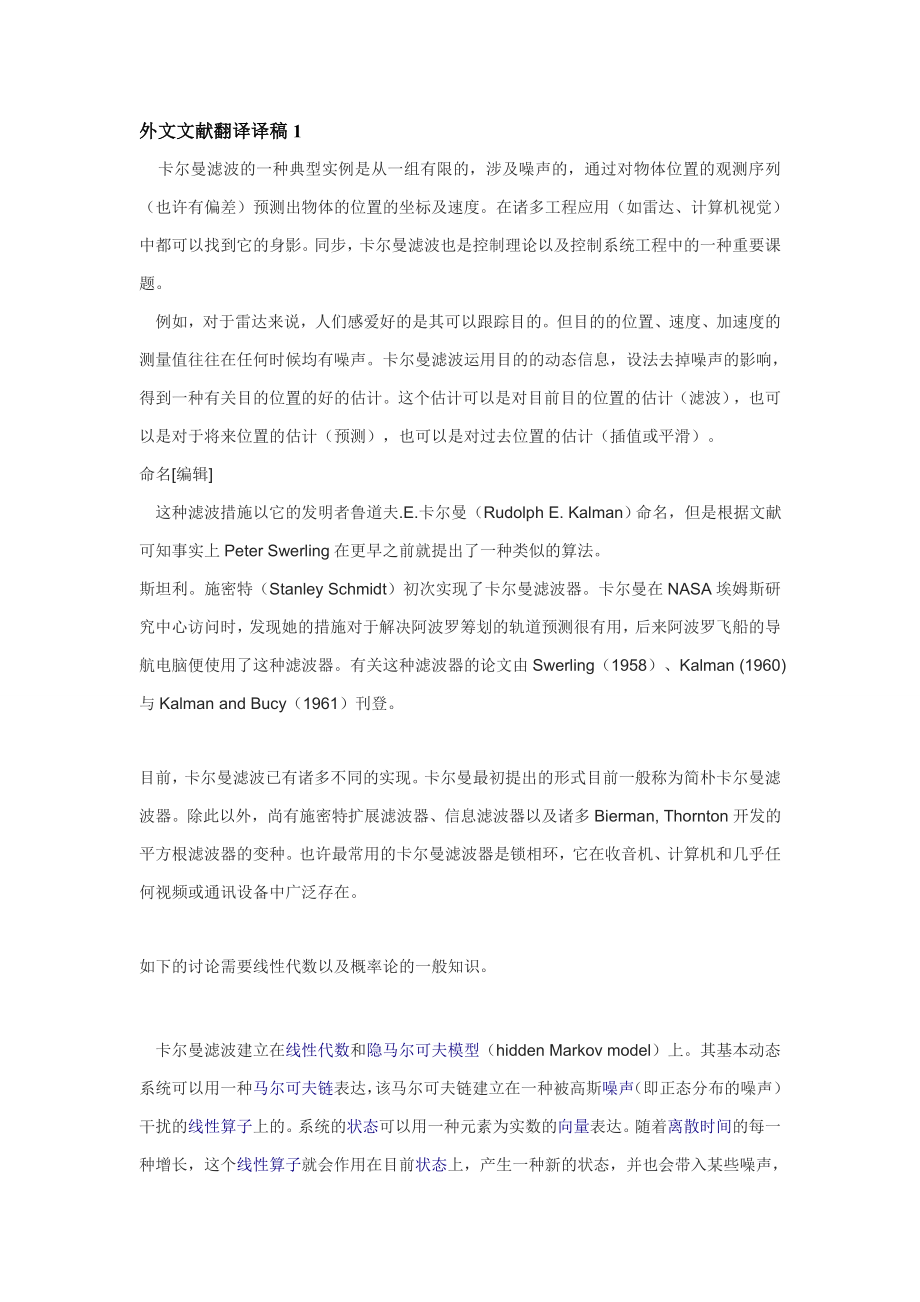


《外文文献翻译译稿和原文》由会员分享,可在线阅读,更多相关《外文文献翻译译稿和原文(19页珍藏版)》请在装配图网上搜索。
1、外文文献翻译译稿1 卡尔曼滤波的一种典型实例是从一组有限的,涉及噪声的,通过对物体位置的观测序列(也许有偏差)预测出物体的位置的坐标及速度。在诸多工程应用(如雷达、计算机视觉)中都可以找到它的身影。同步,卡尔曼滤波也是控制理论以及控制系统工程中的一种重要课题。例如,对于雷达来说,人们感爱好的是其可以跟踪目的。但目的的位置、速度、加速度的测量值往往在任何时候均有噪声。卡尔曼滤波运用目的的动态信息,设法去掉噪声的影响,得到一种有关目的位置的好的估计。这个估计可以是对目前目的位置的估计(滤波),也可以是对于将来位置的估计(预测),也可以是对过去位置的估计(插值或平滑)。命名编辑这种滤波措施以它的发明
2、者鲁道夫.E.卡尔曼(Rudolph E. Kalman)命名,但是根据文献可知事实上Peter Swerling在更早之前就提出了一种类似的算法。斯坦利。施密特(Stanley Schmidt)初次实现了卡尔曼滤波器。卡尔曼在NASA埃姆斯研究中心访问时,发现她的措施对于解决阿波罗筹划的轨道预测很有用,后来阿波罗飞船的导航电脑便使用了这种滤波器。有关这种滤波器的论文由Swerling(1958)、Kalman (1960)与Kalman and Bucy(1961)刊登。目前,卡尔曼滤波已有诸多不同的实现。卡尔曼最初提出的形式目前一般称为简朴卡尔曼滤波器。除此以外,尚有施密特扩展滤波器、信息
3、滤波器以及诸多Bierman, Thornton开发的平方根滤波器的变种。也许最常用的卡尔曼滤波器是锁相环,它在收音机、计算机和几乎任何视频或通讯设备中广泛存在。如下的讨论需要线性代数以及概率论的一般知识。 卡尔曼滤波建立在线性代数和隐马尔可夫模型(hidden Markov model)上。其基本动态系统可以用一种马尔可夫链表达,该马尔可夫链建立在一种被高斯噪声(即正态分布的噪声)干扰的线性算子上的。系统的状态可以用一种元素为实数的向量表达。随着离散时间的每一种增长,这个线性算子就会作用在目前状态上,产生一种新的状态,并也会带入某些噪声,同步系统的某些已知的控制器的控制信息也会被加入。同步,
4、另一种受噪声干扰的线性算子产生出这些隐含状态的可见输出。 卡尔曼滤波是一种递归的估计,即只要获知上一时刻状态的估计值以及目前状态的观测值就可以计算出目前状态的估计值,因此不需要记录观测或者估计的历史信息。卡尔曼滤波器与大多数滤波器不同之处,在于它是一种纯正的时域滤波器,它不需要像低通滤波器等频域滤波器那样,需要在频域设计再转换届时域实现。卡尔曼滤波器的状态由如下两个变量表达:,在时刻k的状态的估计;,误差有关矩阵,度量估计值的精确限度。卡尔曼滤波器的操作涉及两个阶段:预测与更新。在预测阶段,滤波器使用上一状态的估计,做出对目前状态的估计。在更新阶段,滤波器运用对目前状态的观测值优化在预测阶段获
5、得的预测值,以获得一种更精确的新估计值。预测(预测状态)(预测估计协方差矩阵)更新一方面要算出如下三个量:(测量余量,measurement residual)(测量余量协方差)(最优卡尔曼增益)然后用它们来更新滤波器变量x与P:(更新的状态估计)(更新的协方差估计)使用上述公式计算仅在最优卡尔曼增益的时候有效。使用其她增益的话,公式要复杂某些不变量(Invariant)如果模型精确,并且与的值精确的反映了最初状态的分布,那么如下不变量就保持不变:所有估计的误差均值为零且协方差矩阵精确的反映了估计的协方差:请注意,其中表达的盼望值,。 实例考虑在无摩擦的、无限长的直轨道上的一辆车。该车最初停在
6、位置0处,但时不时受到随机的冲击。我们每隔t秒即测量车的位置,但是这个测量是非精确的;我们想建立一种有关其位置以及速度的模型。我们来看如何推导出这个模型以及如何从这个模型得到卡尔曼滤波器。由于车上无动力,因此我们可以忽视掉Bk和uk。由于F、H、R和Q是常数,因此时间下标可以去掉。车的位置以及速度(或者更加一般的,一种粒子的运动状态)可以被线性状态空间描述如下:其中是速度,也就是位置对于时间的导数。我们假设在(k1)时刻与k时刻之间,车受到ak的加速度,其符合均值为0,原则差为a的正态分布。根据牛顿运动定律,我们可以推出其中且我们可以发现(由于a是一种标量)。在每一时刻,我们对其位置进行测量,
7、测量受到噪声干扰。我们假设噪声服从正态分布,均值为0,原则差为z。其中且如果我们懂得足够精确的车最初的位置,那么我们可以初始化并且,我们告诉滤波器我们懂得确切的初始位置,我们给出一种协方差矩阵:如果我们不确切的懂得最初的位置与速度,那么协方差矩阵可以初始化为一种对角线元素是B的矩阵,B取一种合适的比较大的数。此时,与使用模型中已有信息相比,滤波器更倾向于使用初次测量值的信息。推推导后验协方差矩阵按照上边的定义,我们从误差协方差开始推导如下:代入再代入与整顿误差向量,得由于测量误差vk与其她项是非有关的,因此有运用协方差矩阵的性质,此式可以写作使用不变量Pk|k-1以及Rk的定义这一项可以写作
8、:这一公式对于任何卡尔曼增益Kk都成立。如果Kk是最优卡尔曼增益,则可以进一步简化,请见下文。最优卡尔曼增益的推导卡尔曼滤波器是一种最小均方误差估计器,后验状态误差估计(英文:a posterioristate estimate)是我们最小化这个矢量幅度平方的盼望值,这等同于最小化后验估计协方差矩阵Pk|k的迹(trace)。将上面方程中的项展开、抵消,得到:当矩阵导数是0的时候得到Pk|k的迹(trace)的最小值:此处须用到一种常用的式子,如下: 从这个方程解出卡尔曼增益Kk:这个增益称为最优卡尔曼增益,在使用时得到最小均方误差。后验误差协方差公式的化简在卡尔曼增益等于上面导出的最优值时,
9、计算后验协方差的公式可以进行简化。在卡尔曼增益公式两侧的右边都乘以SkKkT得到根据上面后验误差协方差展开公式,最后两项可以抵消,得到.这个公式的计算比较简朴,因此实际中总是使用这个公式,但是需注意这公式仅在使用最优卡尔曼增益的时候它才成立。如果算术精度总是很低而导致数值稳定性浮现问题,或者特意使用非最优卡尔曼增益,那么就不能使用这个简化;必须使用上面导出的后验误差协方差公式。自适应滤波器是可以根据输入信号自动调节性能进行数字信号解决的数字滤波器。作为对比,非自适应滤波器有静态的滤波器系数,这些静态系数一起构成传递函数。对于某些应用来说,由于事先并不懂得所需要进行操作的参数,例如某些噪声信号的
10、特性,因此规定使用自适应的系数进行解决。在这种状况下,一般使用自适应滤波器,自适应滤波器使用反馈来调节滤波器系数以及频率响应。总的来说,自适应的过程波及到将代价函数用于拟定如何更改滤波器系数从而减小下一次迭代过程成本的算法。价值函数是滤波器最佳性能的判断准则,例如减小输入信号中的噪声成分的能力。随着数字信号解决器性能的增强,自适应滤波器的应用越来越常用,时至今日它们已经广泛地用于手机以及其他通信设备、数码录像机和数码照相机以及医疗监测设备中假设医院正在监测一种患者的心脏跳动,即心电图,这个信号受到 50Hz(许多国家供电所用频率)噪声的干扰剔除这个噪声的措施之一就是使用 50Hz 的陷波滤波器
11、(en:notch filter)对信号进行滤波。但是,由于医院的电力供应会有少量波动,因此我们假设真正的电力供应也许会在 47Hz 到 53Hz 之间波动。为了剔除 47 到 53Hz 之间的频率的静态滤波器将会大幅度地减少心电图的质量,这是由于在这个阻带之内很有也许就有心脏跳动的频率分量。为了避免这种也许的信息丢失,可以使用自适应滤波器。自适应滤波器将患者的信号与电力供应信号直接作为输入信号,动态地跟踪噪声波动的频率。这样的自适应滤波器一般阻带宽度更小,这就意味着这种状况下用于医疗诊断的输出信号就更加精确。扩展卡尔曼滤波器在扩展卡尔曼滤波器(Extended Kalman Filter,简
12、称EKF)中状态转换和观测模型不需要是状态的线性函数,可替代为(可微的)函数。函数f可以用来从过去的估计值中计算预测的状态,相似的,函数h可以用来以预测的状态计算预测的测量值。然而f和h不能直接的应用在协方差中,取而代之的是计算偏导矩阵(Jacobian)。在每一步中使用目前的估计状态计算Jacobian矩阵,这几种矩阵可以用在卡尔曼滤波器的方程中。这个过程,实质上将非线性的函数在目前估计值处线性化了。这样一来,卡尔曼滤波器的等式为:预测使用Jacobians矩阵更新模型更新预测犹如扩展卡尔曼滤波器(EKF)同样, UKF的预测过程可以独立于UKF的更新过程之外,与一种线性的(或者的确是扩展卡
13、尔曼滤波器的)更新过程合并来使用;或者,UKF的预测过程与更新过程在上述中地位互换亦可。外文文献翻译原文1Kalman filtering, also known aslinear quadratic estimation(LQE), is analgorithmthat uses a series of measurements observed over time, containingnoise(random variations) and other inaccuracies, and produces estimates of unknown variables that tend
14、to be more precise than those based on a single measurement alone. More formally, the Kalman filter operatesrecursivelyon streams of noisy input data to produce a statistically optimalestimateof the underlyingsystem state. The filter is named afterRudolf (Rudy) E. Klmn, one of the primary developers
15、 of its theory.The Kalman filter has numerousapplicationsin technology. A common application is forguidance, navigation and controlof vehicles, particularly aircraft and spacecraft. Furthermore, the Kalman filter is a widely applied concept intime seriesanalysis used in fields such assignal processi
16、ngandeconometrics. Kalman filters also are one of the main topics in the field of Robotic motion planning and control, and sometimes included inTrajectory optimization.The algorithm works in a two-step process. In the prediction step, the Kalman filter produces estimates of the current state variabl
17、es, along with their uncertainties. Once the outcome of the next measurement (necessarily corrupted with some amount of error, including random noise) is observed, these estimates are updated using aweighted average, with more weight being given to estimates with higher certainty. Because of the alg
18、orithms recursive nature, it can run inreal timeusing only the present input measurements and the previously calculated state and its uncertainty matrix; no additional past information is required.It is a common misconception that the Kalman filter assumes that all error terms and measurements are G
19、aussian distributed. Kalmans original paper derived the filter using orthogonal projection theory to show that the covariance is minimized, and this result does not require any assumption, e.g., that the errors are Gaussian.1He then showed that the filter yields the exact conditional probability est
20、imate in the special case that all errors are Gaussian-distributed.Extensions and generalizations to the method have also been developed, such as theextended Kalman filterand theunscented Kalman filterwhich work on nonlinear systems. The underlying model is aBayesian modelsimilar to ahidden Markov m
21、odelbut where the state space of thelatent variablesis continuous and where all latent and observed variables have Gaussian distributions.The Kalman filters are based on linear dynamic systems discretized in the time domain. They are modelled on a Markov chain built on linear operators perturbed by
22、errors that may include Gaussian noise. The state of the system is represented as a vector of real numbers. At each discrete time increment, a linear operator is applied to the state to generate the new state, with some noise mixed in, and optionally some information from the controls on the system
23、if they are known. Then, another linear operator mixed with more noise generates the observed outputs from the true (hidden) state. The Kalman filter may be regarded as analogous to the hidden Markov model, with the key difference that the hidden state variables take values in a continuous space (as
24、 opposed to a discrete state space as in the hidden Markov model).The Kalman filter is arecursiveestimator. This means that only the estimated state from the previous time step and the current measurement are needed to compute the estimate for the current state. In contrast to batch estimation techn
25、iques, no history of observations and/or estimates is required. In what follows, the notationrepresents the estimate ofat timengiven observations up to, and including at timem n.The state of the filter is represented by two variables: , thea posterioristate estimate at timekgiven observations up to
26、and including at timek; , thea posteriorierror covariance matrix (a measure of the estimatedaccuracyof the state estimate).The Kalman filter can be written as a single equation, however it is most often conceptualized as two distinct phases: Predict and Update. The predict phase uses the state estim
27、ate from the previous timestep to produce an estimate of the state at the current timestep. This predicted state estimate is also known as thea prioristate estimate because, although it is an estimate of the state at the current timestep, it does not include observation information from the current
28、timestep. In the update phase, the currenta prioriprediction is combined with current observation information to refine the state estimate. This improved estimate is termed thea posterioristate estimate.Typically, the two phases alternate, with the prediction advancing the state until the next sched
29、uled observation, and the update incorporating the observation. However, this is not necessary; if an observation is unavailable for some reason, the update may be skipped and multiple prediction steps performed. Likewise, if multiple independent observations are available at the same time, multiple
30、 update steps may be performed (typically with different observation matricesHk).1415PredictPredicted (a priori) state estimatePredicted (a priori) estimate covarianceUpdateInnovation or measurement residualInnovation (or residual) covarianceOptimalKalman gainUpdated (a posteriori) state estimateUpd
31、ated (a posteriori) estimate covarianceThe formula for the updated estimate and covariance above is only valid for the optimal Kalman gain. Usage of other gain values require a more complex formula found in thederivationssection.InvariantsIf the model is accurate, and the values forandaccurately ref
32、lect the distribution of the initial state values, then the following invariants are preserved: (all estimates have a mean error of zero)whereis theexpected valueof, and covariance matrices accurately reflect the covariance of estimatesExample application, technicaleditConsider a truck on frictionle
33、ss, straight rails. Initially the truck is stationary at position 0, but it is buffeted this way and that by random uncontrolled forces. We measure the position of the truck every tseconds, but these measurements are imprecise; we want to maintain a model of where the truck is and what itsvelocityis
34、. We show here how we derive the model from which we create our Kalman filter.Sinceare constant, their time indices are dropped.The position and velocity of the truck are described by the linear state spacewhereis the velocity, that is, the derivative of position with respect to time.We assume that
35、between the (k1) andktimestep uncontrolled forces cause a constant acceleration ofakthat isnormally distributed, with mean 0 and standard deviationa. FromNewtons laws of motionwe conclude that(note that there is noterm since we have no known control inputs. Instead, we assume thatakis the effect of
36、an unknown input andapplies that effect to the state vector) whereandso thatwhereandAt each time step, a noisy measurement of the true position of the truck is made. Let us suppose the measurement noisevkis also normally distributed, with mean 0 and standard deviationz.whereandWe know the initial st
37、arting state of the truck with perfect precision, so we initializeand to tell the filter that we know the exact position and velocity, we give it a zero covariance matrix:If the initial position and velocity are not known perfectly the covariance matrix should be initialized with a suitably large nu
38、mber, sayL, on its diagonal.The filter will then prefer the information from the first measurements over the information already in the model.Deriving thea posterioriestimate covariance matrixStarting with our invariant on the error covariancePk|kas abovesubstitute in the definition ofand substitute
39、andand by collecting the error vectors we getSince the measurement errorvkis uncorrelated with the other terms, this becomesby the properties ofvector covariancethis becomeswhich, using our invariant onPk|k1and the definition ofRkbecomesThis formula (sometimes known as the Joseph form of the covaria
40、nce update equation) is valid for any value ofKk. It turns out that ifKkis the optimal Kalman gain, this can be simplified further as shown below.Kalman gain derivationThe Kalman filter is aminimum mean-square errorestimator. The error in thea posterioristate estimation isWe seek to minimize the exp
41、ected value of the square of the magnitude of this vector,. This is equivalent to minimizing thetraceof thea posterioriestimatecovariance matrix. By expanding out the terms in the equation above and collecting, we get:The trace is minimized when itsmatrix derivativewith respect to the gain matrix is
42、 zero. Using thegradient matrix rulesand the symmetry of the matrices involved we find thatSolving this forKkyields the Kalman gain:This gain, which is known as theoptimal Kalman gain, is the one that yieldsMMSEestimates when used.Simplification of thea posteriorierror covariance formulaThe formula
43、used to calculate thea posteriorierror covariance can be simplified when the Kalman gain equals the optimal value derived above. Multiplying both sides of our Kalman gain formula on the right bySkKkT, it follows thatReferring back to our expanded formula for thea posteriorierror covariance,we find t
44、he last two terms cancel out, givingThis formula is computationally cheaper and thus nearly always used in practice, but is only correct for the optimal gain. If arithmetic precision is unusually low causing problems withnumerical stability, or if a non-optimal Kalman gain is deliberately used, this
45、 simplification cannot be applied; thea posteriorierror covariance formula as derived above must be used.Anadaptive filteris a system with a linearfilterthat has atransfer functioncontrolled by variable parameters and a means to adjust those parameters according to anoptimization algorithm. Because
46、of the complexity of the optimization algorithms, most adaptive filters aredigital filters. Adaptive filters are required for some applications because some parameters of the desired processing operation (for instance, the locations of reflective surfaces in areverberantspace) are not known in advan
47、ce or are changing. The closed loop adaptive filter uses feedback in the form of an error signal to refine its transfer function. Generally speaking, the closed loop adaptive process involves the use of acost function, which is a criterion for optimum performance of the filter, to feed an algorithm,
48、 which determines how to modify filter transfer function to minimize the cost on the next iteration. The most common cost function is the mean square of the error signal.As the power ofdigital signal processorshas increased, adaptive filters have become much more common and are now routinely used in
49、 devices such as mobile phones and other communication devices, camcorders and digital cameras, and medical monitoring equipment.Assuming thehospitalis monitoringa patientsheartbeating,namely,ECG,thesignal is50 Hz(frequency is usedby many countriessupply)noiseNotch filtermethod to eliminatenoiseofth
50、isis the use of50Hz(en:notchfilter)of the signal filtering.However,because of the powersupply in hospital.There will be a littlefluctuation,sowe assume that thepowersupplyrealmay fluctuatein the 47Hzto 53Hz.In order toeliminate47 tostaticfilterswill greatlyreduce thefrequency of53HzbetweentheECGqual
51、ity,this isbecause in thestopbandwithinmight well havea frequency componentof beating heart.In order to avoid thepossibleloss of information,you can use theadaptive filter.The adaptive filter willsupplysignaland powerof patients directlyas the input signal,dynamicallytracking noisefluctuationfrequen
52、cy.Adaptive filterthis usuallystopbandwidth is smaller,which meansin this casean output signal formedical diagnosisis more accurate.Hybrid Kalman filtereditMost physical systems are represented as continuous-time models while discrete-time measurements are frequently taken for state estimation via a
53、 digital processor. Therefore, the system model and measurement model are given bywhere.InitializePredictThe prediction equations are derived from those of continuous-time Kalman filter without update from measurements, i.e.,. The predicted state and covariance are calculated respectively by solving a set of differential equations with the initial value equal to the estimate at the previous step.UpdateThe update equations are identical to those of the discrete-time Kalman filter.外文文献翻译译稿2外文文献翻译原文2
- 温馨提示:
1: 本站所有资源如无特殊说明,都需要本地电脑安装OFFICE2007和PDF阅读器。图纸软件为CAD,CAXA,PROE,UG,SolidWorks等.压缩文件请下载最新的WinRAR软件解压。
2: 本站的文档不包含任何第三方提供的附件图纸等,如果需要附件,请联系上传者。文件的所有权益归上传用户所有。
3.本站RAR压缩包中若带图纸,网页内容里面会有图纸预览,若没有图纸预览就没有图纸。
4. 未经权益所有人同意不得将文件中的内容挪作商业或盈利用途。
5. 装配图网仅提供信息存储空间,仅对用户上传内容的表现方式做保护处理,对用户上传分享的文档内容本身不做任何修改或编辑,并不能对任何下载内容负责。
6. 下载文件中如有侵权或不适当内容,请与我们联系,我们立即纠正。
7. 本站不保证下载资源的准确性、安全性和完整性, 同时也不承担用户因使用这些下载资源对自己和他人造成任何形式的伤害或损失。